Sampling a dataset
The dplyr
package has a function to gather a sample from your dataset, sample()
. You pass in the dataset to operate against and how many samples you want drawn, sample_n()
, and the fraction percentage, sample_frac()
, as in this example:
data <- sample_n(players, 30)glimpse(data)
We see the results as shown in the following screenshot:
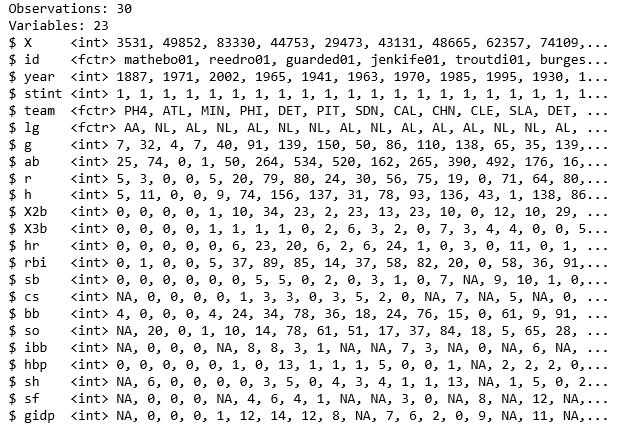
Note that there are 30 observations in the results set, as requested.
Filtering rows in a data frame
Another function we can use is the filter
function. The filter
function takes a data frame as an argument and a filtering statement. The function passes over each row of the data frame and returns those rows that meet the filtering statement:
#filter only players with over 200 hits in a season over200 <- filter(players, h > 200) head(over200) nrow(over200)
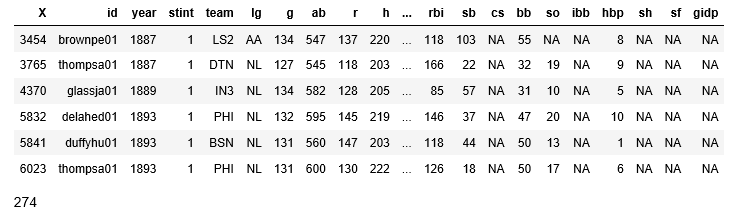
it looks like many players were capable of 200 hits a season. How about if we look at those players that could also get over 40 home runs in a season?
over200and40hr <- filter(players...