In this chapter, we discussed what recommender systems are and the types of these that exist today. We studied two main approaches to building recommender systems: content-based recommendations and collaborative filtering. We identified two types of collaborative filtering: user-based and item-based. We looked at the implementation of these approaches, and their pros and cons. We found out that an important issue in the implementation of recommender systems is the amount of data and the associated large computational complexity of algorithms. We considered approaches to overcome computational complexity problems, such as partial data updates and approximate iterative algorithms, such as ALS. We found out how matrix factorization can help to solve the problem with incomplete data, improve the generalizability of the model, and speed up the calculations. Also, we implemented...




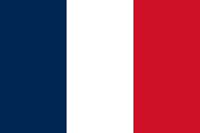


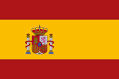



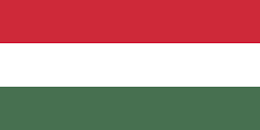

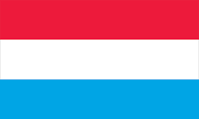





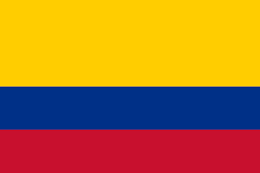
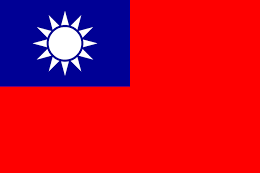
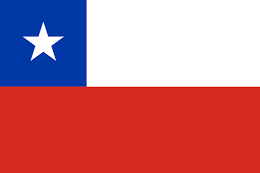



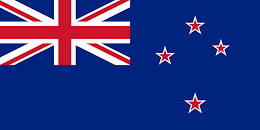
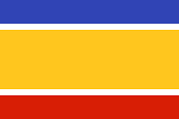
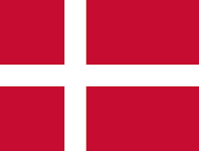
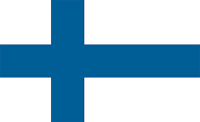







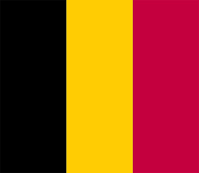

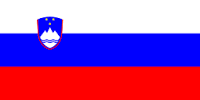





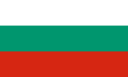


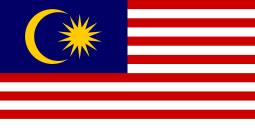



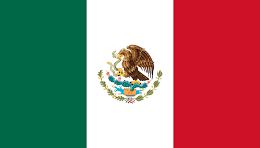
