The fundamental feature of a Recurrent Neural Network (RNN) is that the network contains at least one feedback connection, so the activations can flow around in a loop. It enables the networks to do temporal processing and learn sequences, for example, perform sequence recognition/reproduction or temporal association/prediction. RNN architectures can have many different forms. One common type consists of a standard multilayer perceptron (MLP) plus added loops. These can exploit the powerful non-linear mapping capabilities of the MLP, and also have some form of memory. Others have more uniform structures, potentially with every neuron connected to all the others, and may also have stochastic activation functions. For simple architectures and deterministic activation functions, learning can be achieved using similar gradient descent procedures to those leading to the backpropagation algorithm for feed-forward networks.
The following figure shows a few of the most important types and features of RNNs:
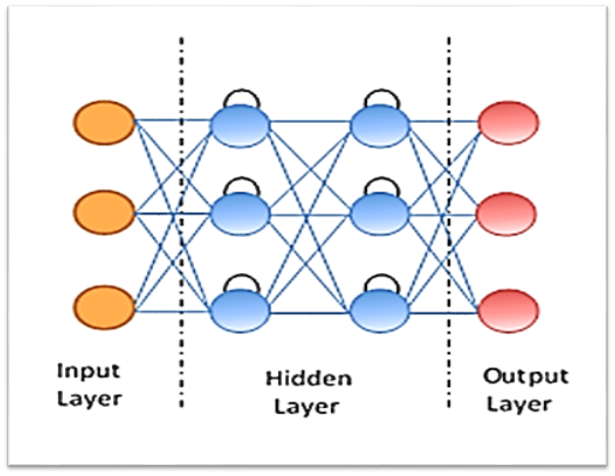