Humans' conceptual learning tends to differ from machine learning in two major aspects. Consider an example of handwritten digits from a large vocabulary in the following diagram:
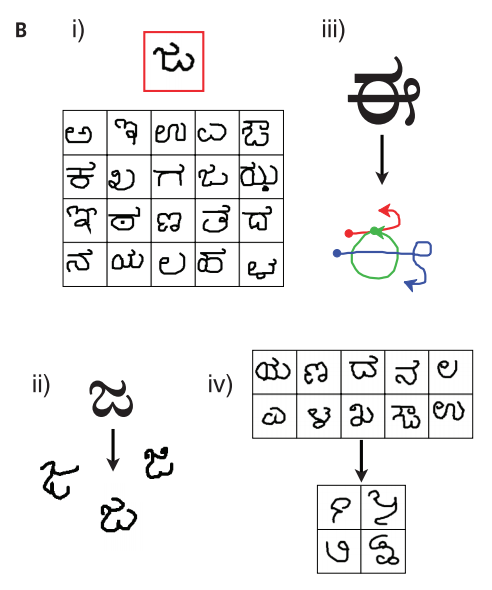
Firstly, people tend to learn meaningful information about objects, for example, object boundaries, from just one or a few examples and classify them with high accuracy (refer to the i) part in the preceding diagram). On the other hand, deep learning models need lots of labeled data to achieve human-level performance on tasks such as object recognition.
Secondly, humans learn a vast majority of functions from just one example, for example, creating new characters (refer to the ii) part in the preceding diagram), decomposing objects/characters into various parts and relations (refer to the iii) part in the preceding diagram), and developing new, meaningful concepts...