The gradient descent algorithm is not the only optimization algorithm available to optimize our network weights, however it's the basis for most other algorithms. While understanding every optimization algorithm out there is likely a PhD worth of material, we will devote a few sentences to some of the most practical.
Optimization algorithms for deep learning
Using momentum with gradient descent
Using gradient descent with momentum speeds up gradient descent by increasing the speed of learning in directions the gradient has been constant in direction while slowing learning in directions the gradient fluctuates in direction. It allows the velocity of gradient descent to increase.
Momentum works by introducing a velocity term, and using a weighted moving average of that term in the update rule, as follows:

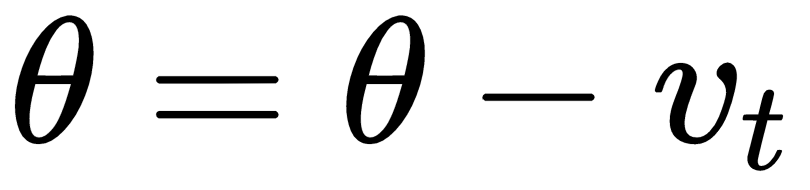
Most typically is set to 0.9 in the case of momentum, and usually this is not a hyper-parameter that needs to be changed.
The RMSProp algorithm
RMSProp is another algorithm that can speed up gradient descent by speeding up learning in some directions, and dampening oscillations in other directions, across the multidimensional space that the network weights represent:
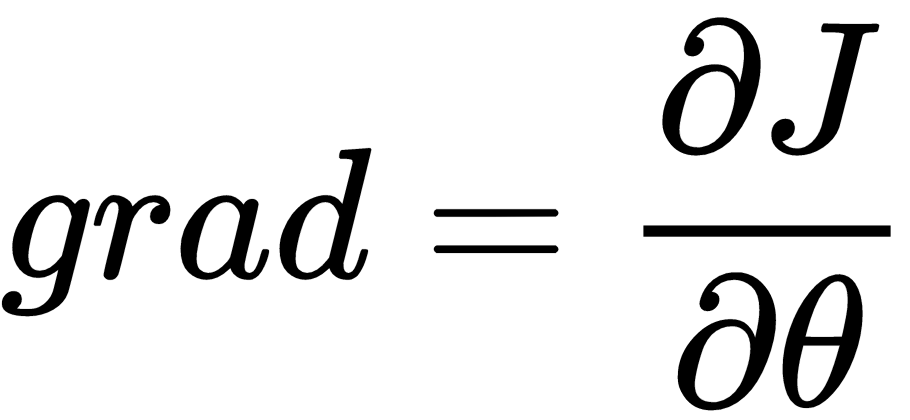

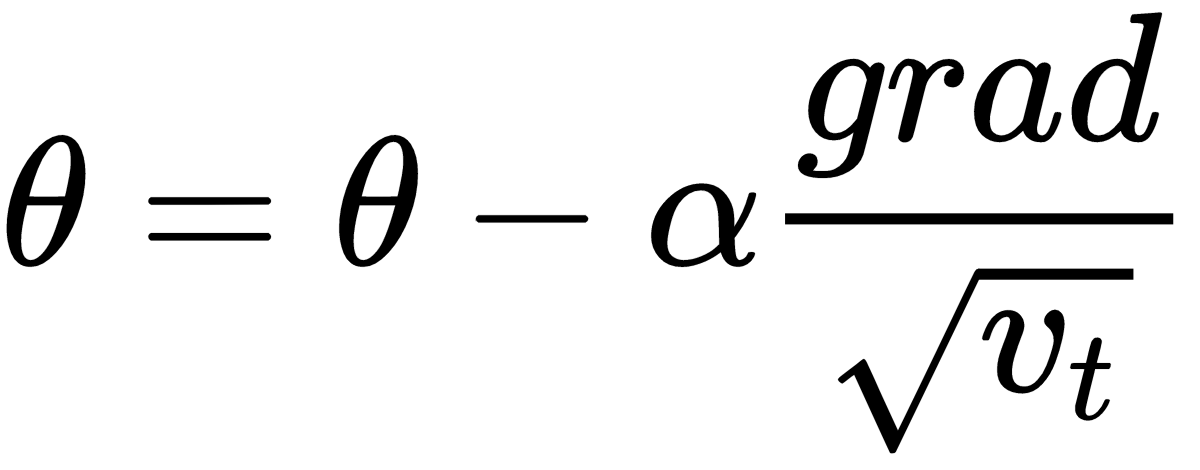
This has the effect of reducing oscillations more in directions where is large.
The Adam optimizer
Adam is one of the best performing known optimizer and it's my first choice. It works well across a wide variety of problems. It combines the best parts of both momentum and RMSProp into a single update rule:
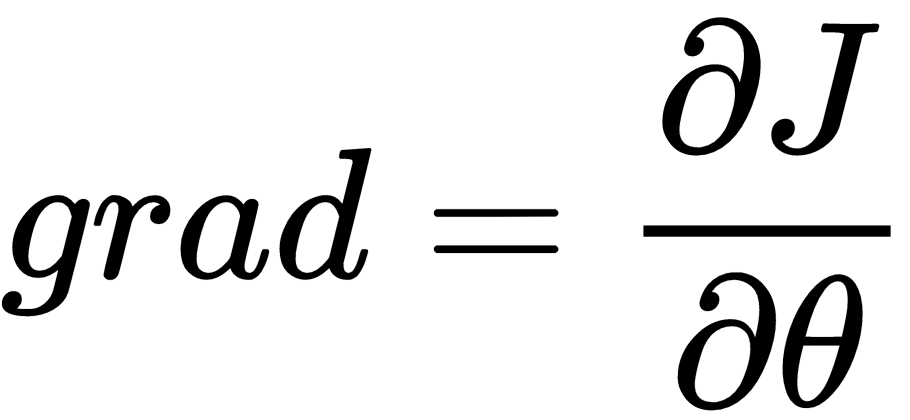


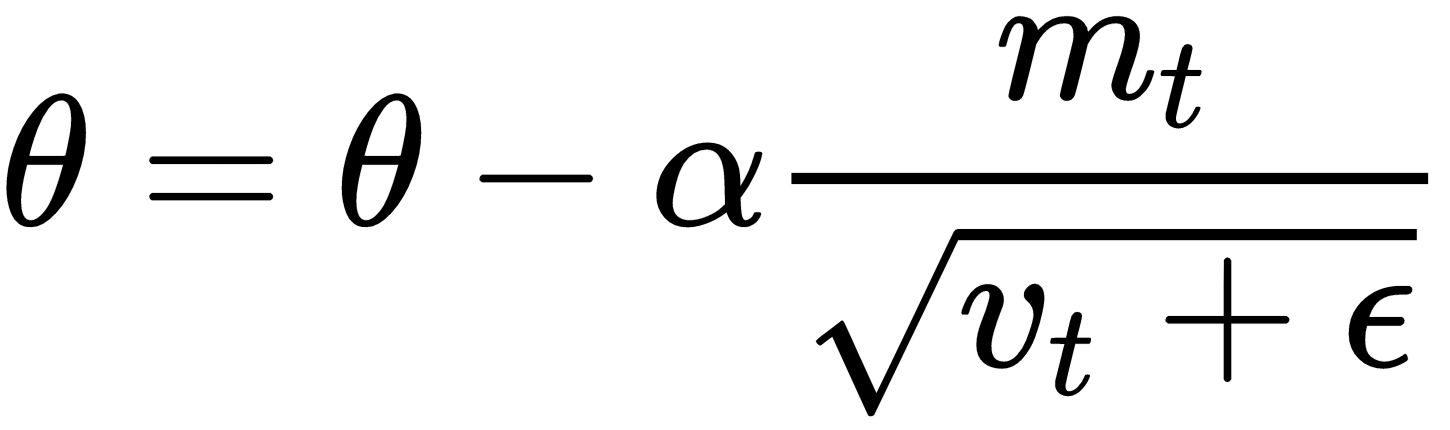
Where is some very small number to prevent division by 0.