Milestone 7 – Executing the training loops
To begin training, just run the following command:
trainer.train()
Figure 4.1 shows an example of the output you can expect to see from the trainer.train()
command’s execution:
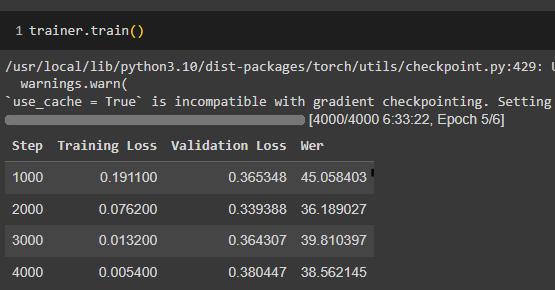
Figure 4.1 – Sample output from trainer.train() in Google Colab
Each training batch will have an evaluation step that calculates and displays training/validation losses and WER metrics. Depending on your GPU, training could take 5–10 hours. If you run into memory issues, try reducing the batch size and adjusting gradient_accumulation_steps
in the declaration of Seq2SeqTrainingArguments
.
Because of the parameters we established when declaring Seq2SeqTrainingArguments
, our model metrics and performance will be pushed to the Hugging Face Hub with each training iteration. The key parameters driving that push to the Hub are shown here:
from transformers import Seq2SeqTrainingArguments training_args = Seq2SeqTrainingArguments...