Analyzing why AI projects fail slowly
Data scientists often come from research backgrounds and approach the work of building machine learning models methodically. After obtaining a business problem and determining what an AI solution looks like, the process looks like this:
- Gather the data.
- Cleanse the data.
- Transform the data.
- Build a machine learning model.
- Determine whether the model is acceptable.
- Tune the hyperparameters.
- Deploy the model.
If the model is acceptable in step 5, data scientists will proceed to step 6. If the model is unacceptable, they will return to step 3 and try different models and transformations. This process can be seen in Figure 1.2:
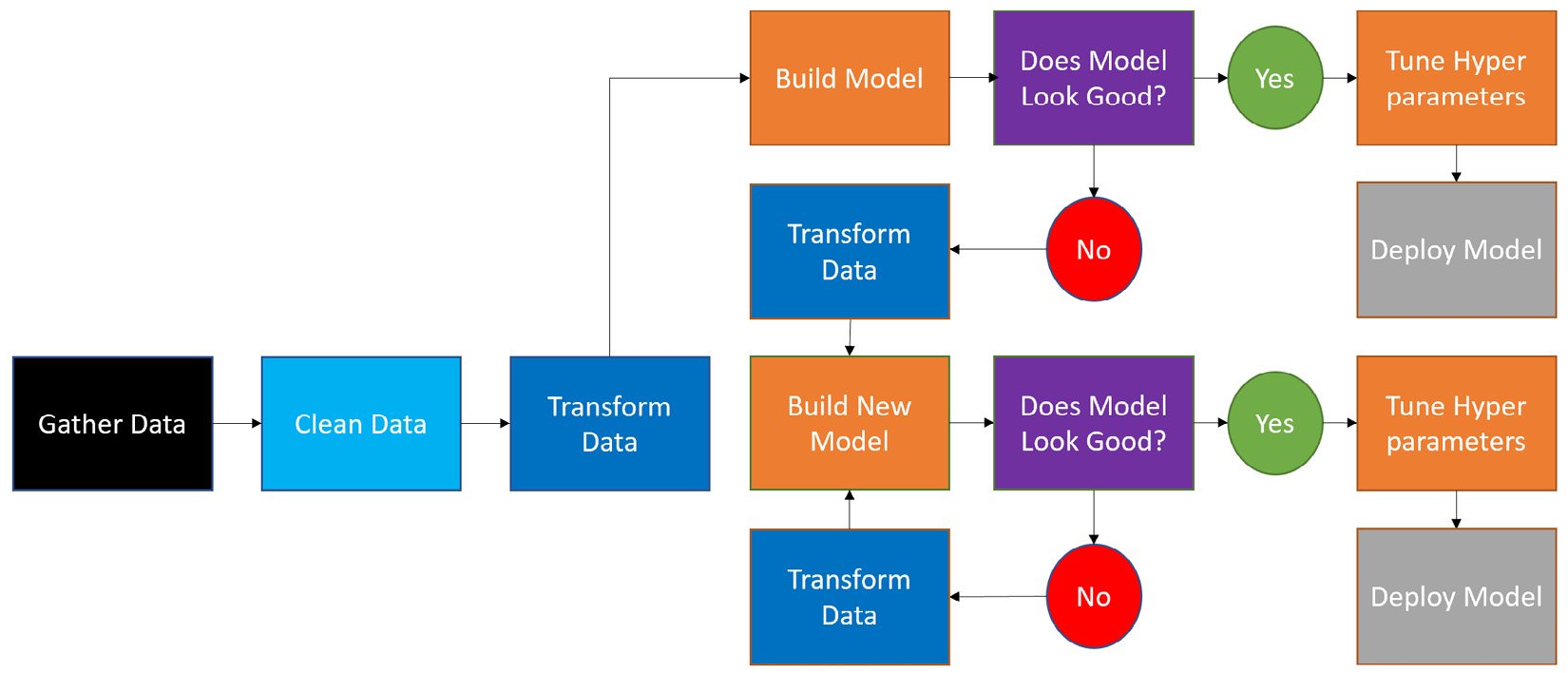
Figure 1.2 – Traditional approach to building a machine learning solution
While this process follows the five steps outlined in Figure 1.1, it's long and cumbersome. There are also specific drawbacks related to transforming data, building models, tuning hyperparameters, and deploying models. We will now take a closer look at the drawbacks inherent in this process that cause data science projects to fail slowly instead of quickly, greatly impacting the ROI problem:
- Data has to be transformed differently by algorithms. Data transformation can be a tedious process. Learning how to fill in null values, one-hot encode categorical variables, remove outliers, and scale datasets appropriately isn't easy and takes years of experience to master. It also takes a lot of code, and it's easy for novice programmers to make mistakes, especially when learning a new programming language.
Furthermore, different data transformations are required for different algorithms. Some algorithms can handle null values while others cannot. Some can handle highly imbalanced datasets, those in which you only have a few samples of a category you're trying to predict, while other algorithms break.
One algorithm may perform well if you remove outliers while another will be completely unaffected. Whenever you choose to try a new model, there's a high likelihood that you will need to spend time reengineering your data for your algorithm.
- Some algorithms require hyperparameter tuning to perform well. Unlike Random Forest, models built with algorithms such as XGBoost and LightGBM only perform well if you tune their hyperparameters, but they can perform really well. Â
Thus, you have two choices: stick to models that perform decently without tuning or spend days to weeks tuning a model with high potential but no guarantee of success. Furthermore, you need a massive amount of algorithm-specific knowledge to become a successful data scientist using the traditional approach.
- Hyperparameter tuning takes a lot of compute hours. Days to weeks to train a single machine learning model may seem like an exaggeration, but in practice, it's common. At most companies, GPUs and CPUs are a limited resource, and datasets can be quite large. Certain companies have lines to get access to computing power, and data scientists require a lot of it.
When hyperparameter tuning, it's common to do something called a grid search where every possible combination of parameters is trained over a specified numerical space. For example, say algorithm X has parameters A, B, and C, and you want to try setting A to 1, 2, and 3, B to 0 and 1, and C to 0.5, 1, and 1.5. Tuning this model requires building 3 x 2 x 3 = 15 machine learning models to find the ideal combination.
Now, 15 models may take a few minutes or a few days to train depending on the size of the data you are using, the algorithm you wish to employ, and your computing capacity. However, 15 models is very little. Many modern machine learning algorithms require you to tune five to six parameters over a wide range of values, producing hundreds to thousands of models in search of finding the best performance.
- Deploying models is hard and it isn't taught in school. Even after you transform data correctly and find the perfect model with the best set of hyperparameters, you still need to deploy it to be used by the business. When new data comes in, it needs to get scored by the model and delivered somewhere. Monitoring is necessary as machine learning models do malfunction occasionally and must be retrained. Yet, data scientists are rarely trained in model deployment. It's considered more of an IT function.
As a result of this lack of training, many companies use hacky infrastructures with an array of slapped-together technologies for machine learning deployment. A database query may be triggered by third-party software on a trigger. Data may be transformed using one computer language, stored in a file on a file share, and picked up by another process that scores the model in another language and saves it back to the file share. Fragile, ad hoc solutions are the norm, and for most data scientists, this is all on-the-job training.
- Data scientists focus on accuracy instead of explainability. Fundamentally, data scientists are trained to build the most accurate AI they can. Kaggle competitions are all about accuracy and new algorithms are judged based on how well they perform compared to solutions of the past.
Businesspeople, on the other hand, often care more about the why behind the prediction. Forgetting to include explainability undermines the trust end users need to place in machine learning, and as a result, many models end up unused.
All in all, building machine learning models takes a lot of time, and when 87% of AI projects fail to make it to production, that's a lot of time wasted. Gathering data and cleansing data are processes that, by themselves, take a lot of time.
Transforming data for each model, tuning hyperparameters, and figuring out and implementing a deployment solution can take even longer. In such a scenario, it's easy to focus on finding the best model possible and overlook the most important part of the project: earning the trust of your end users and ensuring your solution gets used. Luckily, there's a solution to a lot of these problems.