In the last chapter, we revisited the Q-learning algorithm and implemented the Q_Learner class. For the Mountain car environment, we used a multi-dimensional array of shape 51x51x3 to represent the action-value function,. Note that we had discretized the state space to a fixed number of bins given by the NUM_DISCRETE_BINS configuration parameter (we used 50) . We essentially quantized or approximated the observation with a low-dimensional, discrete representation to reduce the number of possible elements in the n-dimensional array. With such a discretization of the observation/state space, we restricted the possible location of the car to a fixed set of 50 locations and the possible velocity of the car to a fixed set of 50 values. Any other location or velocity value would be approximated to one of those fixed set of values. Therefore, it is possible...




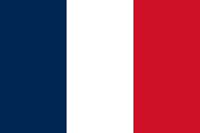


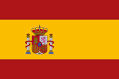



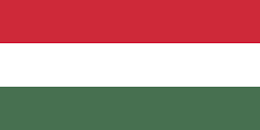

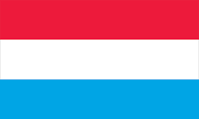





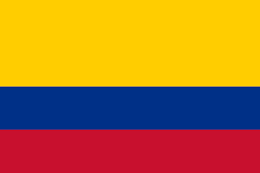
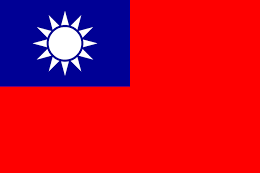
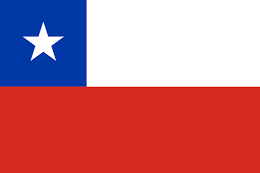



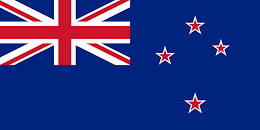
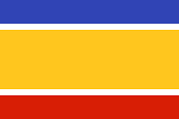
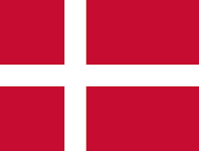
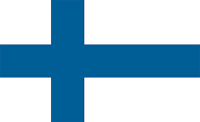







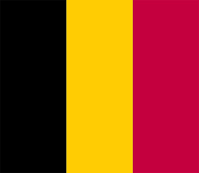

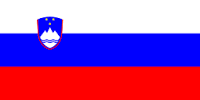





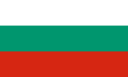


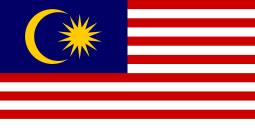



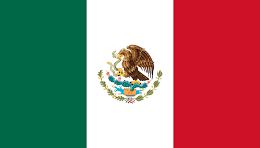
