When analyzing data we'll frequently encounter unusual cases, outliers, and anomalies. Those cases are different from the majority and they don't match the pattern that the rest of the cases fit in. Sometimes, we might want to identify them in order to remove them from the analysis, because they can skew our results. In other cases, we might be interested in analyzing them. Either way, it's very important to know how to deal with them properly. In Chapter 11, Forecasting with Tableau, the Forecasting on a dataset with outliers recipe taught up how to deal with outliers on one dimension, which is relatively simple. But when we have more than one dimension, things get much more complicated. In this recipe, we'll learn how to deal with multidimensional outliers.




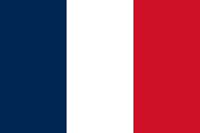


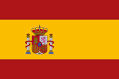



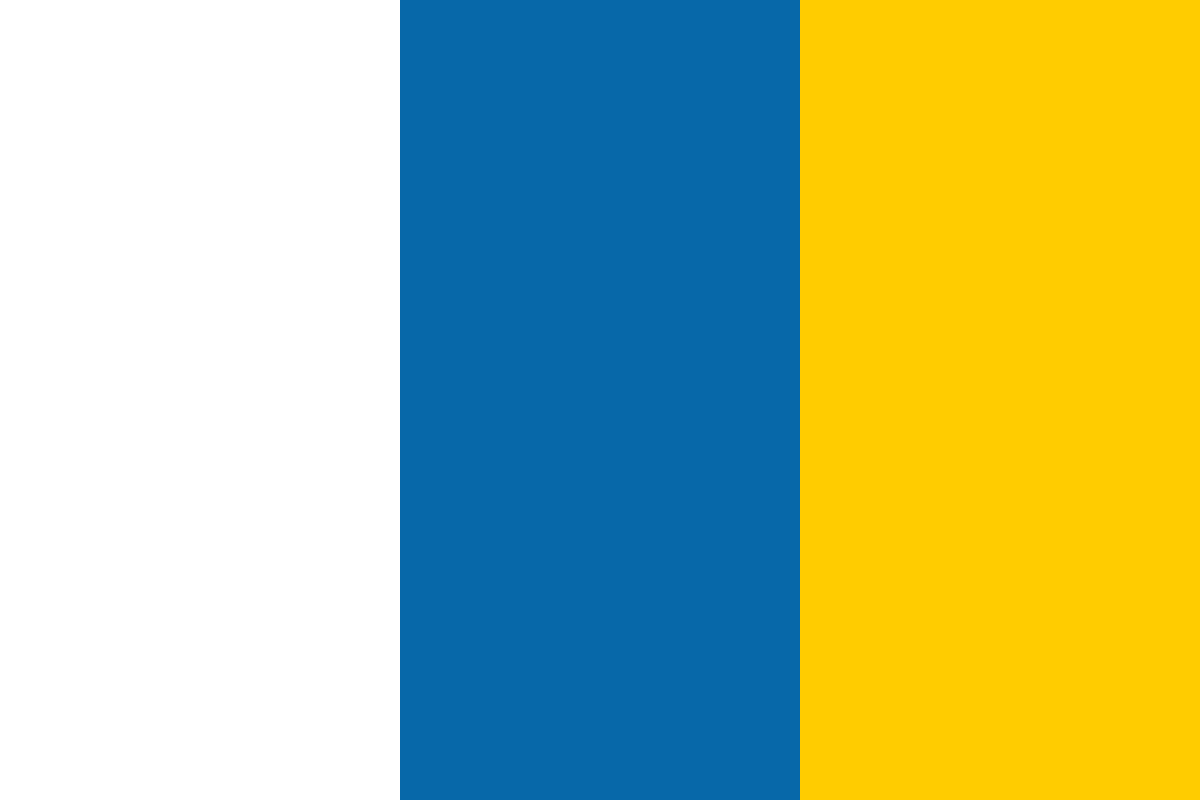
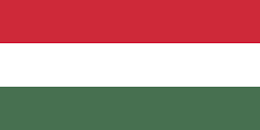

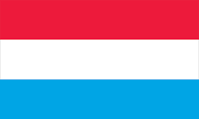





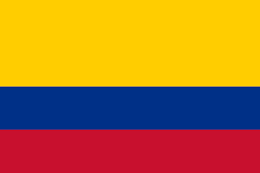
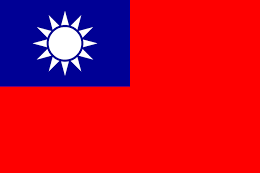
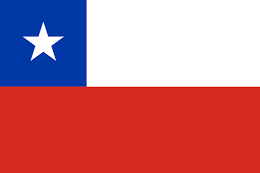



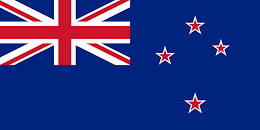
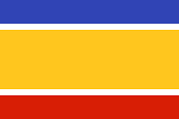
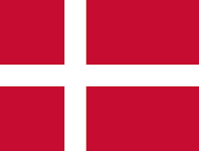
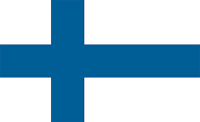







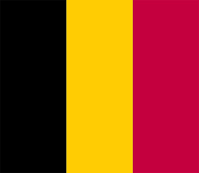

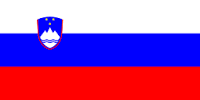





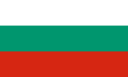


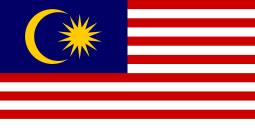



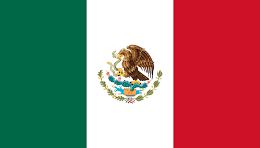
