GANs are neural networks that learn to generate data similar to real data, or the data in the training set. The key idea of a GAN is to have a generator network and a discriminator network playing against each other: the generator tries to generate data that looks like real data, while the discriminator tries to tell whether the generated data is real (from the known real data) or fake (generated by the generator). The generator and the discriminator are trained together, and during the training process, the generator learns to generate data that looks more and more like real data, while the discriminator learns to distinguish real data from fake data. The generator learns by trying to make the discriminator's probability of output being real data, when fed with the generator's output as the discriminator's input, as close to 1.0 as possible...




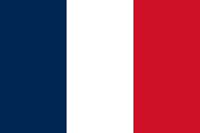


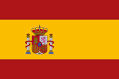



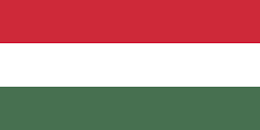

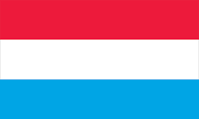





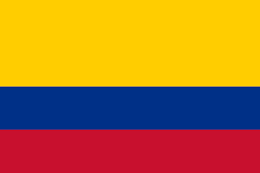
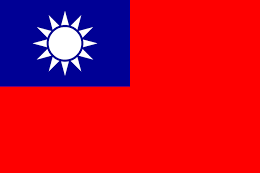
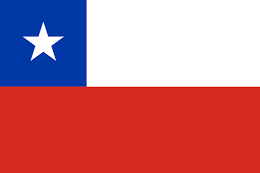



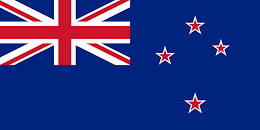
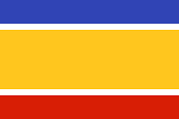
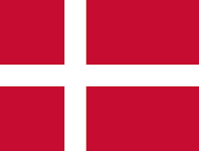
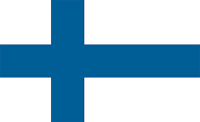







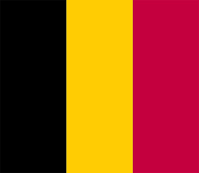

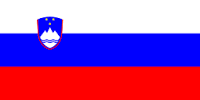





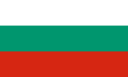


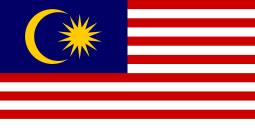



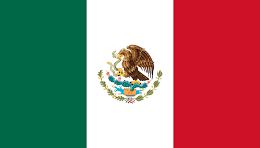
