Troubleshooting and repairing failures in Databricks Workflows
With Databricks Workflows, you can create, manage, and track multi-task workflows for ETL, analytics, and machine learning pipelines. Your data teams can leverage various task types, rich observability features, and high dependability to improve the automation and coordination of any pipeline and boost their efficiency. However, sometimes things can go wrong and your workflows may fail due to various reasons, such as data quality issues, code errors, resource constraints, or external dependencies.
In this recipe, you will learn how to repair common failures in Databricks Workflows, using the tools and best practices provided by the Databricks platform.
How to do it...
- Go to the workflow: Click on Workflows in the sidebar, then click on a job name in the Name column.
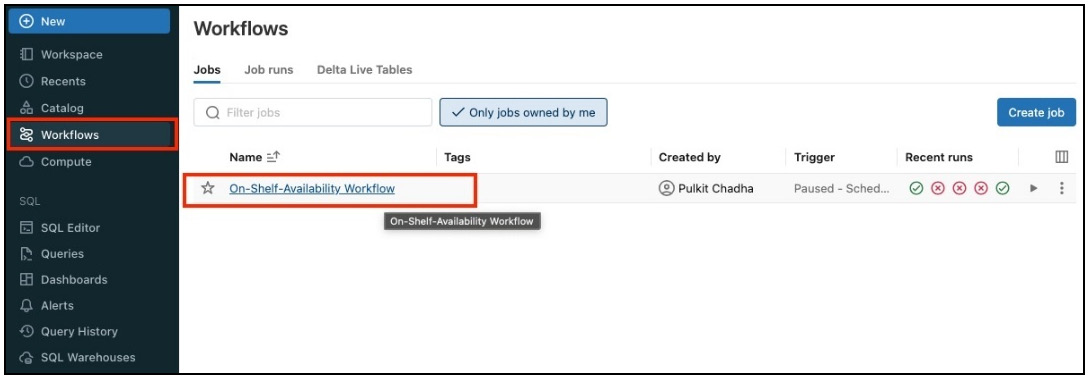
Figure 8.33 – The Databricks Workflows jobs list view
- Job run details: The Runs tab displays the...