Naive Bayes classification
In the past example, we have seen with single word called lottery, however, in this case we will be discussing with a few more additional words such as Million and Unsubscribe to show how actual classifiers do work. Let us construct the likelihood table for the appearance of the three words (W1, W2, and W3), as shown in the following table for 100 emails:
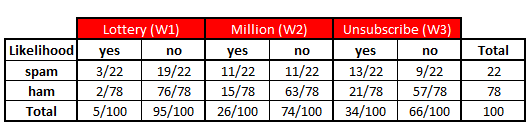
When a new message is received, the posterior probability will be calculated to determine that email message is spam or ham. Let us assume that we have an email with terms Lottery and Unsubscribe, but it does not have word Million in it, with this details, what is the probability of spam?
By using Bayes theorem, we can define the problem as Lottery = Yes, Million = No and Unsubscribe = Yes:

Solving the preceding equations will have high computational complexity due to the dependency of words with each other. As more number of words are added, this will even explode and also huge memory will be needed for processing...