Let's see how the similarity function is implemented through Siamese networks. The idea was first implemented at paper published by Taigman in 2014, DeepFace: Closing the Gap to Human-Level Performance in Face Verification. Then we will see how Siamese networks learn by giving a slightly more formal definition.
First, we will continue to use convolution architectures with many convolution layers:
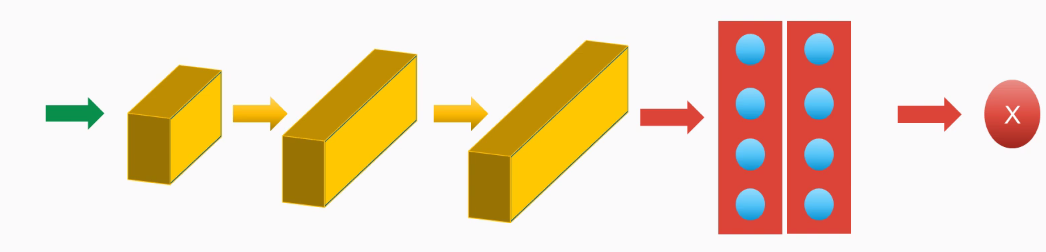
The fully connected layers within neurons, and the softmax for the prediction.
Let's fit the first image we want to compare, X1:
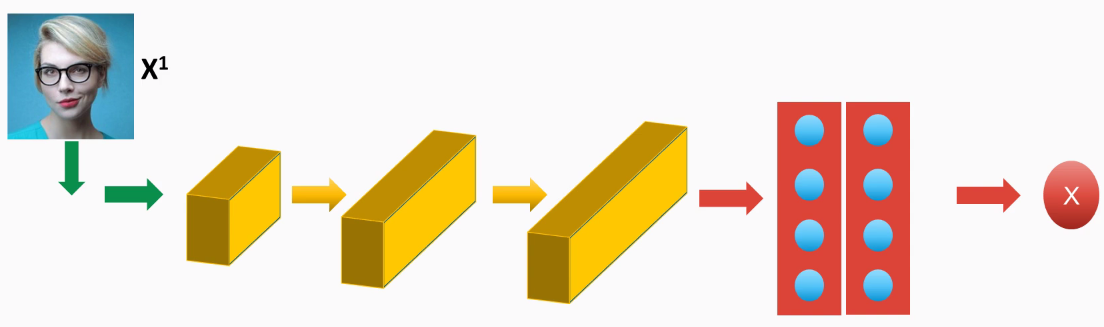
And what we will do is, through a forward pass, grab the activation values of the last fully connected layer, and we will refer to those values as F(x1), or sometimes also the encoded values of the image, because we transform this image through the forward paths to another set of values of the activation...