Index
When constructing both the pd.Series
and pd.DataFrame
objects in the previous sections, you likely noticed the values to the left of these objects starting at 0 and incrementing by 1 for each new row of data. The object responsible for those values is the pd.Index
, highlighted in the following image:
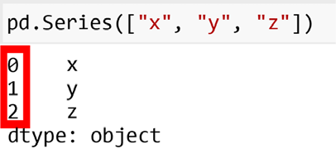
Figure 1.1: Default pd.Index, highlighted in red
In the case of a pd.DataFrame
, you have a pd.Index
not only to the left of the object (often referred to as the row index or even just index) but also above (often referred to as the column index or columns):
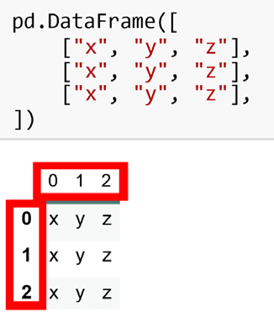
Figure 1.2: A pd.DataFrame with a row and column index
Unless explicitly provided, pandas will create an auto-numbered pd.Index
for you (technically, this is a pd.RangeIndex
, a subclass of the pd.Index
class). However, it is very rare to use pd.RangeIndex
for your columns, as referring to a column named City
or Date
is more expressive than referring to a column in the nth position. The pd.RangeIndex
appears more commonly in the row index, although you may still want custom labels to appear there as well. More advanced selection operations with the default pd.RangeIndex
and custom pd.Index
values will be covered in Chapter 2, Selection and Assignment, to help you understand different use cases, but for now, let’s just look at how you would override the construction of the row and column pd.Index
objects during pd.Series
and pd.DataFrame
construction.
How to do it
When constructing a pd.Series
, the easiest way to change the row index is by providing a sequence of labels to the index=
argument. In this example, the labels dog
, cat
, and human
will be used instead of the default pd.RangeIndex
numbered from 0 to 2:
pd.Series([4, 4, 2], index=["dog", "cat", "human"])
dog 4
cat 4
human 2
dtype: int64
If you want finer control, you may want to construct the pd.Index
yourself before passing it as an argument to index=
. In the following example, the pd.Index
is given the name animal
, and the pd.Series
itself is named num_legs
, providing more context to the data:
index = pd.Index(["dog", "cat", "human"], name="animal")
pd.Series([4, 4, 2], name="num_legs", index=index)
animal
dog 4
cat 4
human 2
Name: num_legs, dtype: int64
A pd.DataFrame
uses a pd.Index
for both dimensions. Much like with the pd.Series
constructor, the index=
argument can be used to specify the row labels, but you now also have the columns=
argument to control the column labels:
pd.DataFrame([
[24, 180],
[42, 166],
], columns=["age", "height_cm"], index=["Jack", "Jill"])
age height_cm
Jack 24 180
Jill 42 166