The monetary power of data
Modern-day organizations are immensely focused on revenue acceleration. Traditionally, organizations have primarily focused on increasing sales as a method of revenue acceleration… but is there a better method?
Modern-day organizations that are at the forefront of technology have made this possible using revenue diversification. Here are some of the methods used by organizations today, all made possible by the power of data.
Organic growth
During my initial years in data engineering, I was a part of several projects in which the focus of the project was beyond the usual. On several of these projects, the goal was to increase revenue through traditional methods such as increasing sales, streamlining inventory, targeted advertising, and so on. This meant collecting data from various sources, followed by employing the good old descriptive, diagnostic, predictive, or prescriptive analytics techniques.
But what can be done when the limits of sales and marketing have been exhausted? Where does the revenue growth come from?
Some forward-thinking organizations realized that increasing sales is not the only method for revenue diversification. They started to realize that the real wealth of data that has accumulated over several years is largely untapped. Instead of solely focusing their efforts entirely on the growth of sales, why not tap into the power of data and find innovative methods to grow organically?
This innovative thinking led to the revenue diversification method known as organic growth. Subsequently, organizations started to use the power of data to their advantage in several ways. Let's look at several of them.
Customer retention
Data scientists can create prediction models using existing data to predict if certain customers are in danger of terminating their services due to complaints. Based on this list, customer service can run targeted campaigns to retain these customers. By retaining a loyal customer, not only do you make the customer happy, but you also protect your bottom line.
Fraud prevention
Banks and other institutions are now using data analytics to tackle financial fraud. Based on key financial metrics, they have built prediction models that can detect and prevent fraudulent transactions before they happen. These models are integrated within case management systems used for issuing credit cards, mortgages, or loan applications.
Using the same technology, credit card clearing houses continuously monitor live financial traffic and are able to flag and prevent fraudulent transactions before they happen. Detecting and preventing fraud goes a long way in preventing long-term losses.
Problem detection
I was part of an internet of things (IoT) project where a company with several manufacturing plants in North America was collecting metrics from electronic sensors fitted on thousands of machinery parts. The sensor metrics from all manufacturing plants were streamed to a common location for further analysis, as illustrated in the following diagram:
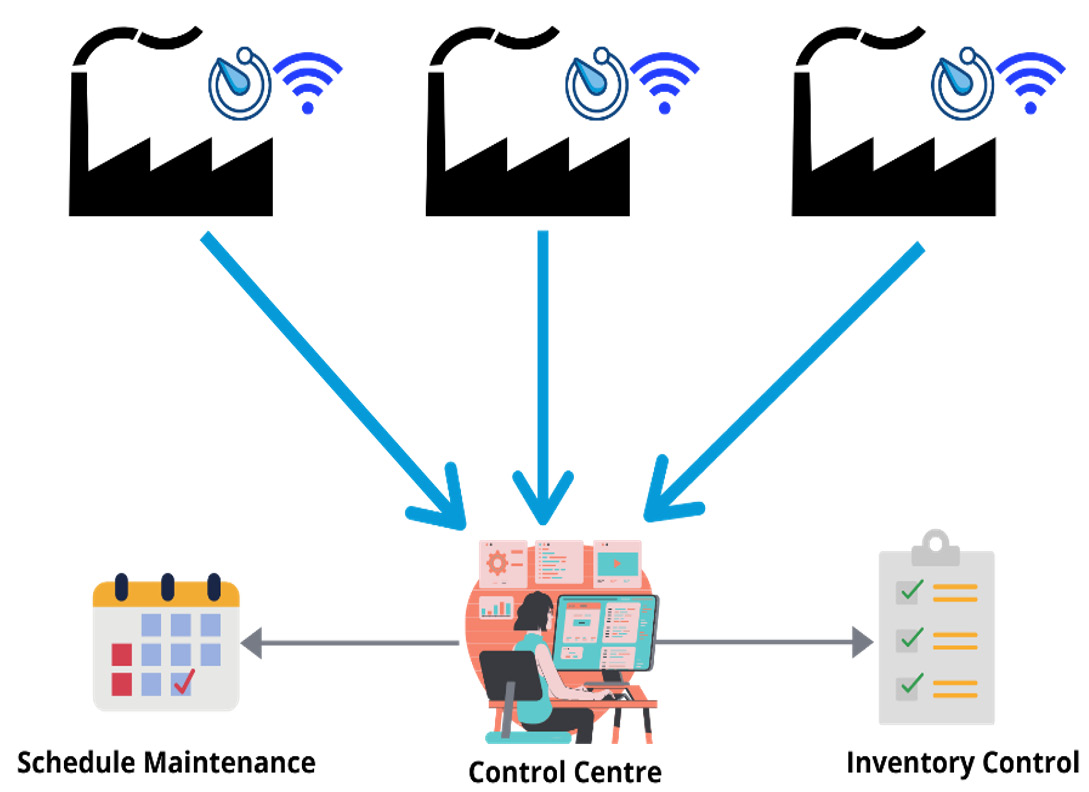
Figure 1.7 – IoT is contributing to a major growth of data
These metrics are helpful in pinpointing whether a certain consumable component such as rubber belts have reached or are nearing their end-of-life (EOL) cycle. Collecting these metrics is helpful to a company in several ways, including the following:
- The data indicates the machinery where the component has reached its EOL and needs to be replaced. Having this data on hand enables a company to schedule preventative maintenance on a machine before a component breaks (causing downtime and delays).
- The data from machinery where the component is nearing its EOL is important for inventory control of standby components. Before this system is in place, a company must procure inventory based on guesstimates. Buy too few and you may experience delays; buy too many, you waste money. At any given time, a data pipeline is helpful in predicting the inventory of standby components with greater accuracy.
The combined power of IoT and data analytics is reshaping how companies can make timely and intelligent decisions that prevent downtime, reduce delays, and streamline costs.
Data monetization
Innovative minds never stop or give up. They continuously look for innovative methods to deal with their challenges, such as revenue diversification. Organizations quickly realized that if the correct use of their data was so useful to themselves, then the same data could be useful to others as well.
As per Wikipedia, data monetization is the "act of generating measurable economic benefits from available data sources".
The following diagram depicts data monetization using application programming interfaces (APIs):
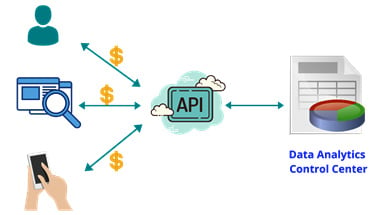
Figure 1.8 – Monetizing data using APIs is the latest trend
In the latest trend, organizations are using the power of data in a fashion that is not only beneficial to themselves but also profitable to others. In a recent project dealing with the health industry, a company created an innovative product to perform medical coding using optical character recognition (OCR) and natural language processing (NLP).
Before the project started, this company made sure that we understood the real reason behind the project—data collected would not only be used internally but would be distributed (for a fee) to others as well. Knowing the requirements beforehand helped us design an event-driven API frontend architecture for internal and external data distribution. At the backend, we created a complex data engineering pipeline using innovative technologies such as Spark, Kubernetes, Docker, and microservices. This is how the pipeline was designed:
- Several microservices were designed on a self-serve model triggered by requests coming in from internal users as well as from the outside (public).
- For external distribution, the system was exposed to users with valid paid subscriptions only. Once the subscription was in place, several frontend APIs were exposed that enabled them to use the services on a per-request model.
- Each microservice was able to interface with a backend analytics function that ended up performing descriptive and predictive analysis and supplying back the results.
The power of data cannot be underestimated, but the monetary power of data cannot be realized until an organization has built a solid foundation that can deliver the right data at the right time. Data engineering plays an extremely vital role in realizing this objective.