Outlier removal
Outliers can stem from two possibilities. They either come from mistakes or they have a story behind them. In principle, outliers should be very rare, otherwise the experiment/survey for generating the dataset is intrinsically flawed.
The definition of an outlier is tricky. Outliers can be legitimate because they fall into the long tail end of the population. For example, a team working on financial crisis prediction establishes that a financial crisis occurs in one out of 1,000 simulations. Of course, the result is not an outlier that should be discarded.
It is often good to keep original mysterious outliers from the raw data if possible. In other words, the reason to remove outliers should only come from outside the dataset – only when you already know the originals. For example, if the heart rate data is strangely fast and you know there is something wrong with the medical equipment, then you can remove the bad data. The fact that you know the sensor/equipment is wrong can't be deduced from the dataset itself.
Perhaps the best example for including outliers in data is the discovery of Neptune. In 1821, Alexis Bouvard discovered substantial deviations in Uranus' orbit based on observations. This led him to hypothesize that another planet may be affecting Uranus' orbit, which was found to be Neptune.
Otherwise, discarding mysterious outliers is risky for downstream tasks. For example, some regression tasks are sensitive to extreme values. It takes further experiments to decide whether the outliers exist for a reason. In such cases, don't remove or correct outliers in the data preprocessing steps.
The following graph generates a scatter plot for the trestbps and chol fields. The highlighted data points are possible outliers, but I probably will keep them for now:
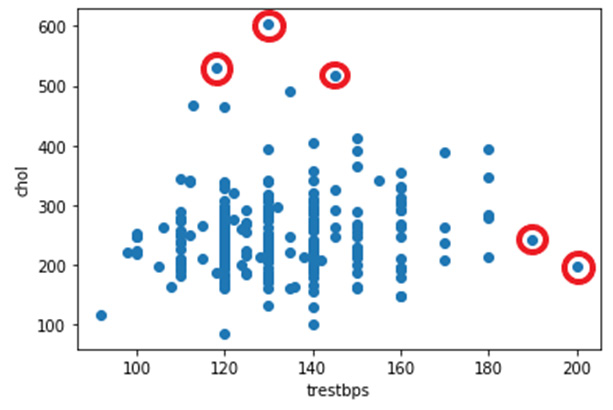
Figure 1.13 – A scatter plot of two fields in heart disease dataset
Like missing data imputation, outlier removal is tricky and depends on the quality of data and your understanding of the data.
It is hard to discuss systemized outlier removal without talking about concepts such as quartiles and box plots. In this section, we looked at the background information pertaining to outlier removal. We will talk about the implementation based on statistical criteria in the corresponding sections in Chapter 2, Essential Statistics for Data Assessment, and Chapter 3, Visualization with Statistical Graphs.