We have already spent quite some time on creating the entire pipeline and tuning the models to achieve better performance. However, what is equally—or even more—important is the model's interpretability: so, not only giving an accurate prediction but also being able to explain the why. In the case of customer churn, an accurate model is important. However, knowing what are the actual predictors of the customers leaving might be helpful in improving the overall service and, potentially, making them stay longer. In a financial setting, banks often use machine learning in order to predict a customer's ability to repay credit. And, in many cases, they are obliged to justify their reasoning, in case they decline a credit application—why exactly this customer's application was not approved. In the case of very...




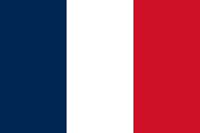


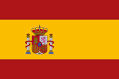



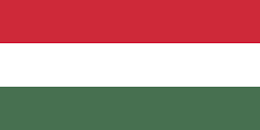

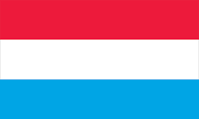





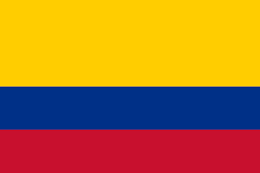
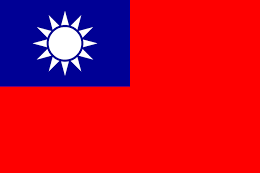
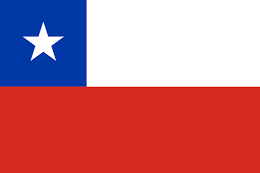



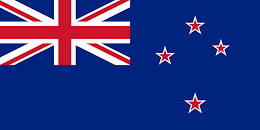
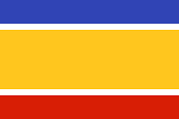
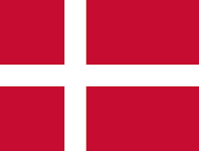
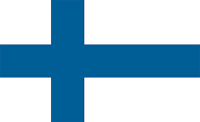







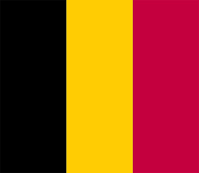

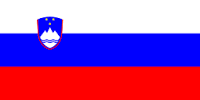





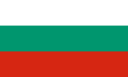


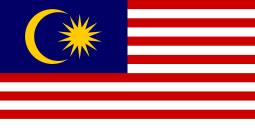



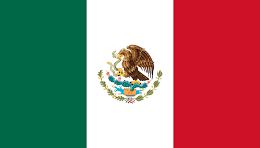
