In DQN architecture, we use experience replay to remove correlations between the training samples. However, uniformly sampling transitions from the replay memory is not an optimal method. Instead, we can prioritize transitions and sample according to priority. Prioritizing transitions helps the network to learn swiftly and effectively. How do we prioritize the transitions? We prioritize the transitions that have a high TD error. We know that a TD error specifies the difference between the estimated Q value and the actual Q value. So, transitions with a high TD error are the transition we have to focus on and learn from because those are the transitions that deviate from our estimation. Intuitively, let us say you try to solve a set of problems, but you fail in solving two of these problems. You then give priority to those two problems alone to focus...




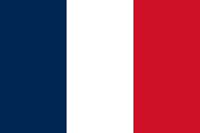


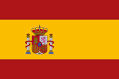



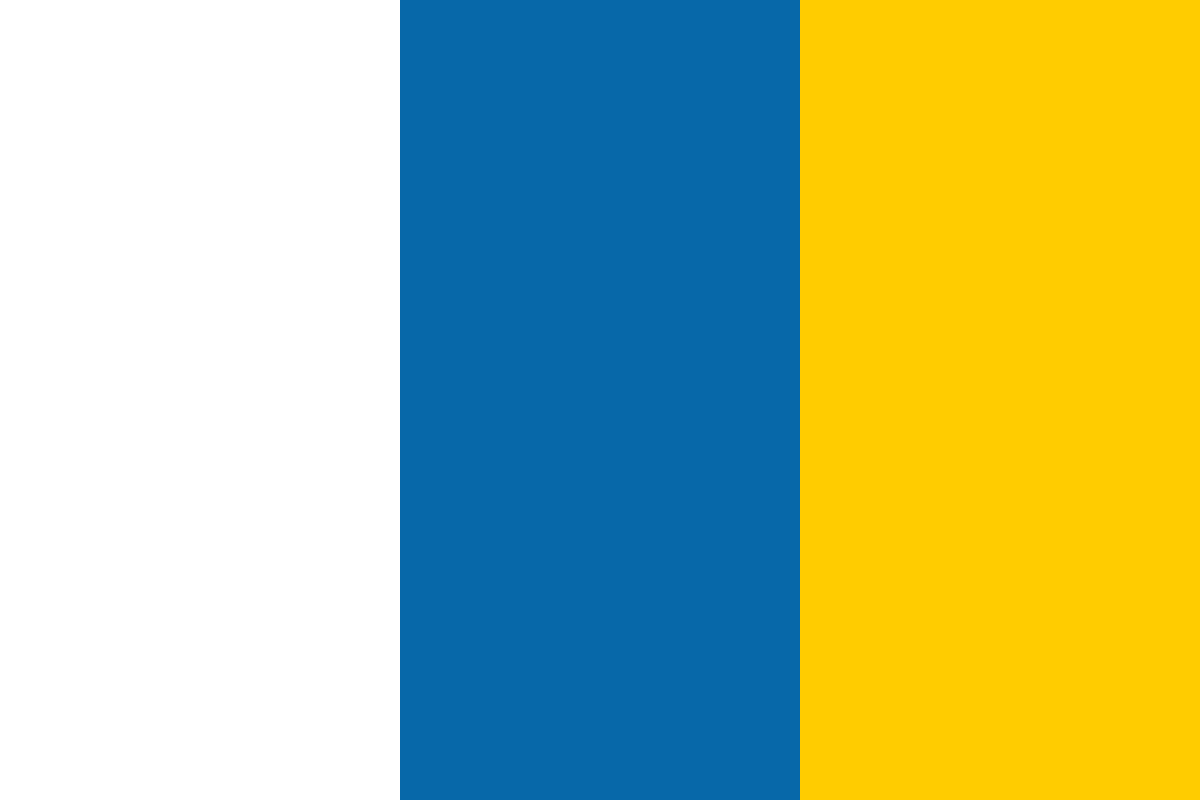
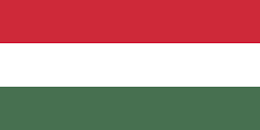

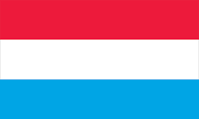





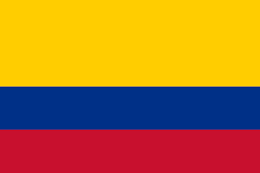
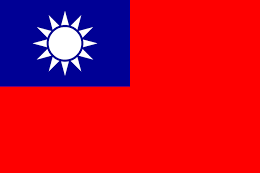
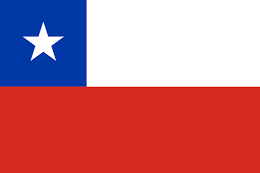



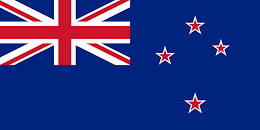
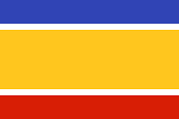
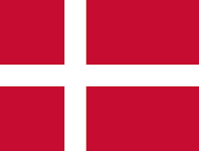
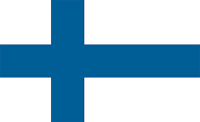







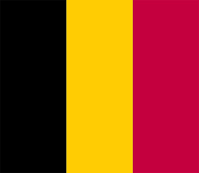

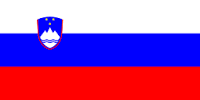





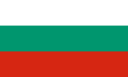


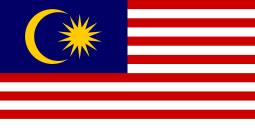



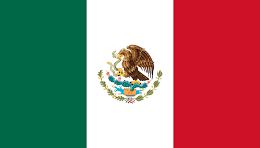
