In Chapter 8, Reinforcement Learning, we learned about how to use policy optimization methods for continuous action spaces. Policy optimization methods learn directly by optimizing a policy from actions taken in their environment, as explained in the following diagram:
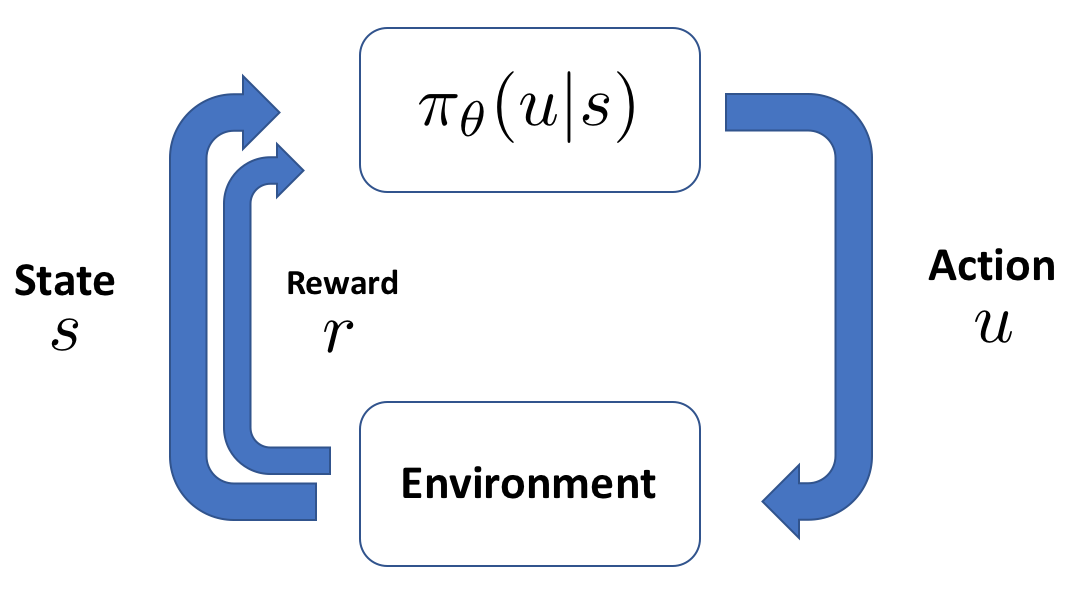
Remember, policy gradient methods are off-policy, meaning that their behavior in a certain moment is not necessarily reflective of the policy they are abiding by. These policy gradient algorithms utilize policy iteration, where they evaluate the given policy and follow the policy gradient in order to learn an optimal policy.
Before we get started, let's quickly review the Markov process that is in reinforcement learning algorithms. The entity (our algorithm) that navigates a Markov Decision process is called an agent. In this case, the agent would be the...