As we've seen in our exploration of the Taxi-v2 environment, your agent is a self-driving taxicab whose job it is to pick up passengers from a starting location and drop them off at their desired destination as efficiently as possible. The taxi collects a reward when it drops off a passenger and gets penalties for taking other actions. The following is a rendering of the taxi environment:
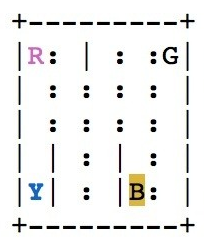
The rewards your agent collects are stored in the Q-table. The Q-table in our model-free algorithm is a lookup table that maps states to actions.
Think of the Q-table as an implementation of a Q-function of the Q form (state, action). The function takes the state we are in and the actions we can take in that state and returns a Q-value. For our purposes, this will be the current highest-valued action the agent has already seen in that state.