After each training iteration, the network's efficiency is measured by evaluating the model against a set of evaluation metrics. We optimize the model further on upcoming training iterations based on the evaluation metrics. We use the test dataset for evaluation. Note that we are performing binary classification for the given use case. We predict the chances of that patient surviving. For classification problems, we can plot a Receiver Operating Characteristics (ROC) curve and calculate the Area Under The Curve (AUC) score to evaluate the model's performance. The AUC score ranges from 0 to 1. An AUC score of 0 represents 100% failed predictions and 1 represents 100% successful predictions.




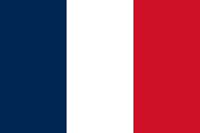


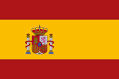



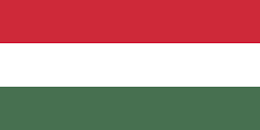

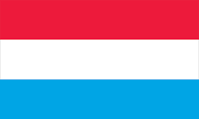





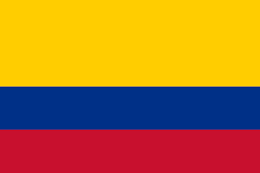
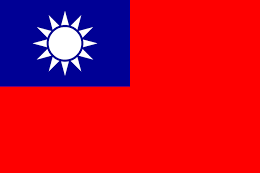
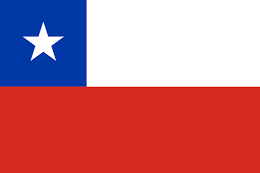



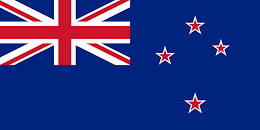
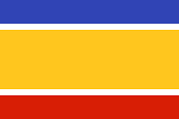
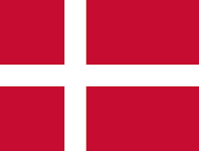
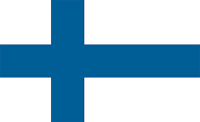







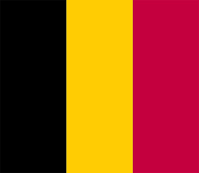

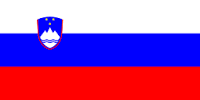





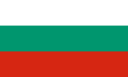


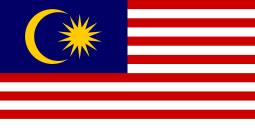



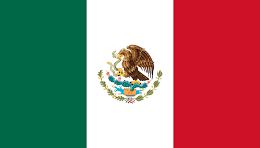
