In this chapter, we're going to introduce another approach to classification using a family of algorithms called Support Vector Machines (SVMs). They can work in both linear and non-linear scenarios, allowing high performance in many different contexts. Together with neural networks, SVMs probably represent the best choice for many tasks where it's not easy to find a good separating hyperplane. For example, for a long time, SVMs were the best choice for MNIST dataset classification, thanks to the fact that they can capture very high non-linear dynamics using a mathematical trick, without complex modifications to the algorithm. In the first part of this chapter, we're going to discuss the basics of linear SVM, which will then be used for their non-linear extensions. We'll also discuss some techniques to control the number of parameters...




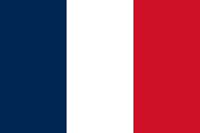


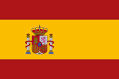




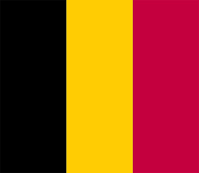
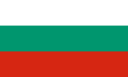
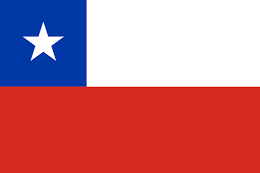
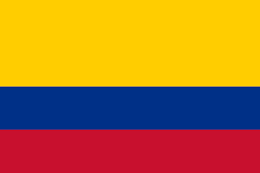
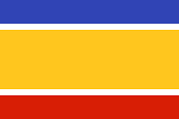

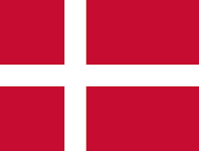



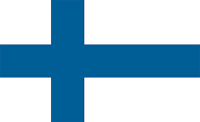

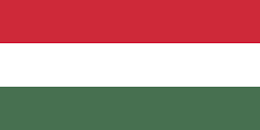






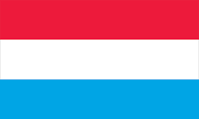
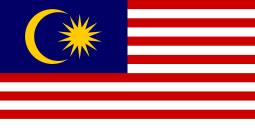

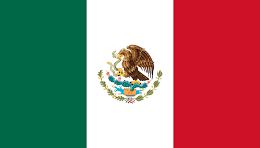

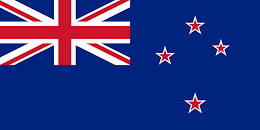







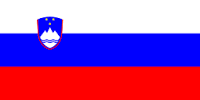




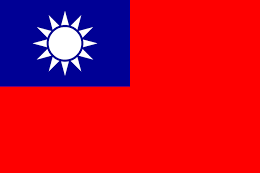


