Turning data quality into SLAs
Trust is an important element for the consumer. The producer is not always fully aware of the data they are treating. Indeed, the producer can be seen as an executor creating an application on behalf of the consumer. They are not a domain expert and work in a black-box environment: on the one hand, the producer doesn’t exactly understand the objectives of their work, while on the other hand, the consumer doesn’t have control over the supply chain.
This can involve psychological barriers. The consumer and the producer may have never met. For instance, the head of sales, who is waiting for their weekly sales report, doesn’t know the data engineer in charge of the Spark ingestion in the data lake. The level of trust between the different stakeholders can be low from the beginning and the relationship can be poisonous and compromised. This kind of trust issue is difficult to solve, and confidence is dramatically complex to restore. This triggers a negative vortex that can lead to very important effects in the company.
As stated in the Identifying information bias in the data section, there is a fundamental asymmetry of information and responsibilities between producers and consumers. To solve this, data quality must be applied as an SLA between the parties. Let’s delve into this key component of data quality.
An agreement as a starting point
A SLA serves as a contract between the producer and the consumer, establishing the expected data quality level of a data asset. Concretely, with a SLA, the data producer is committed to offering the level of quality the consumer expects from the data source. The goals of the SLA are manifold:
- Firstly, it ensures awareness of the producer on the expected level of quality they must deliver.
- Secondly, the contractual engagement asks the producers to assume their responsibilities regarding the quality of the delivered processes.
- Thirdly, it enhances the trust of the consumer in the outcomes of the pipeline, easing the burden of cumbersome double checks. The consumer puts their confidence in the contract.
In essence, data quality can be viewed as an SLA between the data producer and the consumer of the data. The SLA is not something you measure by nature, but this will drive the team’s ability to define proper objectives, as we will see in the next section.
The incumbent responsibilities of producers
To support those SLAs, the producer must establish SLOs. Those objectives are targets the producer sets to meet the requirements of the SLA.
However, it is important to stress that those quality targets can be different for each contract. Let’s imagine a department store conducting several data science projects. The marketing team oversees two data products based on the central data lake of all the store sales. The first one is a machine learning model that forecasts the sales of the latest children’s toy, while the second one is a report of all cosmetic products. The number of kids in the household may be an important feature for the first model, while it won’t be of any importance for the second report. The SLAs that are linked to the first project are different from the SLAs of the second one. However, the producer is responsible for providing both project teams with a good set of data. This said, the producer has to summarize all agreements to establish SLOs that will fulfill all their commitments.
This leads to the notion of vertical SLAs versus transversal SLOs. The SLA is considered vertical as it involves a one-to-one relationship between the producer and the consumer. In contrast, the SLO is transversal as, for a specific dataset, a SLO will be used to fulfill one or several SLAs. Figure 1.11 illustrates these principles. The producer is engaged in a contractual relationship with the customer, while the SLO directs the producers’ monitoring plan. We can say that a data producer, for a specific dataset, has a unique set of SLOs that fit all the SLAs:
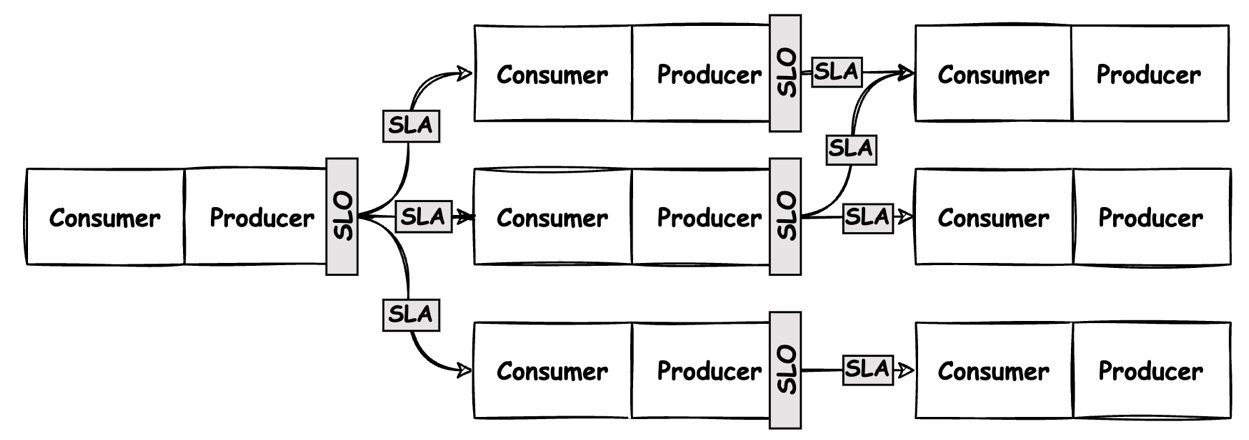
Figure 1.11 – SLAs and SLOs in a data pipeline
The agreement will concern a bilateral relationship between a producer and their consumer. An easy example is as follows: “To run my churn prediction model, I need all the transactions performed by 18+ people in the last 2 weeks.” In this configuration, the data producer knows that the data of the CRM must contain all transactions from the last 2 weeks of people aged 18 and over. They can now create the following objectives:
- The data needs to contain transactions from the last 2 weeks
- The data needs to contain transactions of customers who are 18 and over
We’ll see how indicators can be set to validate the SLO later.
The SLA is a contract between both parties, whereas the SLO is set by the producer to ensure they can respect all SLAs. This means that an SLO can serve several agreements. If another project requires the transaction data of 18+ customers for 3 weeks, the data team could easily adjust its target and set the limit to 3.
The advantage of thinking of data quality as a service is that, by knowing which dimension of quality your consumers need, you can provide the right adjusted level of service to achieve the objectives. As we’ll see later, it will help a lot regarding costs and resource optimization.
Considerations for SLOs and SLAs
To ensure SLAs and SLOs remain effective and relevant, it is important to focus on different topics.
First, as business requirements change over time, it is essential for both parties to regularly review the existing agreements and adjust objectives accordingly. Collaboration and communication are key to ensuring the objectives are always well aligned with consumer expectations.
Documentation and traceability are also important when it comes to agreements and objectives. By documenting those, all parties can maintain transparency and keep track of changes over time. This can be helpful in case of misalignment or misunderstandings.
Also, to encourage producers to respect their agreements, incentives and penalties can be activated, regardless of whether the agreements are met.
What is important and at the core of the SLA/SLO framework is being able to measure the performance against the established SLOs. It is crucial to set up metrics that will assess the data producer’s performance.
To be implemented, objectives have to be aligned with indicators. In the next section, we will see which indicators are important for measuring data quality.