Often when data mining, an analyst is happy to get their hands on a new feature column because the hope is that this added feature will bring additional new information. While this expectation fits with human intuition, there is an enormous caveat that must be understood and respected. This caveat is a result of what's known as the curse of dimensionality, which was coined in the 1950s by the mathematician, Richard E. Bellman. In short, a statistically-significant representation of chunks of feature space requires exponentially more and more examples (that is, rows) as the number of dimensions (that is, features) grows. Failure to grow the number of examples with the number of dimensions causes the dataset to become sparse and no longer representative of ground truth. The common rule of thumb is that you should have five examples for every one dimension...




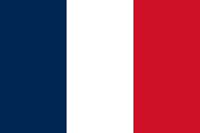


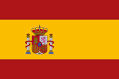



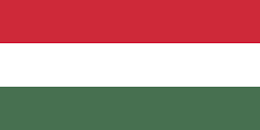

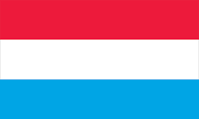





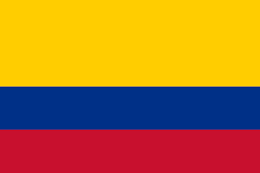
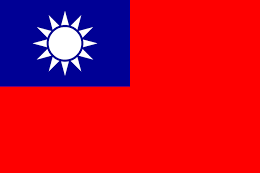
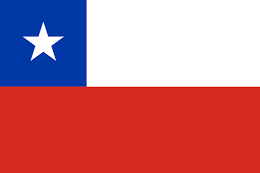



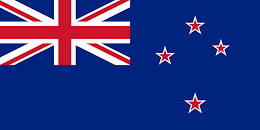
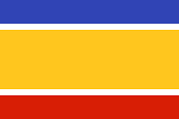
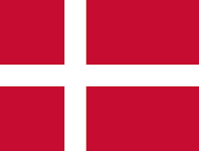
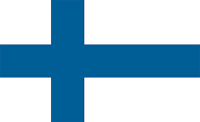







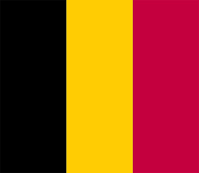

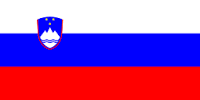





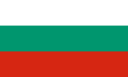


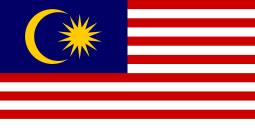



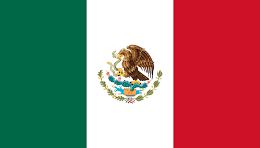
