More bells and whistles for our neural network
Let's take a minute to look at some of the other elements of our neural network.
Momentum
In previous chapters we've explained gradient descent in terms of someone trying to find the way down a mountain by just following the slope of the floor. Momentum can be explained with an analogy to physics, where a ball is rolling down the same hill. A small bump in the hill would not make the ball roll in a completely different direction. The ball already has some momentum, meaning that its movement gets influenced by its previous movement.
Instead of directly updating the model parameters with their gradient, we update them with the exponentially weighted moving average. We update our parameter with an outlier gradient, then we take the moving average, which will smoothen out outliers and capture the general direction of the gradient, as we can see in the following diagram:
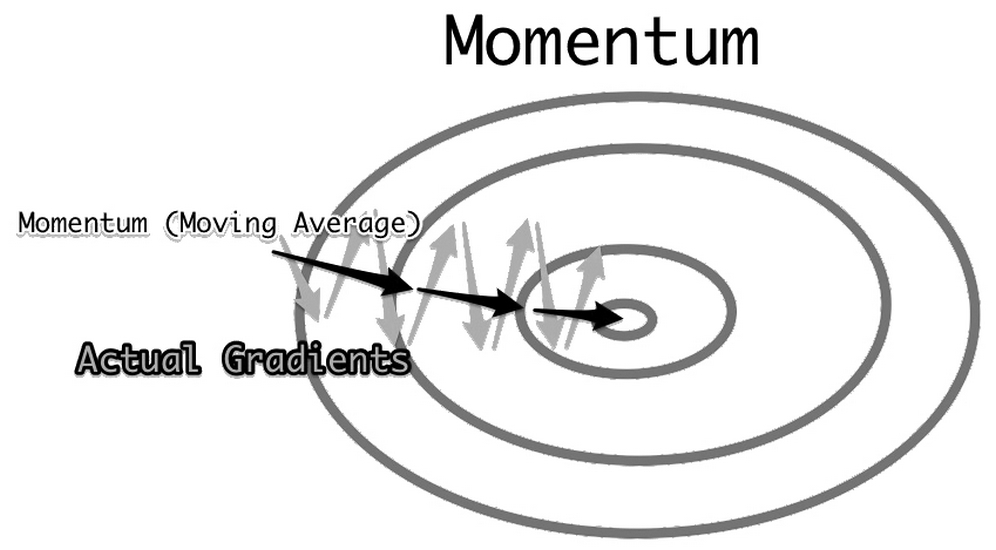
How momentum smoothens gradient updates
The exponentially weighted moving average...