History of the Digital Twin
In this section, we will explore the Digital Twin concept's origins and intent by the original creators as we define the Digital Twin for this book and identify its key characteristics. The initial goal of a Digital Twin is to provide the same or better information than could be obtained by being in physical possession of the physical twin, in contrast to simulation and modeling to predict behavior based on "what-if scenarios."
Origin of the Digital Twin concept
The concept of a Digital Twin was first described by Dr Michael Grieves of the University of Michigan in a presentation to the Society of Manufacturing Engineering in October 2002. Grieves originally named it the Mirrored Spaces Model (MSM), but the name evolved, and he ascribed the term "Digital Twin" to John Vickers of NASA, who worked with Grieves on product life cycle management for complex systems.
Grieves and Vickers observed that technological advances in physical products and assets made systems more complex. New technologies also brought new capabilities, such as communication and computing, that could not be represented in the physical (mechanical and electronic) space. These capabilities increased the complexity of systems and required a mechanism to mitigate system complexity by providing improved information about the physical product or entity:
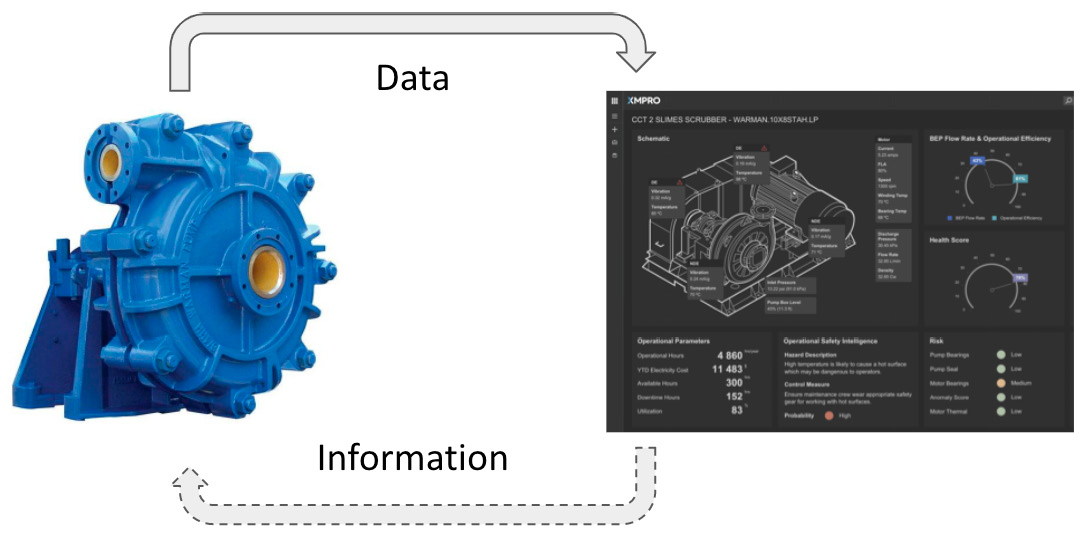
Figure 1.1 – Physical and virtual products combined to create a Digital Twin
MSM provides some insight into Grieves' approach to having a Digital Twin "mirror" a Physical Twin through the flow of data, from the physical product to the digital instance, and then how this information was exchanged and sent back to the physical one:
Three key concepts characterized these early Digital Twins. Digital Twin Prototype (DTP) is the "type" or model representation of the physical twin. It was also described as the "design version with all its variants." The DTP of a centrifugal pump, for example, is a single description and information model for the specific model of a pump. There is only one DTP or model, but multiple pumps may use that same model description. This brings us to the instance of a Digital Twin.
The Digital Twin Instance (DTI) is each instance of every physical entity, based on the DTP. We may have 150 pumps in the centrifugal pump example, and each pump may represent a unique instance, all of which is based on the common DTP for that specific model of the pump. It is possible to have only one instance based on a single model. A building is a typical example of such a configuration. There is only one building (DTI) and only one model of DTP. It is worth noting that any changes to the DTP will require the DTI to be updated to maintain the fidelity of the instance to the prototype.
Physical entities such as buildings and other complex products can often not be described by a single DTI. It is generally more of a collection or composition of different instances to make up the overall building definition. Grieves and Vickers addressed this challenge by introducing aggregation of instances. The following diagram illustrates the progression from a DTP model based on a physical entity, and how this is instantiated as a DTIs for individual physical entities that conform to the same Digital Twin Prototype:
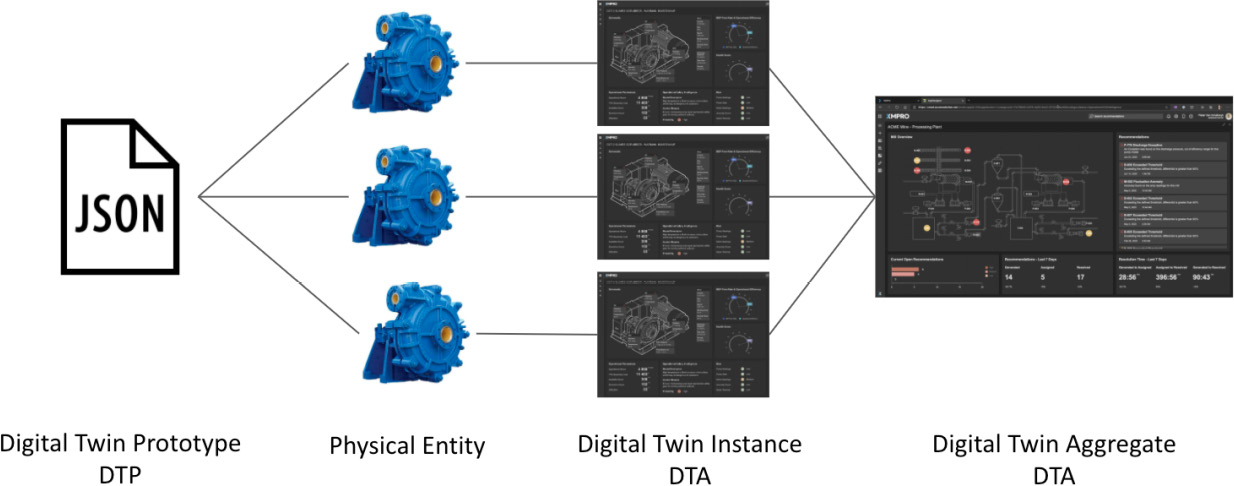
Figure 1.2 – Relationships between Digital Twin concepts
It also shows how multiple DTIs can be combined to create a Digital Twin Aggregate, which we will introduce next.
A Digital Twin Aggregate (DTA) is an aggregate collection of DTIs and other DTAs, and the mechanisms to query them. DTIs can exist independently of other instances, but DTAs cannot. The DPI of a single centrifugal pump can exist in isolation, whereas the aggregate of a grouping of pumps are dependent on a collection of instances. A DTA can provide additional insight into the behavior of the aggregate, which cannot be achieved at the instance level. For example, we could monitor the pressure difference across the slurry pumps for a specific processing area, which will give us a different insight into the overall operation of this part of the mining process plant versus the information that we can gather from the DTI of a single pump.
It is important to note that DTPs and their corresponding DTIs are different from Computer-Aided Drawing (CAD) files. A CAD file can describe the physical dimensions of a component, but it lacks the file structure to capture, store, and maintain the properties of all the components based on the CAD file. DTPs and DTIs are typically based on file structures such as JavaScript Object Notation (JSON) or Extensible Markup Language (XML) to manage the extended metadata of a Digital Twin.
Many descriptions and definitions emerged from vendors, analysts, and research organizations over the years based on this initial work by Grieves and Vickers, but they are outside the scope of this book.
In 2012, NASA described a Digital Twin as a multiphysics, multiscale, probabilistic, ultra-high fidelity simulation that reflects the state of a corresponding twin based on historical data, real-time sensor data, and a physical model.
What is a Digital Twin?
There are numerous vendor and analyst definitions of Digital Twins, each describing features that reflect the capabilities or interests of the authors. The Digital Twin Consortium's definition provides a vendor- and technology-agnostic definition that describes the what, when, why, and how of Digital Twins at a high level:
We propose a more focused definition of a Digital Twin for this book that relates to the process that we will describe to help you build your first Digital Twin. It contains the necessary elements and common understanding for your initial Digital Twin:
Our definition addresses the critical elements of a Digital Twin that we will require in the remaining chapters as we demonstrate how to create our first Digital Twin. It highlights the requirement for a prototype or template model of a physical entity. It recognizes that there may be multiple instances that represent multiple assets of the same type. It also highlights the requirement that the Digital Twin should address specific business challenges or use cases and that they could be at any stage of the entity's life cycle.
Important Note
We differentiate between the life cycle of the entity and the life cycle of the Digital Twin. This distinction will be described later in this chapter.
Entities are not limited to physical assets, and we prefer the description of an entity from the ISO organization:
International Organization for Standardization (ISO) 24760-1:2011
In addition to the conventional physical asset view of a Digital Twin, this description of an entity allows us to include Digital Twins of processes, supply chains, organizations, and governments, among others. It can also be used in extreme examples, such as a hurricane or bushfire, in an emergency response use case. We will cover more examples in the Industry use of Digital Twins section.
A vital element of the Grieves and Vickers approach was that Digital Twins synchronized with a physical entity. This means that a digital model that only provides a simulation with no input from a physical asset does not qualify as a Digital Twin. It can be used to start the process of creating a Digital Twin as it may represent the DTP or DTA, but it requires the instance and the data to be synchronized to qualify as a Digital Twin. Examples of these digital models include 2D and 3D CAD design drawings, Building Information Management (BIM) models, planning simulation models, and AR visualizations based on design parameters.
Entity life cycle and Digital Twin development life cycle
Physical entities, products, and assets have life cycles, from planning and design through manufacturing or construction, operations, maintenance, and, finally, retirement or disposal. The asset life cycle represents the phases of physical twin development and use. The twin's digital version is a software-based digitalization that includes models, data, connectivity, analytics, and actions. The twin's digital version requires a software engineering approach, whereas the physical twin is based on engineering management practices such as product life cycle management (PLM).
It is important to note that we distinguish between the asset life cycle/Physical Twin and the Digital Twin development life cycle, as shown in the following diagram. We will be referring to both life cycles throughout this book, so this differentiation is important to keep in mind:
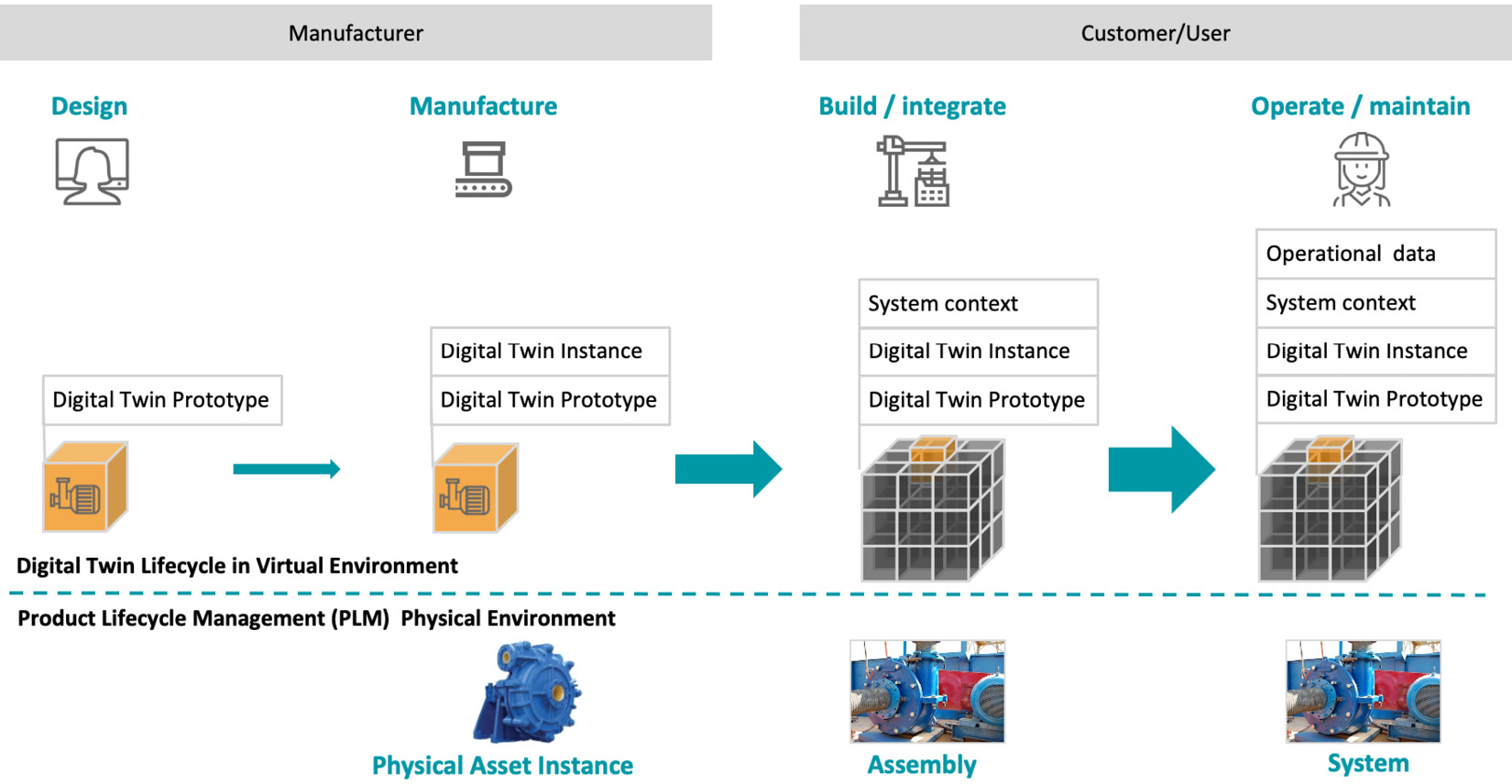
Figure 1.3 – Digital Twin and product life cycle relationship
Developing Digital Twins requires both traditional engineering as well as software development practices so that they work in harmony. This convergence of Operational Technology (OT) and Information Technology (IT) is a positive development for Digital Twins as it creates a shared understanding of both the physical and the digital requirements.
It is not the aim of this book to provide guidance on PLM for the physical twin. The digital development life cycle will be addressed in the remaining chapters as we build our first DTP. Also, it is not this book's aim to provide guidance on the digital development methodology that an organization should follow.
Our preference is an agile-based approach for our initial minimum viable Digital Twin in this book, but other software engineering approaches, such as the V-Model and Waterfall, can be used if you are more familiar with these methodologies. The V-Model approach is popular for designing and manufacturing complex systems in aviation and the military, but that is beyond the scope of this book.
Types of Digital Twins
The types of Digital Twins that we'll describe here are not a full taxonomy of a Digital Twin or a formal classification system. Still, this will highlight that different use cases have different types of Digital Twins.
Discrete versus composite
The scope and scale of a Digital Twin will vary based on the use case or problem that it is addressing. A key consideration when choosing your first Digital Twin is the level of complexity that your use case will require. More complex Digital Twins are typically composed by assembling different discrete or standalone Digital Twins:
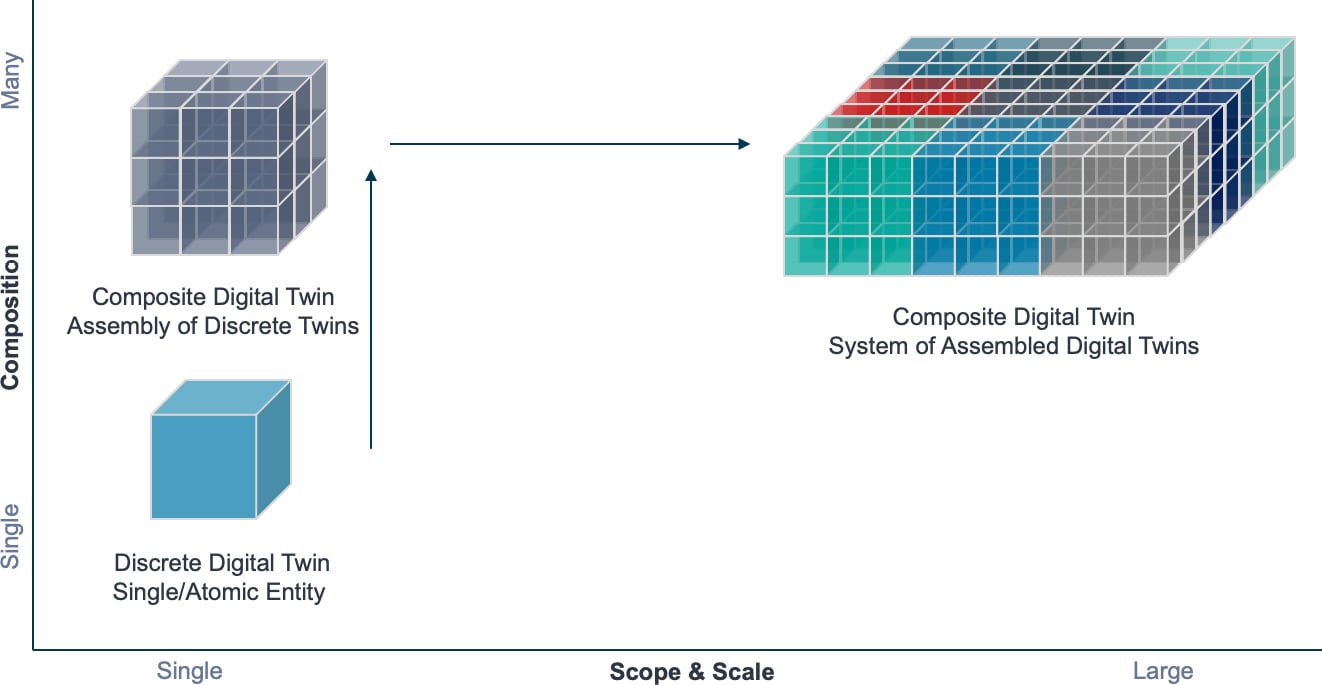
Figure 1.4 – Discrete and composite Digital Twin relationship
The preceding diagram shows the relationship between a discrete Digital Twin and a composite Digital Twin.
A discrete Digital Twin is the lowest level of abstraction that is sufficient to meet the requirements of a specific use case. It is often a single or atomic entity where no value would be added by breaking it down into components or parts, such as the gearbox or motor for a ball mill in mining. It doesn't need to be broken down into its component parts as its status and monitoring processes are reported at this entity level.
A composite Digital Twin is a combination of discrete Digital Twins that represent an entity comprising multiple individual components or parts. A composite Digital Twin can be an Assembly Twin, such as a ball mill in mining, or a System Twin that comprises multiple Assembly Twins, such as processing and refinement plants. A Composite Digital Twin is a system of systems with a more complex life cycle management challenge.
A discrete Digital Twin is typically a standalone component or asset that can function on its own to address a specific use case. An electrically driven centrifugal pump and its electric drive is a typical example of a discrete Digital Twin as monitoring and reporting is done at this level. An example of the discrete pump Digital Twin use case would be to predict pump failures based on a predictive analytics model.
A composite Digital Twin is an assembly of several discrete Digital Twins to create a new functional, composite asset. Combining several discrete pump Digital Twins with discrete autoclave Digital Twins creates a composite Digital Twin of a gold processing plant, which is used for production optimization.
A predictive maintenance use case example for a composite plant Digital Twin is more extensive than the predictive maintenance use case of the discrete pump Digital Twin, even though the pump is used in the plant use case.
The DTP that we will design and develop in this book will follow the Discrete Digital Twin pattern. The difference between the pump and the gold recovery plant leads to the second type of classification we need for Digital Twins.
Product versus facility
Industrial Digital Twins based on physical entities can broadly be applied to two prominent use cases. The first is a Digital Twin representing a manufactured product such as a pump, electric motor, hand drill, X-ray machine, automobile, or any other asset-based entity. The primary objective of the Digital Twin is to monitor the use of this specific entity, which may include indications of failures or sub-optimal operation.
The second type of industrial Digital Twin use case is around manufacturing or production facilities, which generally consist of a composition of individual assets. The Digital Twin is used to provide insight into the operations of the facility. The facility Digital Twin will consist of an assembly of unique product twins.
The discrete product Digital Twin can be used in two different ways in the composite facility Digital Twin; for example, where the product is part of the facility, such as a robotic arm on an assembly line.
The second use case is where the Digital Twin is part of the manufactured product, for example, an electric motor, a jet engine, or wind turbine, in the manufacturing facility.
A smart manufacturing use case, for example, uses a combination of product and facility Digital Twins. The product Digital Twin is used on the manufacturing line to determine the machine setup, tooling, and parts requirements.
Product Digital Twins are currently predominantly used in manufacturing scenarios, and manufacturers have no visibility of the use of the product once it has been shipped. As the adoption of Digital Twins increases, this scenario will likely change.
Product manufacturers are keen to extend access to the Digital Twin of the product beyond the point of the manufacture. Manufacturers provide Digital Twin solutions that allow them to collect operations and usage data that will be used for product improvements and new service-based products.
The Digital Twin can provide insights into how products are used, and this can be fed back into the product's design for improvements. These insights will enable manufacturers to develop and manufacture products that are more fit for purpose and at higher quality levels.
The real opportunity for many manufacturers is to provide not only the product but also the maintenance services around the product. Access to the operating product's Digital Twin will provide the necessary insight to predict when failures are likely to happen, or when the product is due for servicing or replacement. This opens up a new business model for many manufacturers with new revenue streams, which wasn't possible without the Digital Twin technology.
Simulation versus operational
During the early stages of the product or asset life cycle, the Digital Twin use cases focus more on simulation scenarios. In contrast, use cases in the later stages of the product tend to focus more on operational and maintenance challenges.
We can broadly classify Digital Twins into simulation and operations twins. This does not mean to say that simulation cannot be used in operations, but the predominant application is to manage operations. During the design phase, the principal use cases simulate different scenarios to determine the product or facility's ideal design:
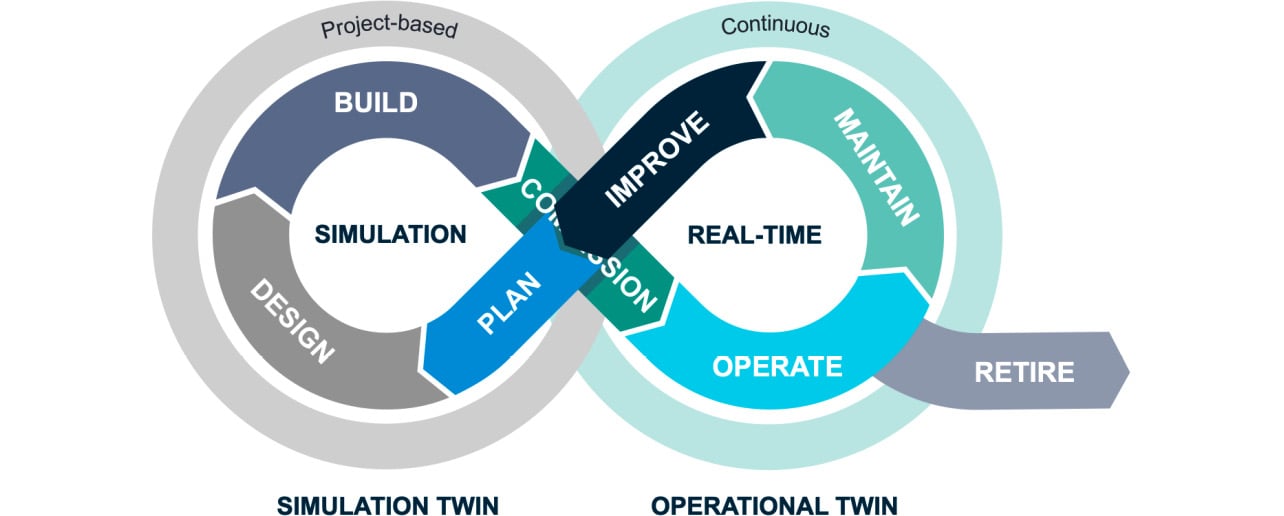
Figure 1.5 – Simulation and operational Digital Twin types
Simulations also tend to be more project-based, whereas operational use cases are continuous over the operations and maintenance life cycle of the entity. The infinity loop model best describes this, as shown in the preceding diagram. The plan, design, build, and commissioning phases are typically project-based simulations for Digital Twins with very slow synchronization or twinning rates.
The operate, maintain, and improve phases are continuous and often real-time applications of Digital Twins. Recommendations from the improve phase may require us to make modifications to the product that would lead back into the planning and project-based designing manufacturing cycle. It is important to note that this is not a qualifying criterion for classification but rather a typical pattern when using industrial Digital Twins.
Analytics versus physics-based
The digital models that represent the entity can be based on analytics and physics-based algorithms. These algorithms can use historical and real-time data to enable simulation and predictions of the current and future state or behavior:
- Analytics-based algorithms are statistical or mathematical techniques that are used to predict entity behavior based on historical data. These models are most often based on AI or machine learning techniques. Predicting the remaining useful life of equipment such as a centrifugal pump using a regression model is an example of an analytics-based application.
- Physics-based algorithms are based on the physical laws of using engineering equations of state and material properties to provide insight into the current or predicted state of a product. A Computational Fluid Dynamic (CFD) could use design parameters or real-time data to provide insights into a centrifugal pump's behavior under specific conditions. Finite element analysis is another example of a physics-based algorithm that's used to provide insights into a product's structural integrity under simulated or real-time conditions.
Simulation and operational twins can use both analytics and physics-based models for simulation or operational analysis and predictions.
Characteristics of a Digital Twin
The characteristics described in this book are focused on the key elements that will be required when you develop your first DTP. There may be additional characteristics in other more complex use cases, but these provide a great starting point for Digital Twin evaluation:
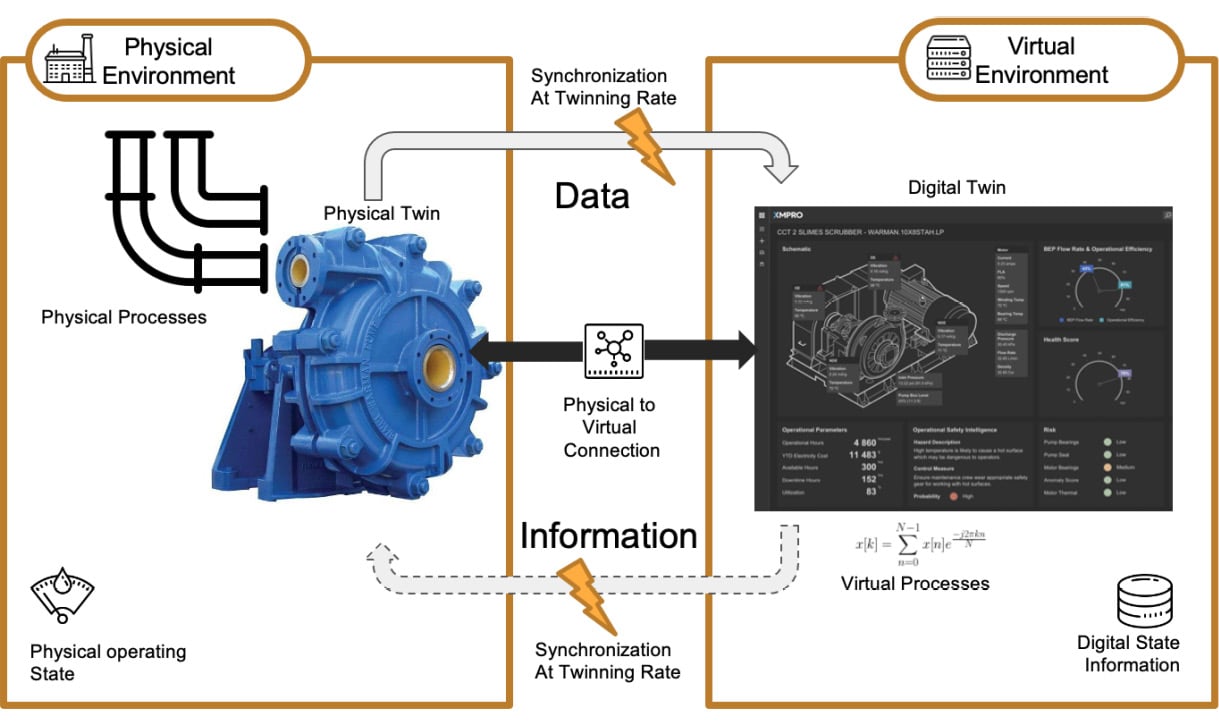
Figure 1.6 – Digital Twin characteristics
The preceding diagram provides a visual representation of the characteristics of a Digital Twin that are based on the Digital Twin definition:
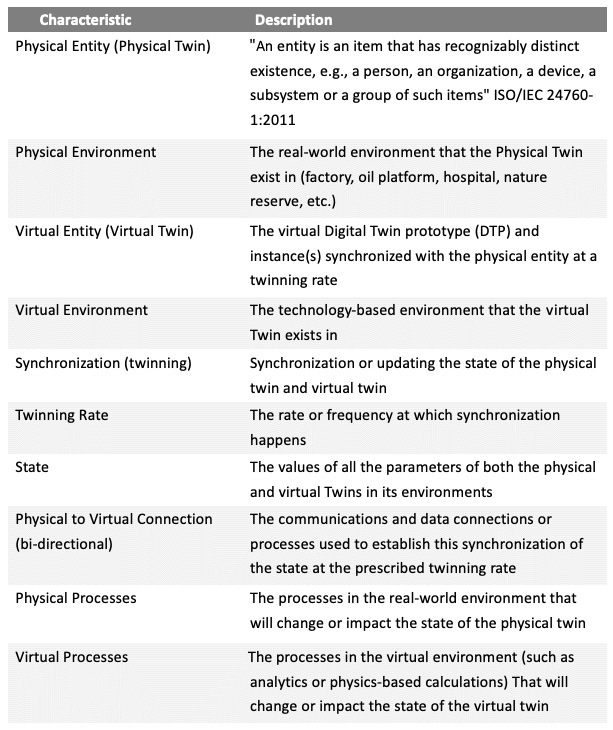
Figure 1.7 – Key characteristics
Digital Twin fidelity is not necessarily a characteristic and more of a result of the model's sophistication and twinning rate. We are seeing an increase in Digital Twin fidelity as the computing capabilities in the virtual environment continue to expand exponentially according to Moore's law.
Metrology is also an essential requirement for twinning as it involves accurately measuring the state parameters. It is not a characteristic, but it is essential to ensure accurate representations of the physical state.
As we start building the first DTP in this book, we will continue to refer to these characteristics. It is useful to document the specific aspects of your Digital Twin in this table format when you choose your ideal candidate. It will provide an initial test to help determine if a particular use case or scenario would qualify as a Digital Twin.
Models and data
Virtual twins exist in virtual environments in a digitized format and rely on different data sources and models to turn data into information. There is a wide variety of data sources and models that provide virtual twin data capabilities. For this book, we have simplified them into six main categories:
- Temporal or Time Series Data: This data provides time-stamped, real-time synchronization of physical state data through sensors, automation, and control and IoT systems. It is stored and accessed from time series databases, historians, and IoT platforms.
- Master Data: Master data is generally slow-changing contextual data that's stored in systems. It is used to describe assets or entities such as enterprise asset management systems (EAM) and enterprise resource management systems (ERP), as well as in Digital Twin services such as Azure Digital Twins.
- Transactional Data: Operational, transactional data such as production records, maintenance records, supply chain information, and other business records related to the Digital Twin are generally stored in ERP, Computerized Maintenance Management Systems (CMMS), Manufacturing Execution Systems (MES), Business Process Management (BPM), and production systems.
- Physics-Based Models: Physics-based and engineering calculations use real-time, transactional, and master data to describe or predict the physical entity's state. Examples include finite element methods (FEM) and computational fluid dynamics (CFD), as well as other laws of nature, such as the laws of thermodynamics.
- Analytical Models: These are mathematical and statistical models in the virtual environment that use the same data sources described previously to predict the current and future state of the physical twin and its environment. This includes AI and machine learning for predictive maintenance and operations use cases.
- Visual Models: These are digitalized visualization modeling capabilities such as Computer Aided Design (CAD), Augmented Reality (AR), Virtual Reality (VR), Building Information Modeling (BIM), Geographic Information System (GIS), and geophysics models. These models are often used to reduce system complexity and provide a visual analysis of the different data sources.
The heterogeneous nature of all these different data sources contributes to the fact that integration remains a significant challenge when creating Digital Twins. We will refer to this point when we start building the DTP in the technical chapters of this book. Integration and interoperability can consume a large part of the resources in a Digital Twin project. It is essential to understand the data requirements, as well as what physics and analytical models will be required to address the specific business problem that the Digital Twin set out to solve.
Digital Thread
Digital Thread is a term that gained popularity at the same time as Digital Twins. It is sometimes confused or associated with Digital Twins. The Digital Thread can exist without formal Digital Twins, but Digital Twins are built on Digital Thread information.
The Digital Thread evolved from PLM, which captured the process from design to manufacture for physical products. The Digital Thread creates a traceable, unique birth record with the actual data for each composite entity or product assembly and all its components. It captures all its interactions and transactions throughout its life cycle through to retirement. It extends the life cycle record beyond the PLM's focus into operations, maintenance, and disposal.
The Digital Twin's focus on the life cycle phase is to address specific use cases, whereas the Digital Thread is the data aggregator across all life cycle phases. The Digital Thread provides component traceability regarding its design iterations, manufacturing processes, testing, and quality measures. It often includes environmental metadata such as manufacturer information, storage temperature, and humidity for specific components. The Digital Thread may also include information on the relationship between components, including Bill of Material (BOM) structures and maintenance records.
Some Digital Twins may extend beyond a single phase or blend phases, such as operate and maintain, but it is unlikely to extend across the full life cycle. Digital Twin components such as models, analytics, and real-time sensor data may be reused, but there is generally not a single Digital Twin that spans all of the asset life cycle phases.
Digital Threads integrate data from multiple design, manufacturing, and operational data sources. They may not replicate the information from CAD, MES, EAM, and ERP systems, but they maintain references to the source data for the "birth record to death certificate" for products and components.
The Digital Twin of an aircraft fleet, for example, may be used to prioritize maintenance for individual aircraft, where the Digital Thread for the aircraft will assist in Failure Mode and Effect Analysis (FMEA) and Root Cause Analysis (RCA) in the event of a component failure. It can provide insight into the design, manufacture, and maintenance of the component. A Digital Twin can also assist in identifying aircraft that may have the same faulty component:
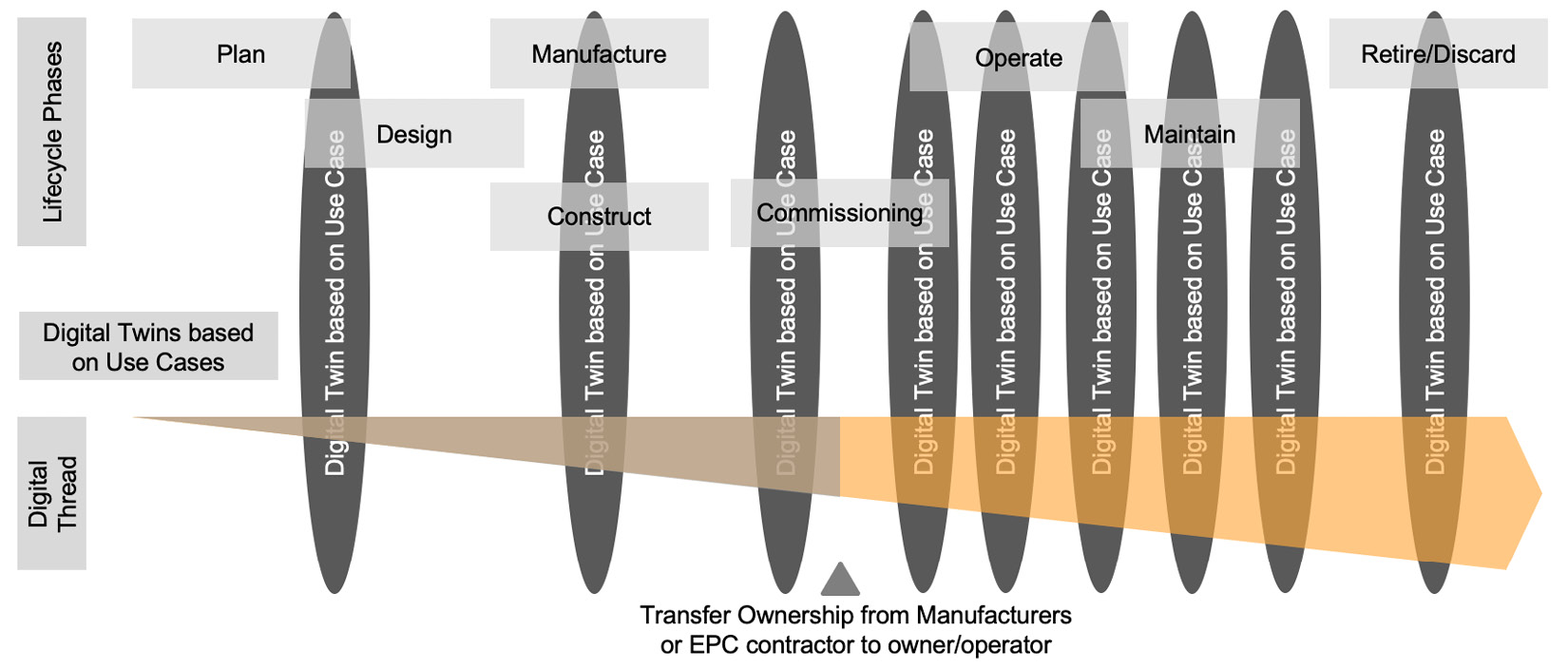
Figure 1.8 – Digital Twin versus Digital Thread
The preceding diagram shows the development of the Digital Thread across the overall life cycle of the entity, where the Digital Twin use cases address specific challenges within one or possibly two life cycle phases. How this is used in practice will be explained in the next section.