Throughout this book, we have understood how DL can address several problems in computer vision, natural language processing, and time series forecasting. This combination of DL with RL can lead to more astonishing applications to solve more complex problems. But what is RL? It is a specific area of ML, where agents have to take action to maximize the reward in a given environment. The term reinforcement comes from the similarity of this learning process to what happens when children are incentivized by sweets; the RL algorithms are rewarded when making the right decision and penalized when making a wrong one. RL differs from supervised learning, where the training data brings the answer key with it and a model is then trained with the correct answer itself. In RL, the agents decide what to do to perform the given task and, if no training dataset is available...




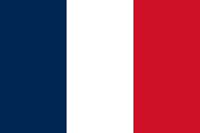


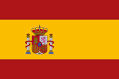



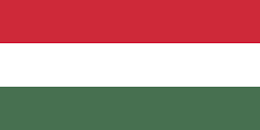

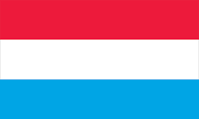





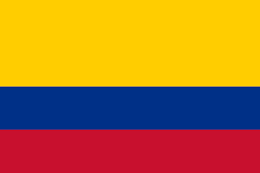
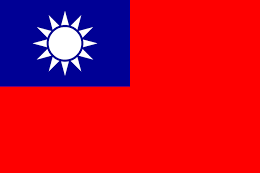
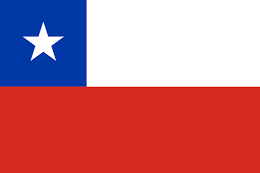



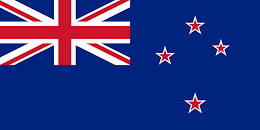
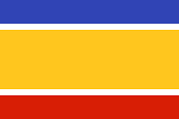
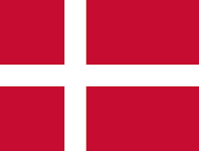
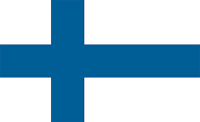







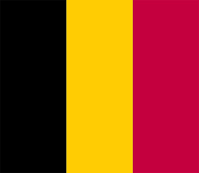

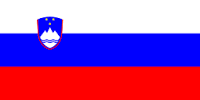





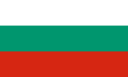


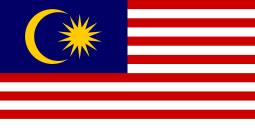



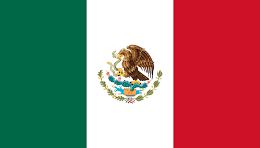
