As we discussed in the Value function approximation section, we are training the network online, as the agent receives stream of experiences from the environment. But the environment is usually sequential, and consecutive experiences might not differ much. For example, imagine that the agent is a car, which is currently sliding downhill. While doing so, it receives consistent feedback that the speed increases. If we feed the network with such unified training data, there is a chance that it will start dominating all other experiences. The network might "forget" previous situations and the performance would decrease (this is a disadvantage of some neural networks). We can solve this issue with experience replay. As the environment interaction goes, we'll store a sliding window of the latest n interactions: (state st-1, action at-1, reward rt, state...




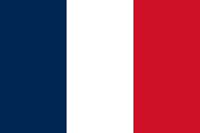


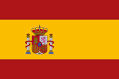



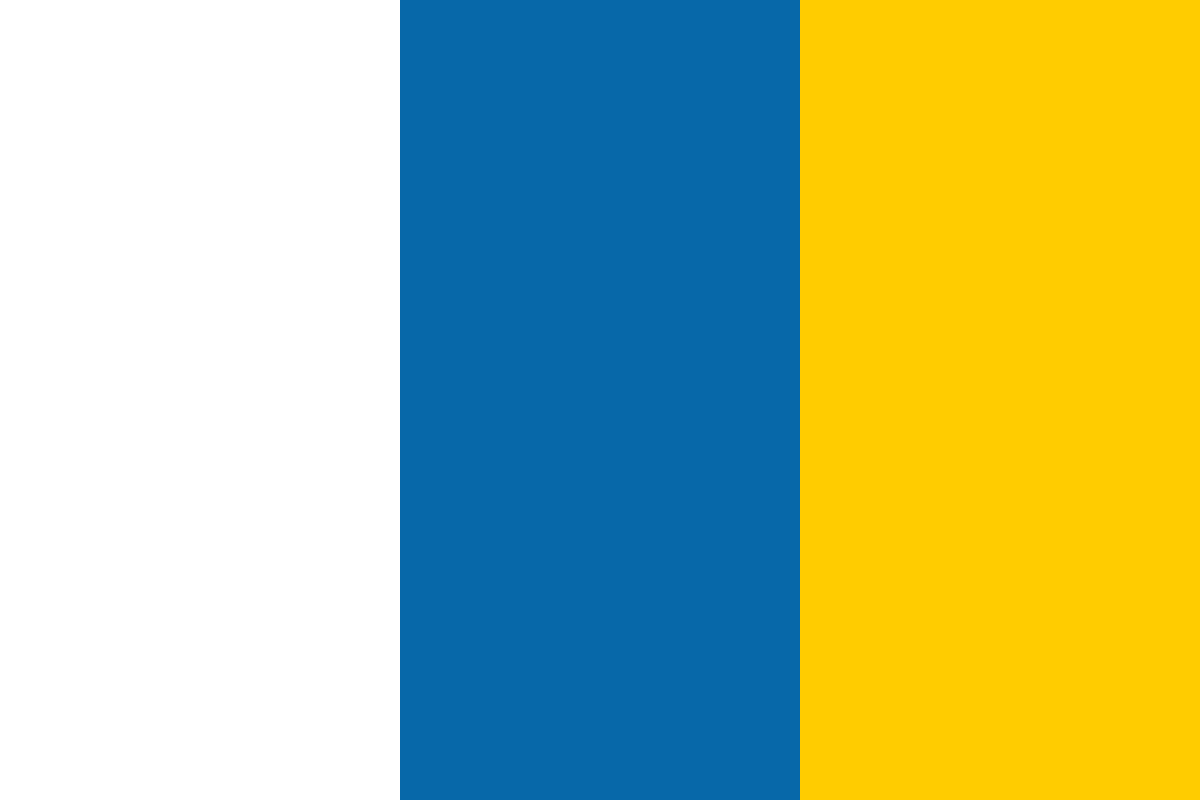
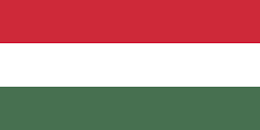

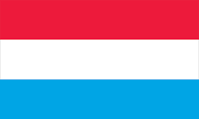





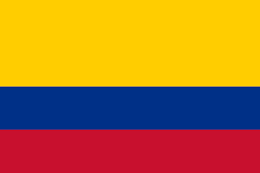
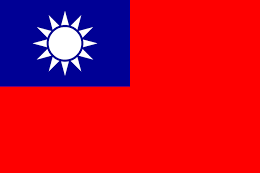
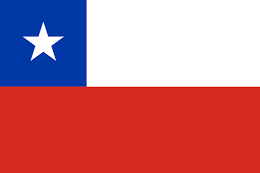



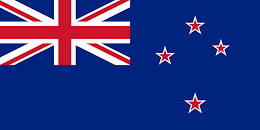
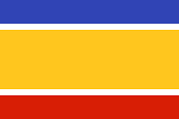
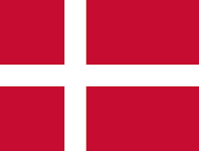
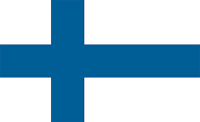







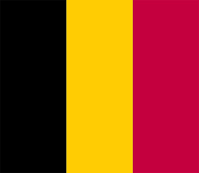

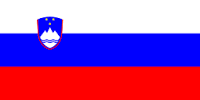





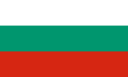


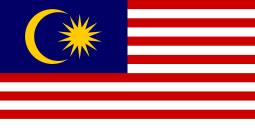



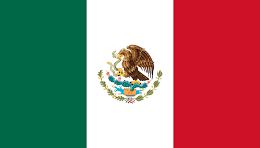
