Generating full factorial designs
Often in scientific computing, we are interested in generating a full factorial design of a computational experiment (see, for example, http://www.socialresearchmethods.net/kb/expfact.php or https://en.wikipedia.org/wiki/Factorial_experiment). A typical application of this design is performing a grid search in hyperparameter tuning of machine learning models (see https://cloud.google.com/ml-engine/docs/tensorflow/hyperparameter-tuning-overview or https://en.wikipedia.org/wiki/Hyperparameter_optimization#Grid_search).
Assume that we are given a list of vectors and we want to generate all possible combinations of values taken from those vectors. For instance, if we have the x=[1,2]
and y=['a', 'b']
vectors, we have four possible combinations of values taken from them, namely, (1,'a')
, (2, 'a')
, (1,'b')
, and (2,'b')
. In general, if we have
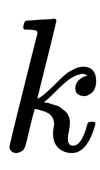
vectors, and vector
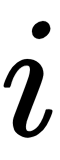
has
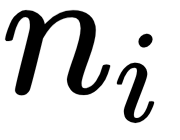
elements, then there are
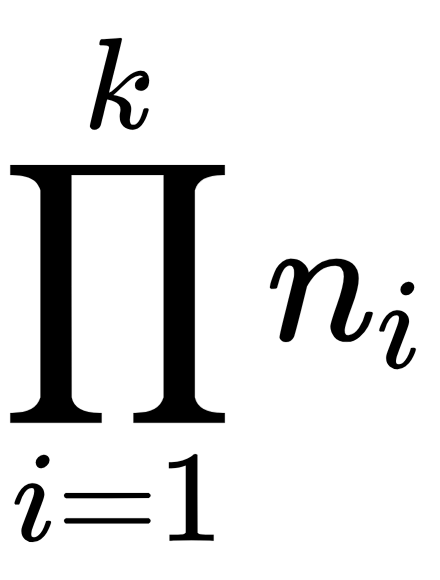
such combinations. In this recipe, we will show how to use the matrix...