



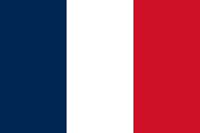


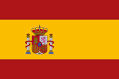



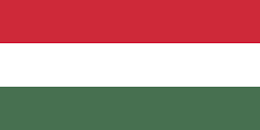

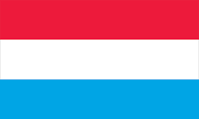





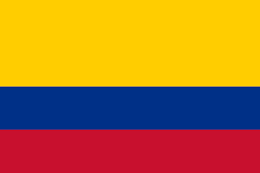
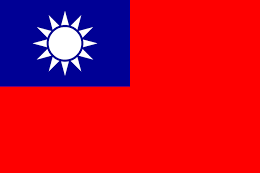
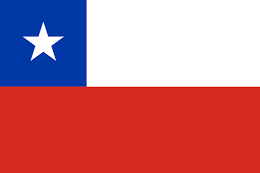



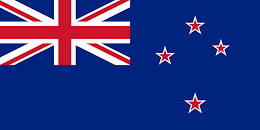
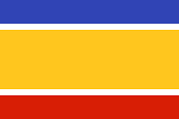
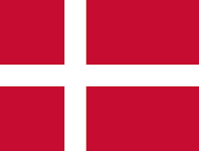
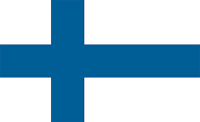







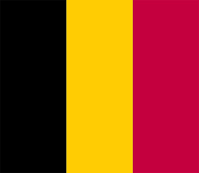

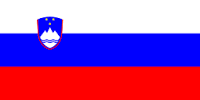





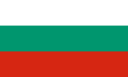


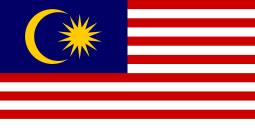



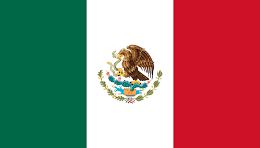

The fastMRI team has come out with baseline models for ML-based image reconstruction from k-space data subsampled at 4x and 8x scan accelerations. A common challenge faced by AI researchers in the field of MR reconstruction is consistency, as they use a variety of datasets for training AI systems. This is why the latest and the largest open source MRI dataset will help tackle this problem of MR image reconstruction by providing an industry-wide and benchmark ready dataset.
This dataset comprises approximately 1.5 million MR images drawn from 10,000 scans, as well as raw measurement data from nearly 1,600 scans. NYU fully anonymized the data set, as well as the metadata and image content manually. It includes the k-space data collected during scanning. NYU School of Medicine has decided to offer researchers with unprecedented access to data so that they can easily train their models, validate their performance, and get a general idea on how image reconstruction techniques could be used in real-world conditions. The k-space data in this data set is derived from MR devices comprising multiple magnetic coils. It also comprises data simulating the measurements from single-coil machines.
FastMRI team mainly focused on two tasks, namely, single-coil reconstruction and multi-coil reconstruction. In both the single-coil and multi-coil deep learning baselines, the AI models are based on u-nets, a convolutional network architecture developed specifically for image segmentation in biomedical applications. U-nets also has a proven track record with an image-to-image prediction. Moreover, a baseline for both classical and non-AI based reconstruction methods has been developed. A separate baseline comprising deep learning models has also been created.
Apart from that, FAIR has created a leaderboard for the consistent measurement of MR progress and reconstruction results. The team has already added the baseline models to start with. Researchers can further add improved results as they begin generating and submitting the results to conferences and journals with the help of the fastMRI data set. It will also help the researchers in evaluating their results against the consistent metrics and to figure out how different approaches compare.
“Our priority for the next phase of this collaboration is to use the experimental foundations we’ve established — the data and baselines — to further explore AI-based image reconstruction techniques. Additionally, any progress that we make at FAIR and NYU School of Medicine will be part of a larger collaboration that spans multiple research communities” says the FAIR team.
For more information, check out the official blog post.
Facebook AI researchers investigate how AI agents can develop their own conceptual shared language
Facebook plans to change its algorithm to demote “borderline content” that promotes misinformation and hate speech on the platform
Babysitters now must pass Perdictim’s AI assessment to be “perfect” to get the job