When doing linear regression, if we include a variable that is severely correlated with our regressors, we will be inflating our standard errors for those correlated variables. This happens because, if two variables are correlated, the model can't be sure to which one it should be assigning the effect/coefficient. Ridge Regression allows us to model highly correlated regressors, by introducing a bias. Our first thought in statistics is to avoid biased coefficients at all cost. But they might not be that bad after all: if the coefficients are biased but have a much smaller variance than our baseline method, we will be in a better situation. Unbiased coefficients with a high variance will change a lot between different model runs (unstable) but they will converge in probability to the right place. Biased coefficients with a low variance will be quite stable...




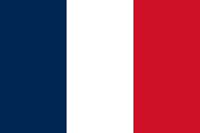


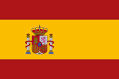



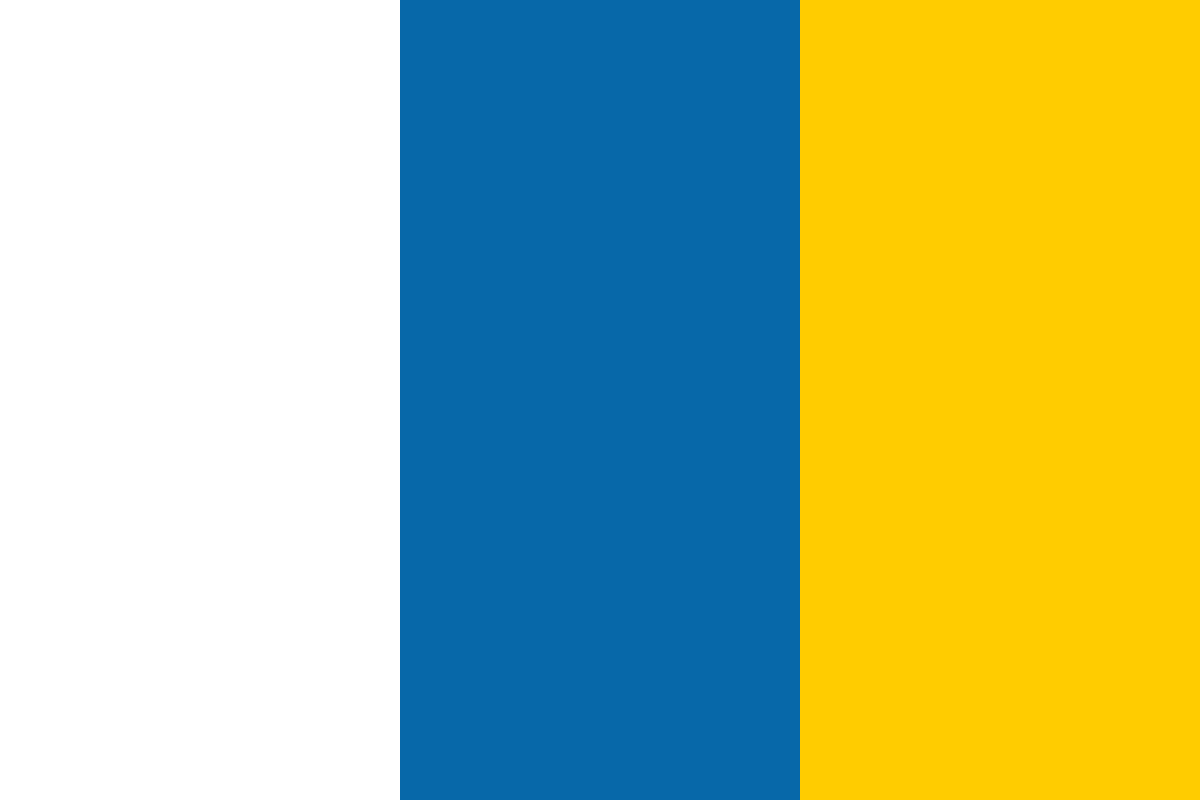
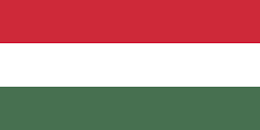

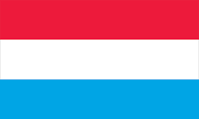





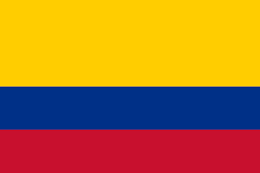
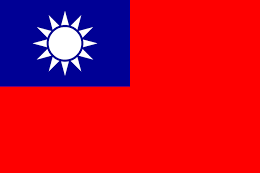
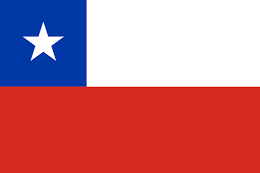



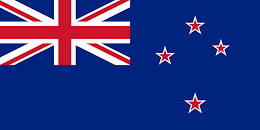
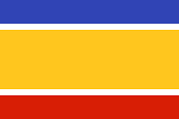
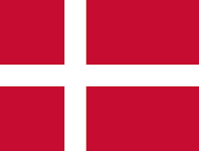
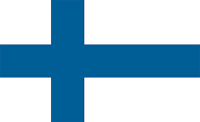







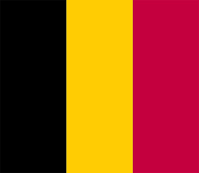

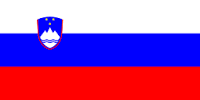





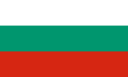


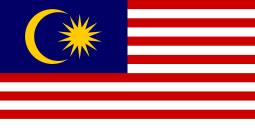



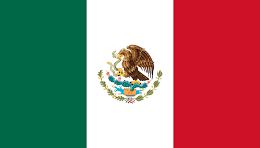
