Using neural networks in data science
An Artificial Neural Network (ANN), which we will call a neural network, is based on the neuron found in the brain. A neuron is a cell that has dendrites connecting it to input sources and other neurons. Depending on the input source, a weight allocated to a source, the neuron is activated, and then fires a signal down a dendrite to another neuron. A collection of neurons can be trained to respond to a set of input signals.
An artificial neuron is a node that has one or more inputs and a single output. Each input has a weight assigned to it that can change over time. A neural network can learn by feeding an input into a network, invoking an activation function, and comparing the results. This function combines the inputs and creates an output. If outputs of multiple neurons match the expected result, then the network has been trained correctly. If they don't match, then the network is modified.
A neural network can be visualized as shown in the following figure, where Hidden Layer is used to augment the process:
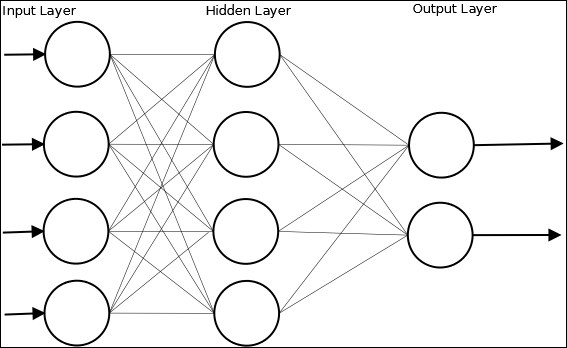
In Chapter 7, Neural Networks, we will use the Weka
class, MultilayerPerceptron
, to illustrate the creation and use of a Multi Layer Perceptron (MLP) network. As we will explain, this type of network is a feedforward neural network with multiple layers. The network uses supervised learning with backpropagation. The example uses a dataset called dermatology.arff
that contains 366 instances that are used to diagnose erythemato-squamous diseases. It uses 34 attributes to classify the disease into one of the five different categories.
The dataset is split into a training set and a testing set. Once the data has been read, the MLP instance is created and initialized using the method to configure the attributes of the model, including how quickly the model is to learn and the amount of time spent training the model.
String trainingFileName = "dermatologyTrainingSet.arff"; String testingFileName = "dermatologyTestingSet.arff"; try (FileReader trainingReader = new FileReader(trainingFileName); FileReader testingReader = new FileReader(testingFileName)) { Instances trainingInstances = new Instances(trainingReader); trainingInstances.setClassIndex( trainingInstances.numAttributes() - 1); Instances testingInstances = new Instances(testingReader); testingInstances.setClassIndex( testingInstances.numAttributes() - 1); MultilayerPerceptron mlp = new MultilayerPerceptron(); mlp.setLearningRate(0.1); mlp.setMomentum(0.2); mlp.setTrainingTime(2000); mlp.setHiddenLayers("3"); mlp.buildClassifier(trainingInstances); ... } catch (Exception ex) { // Handle exceptions }
The model is then evaluated using the testing data:
Evaluation evaluation = new Evaluation(trainingInstances); evaluation.evaluateModel(mlp, testingInstances);
The results can then be displayed:
System.out.println(evaluation.toSummaryString());
The truncated output of this example is shown here where the number of correctly and incorrectly identified diseases are listed:
Correctly Classified Instances 73 98.6486 % Incorrectly Classified Instances 1 1.3514 %
The various attributes of the model can be tweaked to improve the model. In Chapter 7, Neural Networks, we will discuss this and other techniques in more depth.