Introducing Cascading
Cascading is an abstraction that empowers us to write efficient MapReduce applications. The API provides a framework for developers who want to think in higher levels and follow Behavior Driven Development (BDD) and Test Driven Development (TDD) to provide more value and quality to the business.
Cascading is a mature library that was released as an open source project in early 2008. It is a paradigm shift and introduces new notions that are easier to understand and work with.
In Cascading, we define reusable pipes where operations on data are performed. Pipes connect with other pipes to create a pipeline. At each end of a pipeline, a tap is used. Two types of taps exist: source, where input data comes from and sink, where the data gets stored.
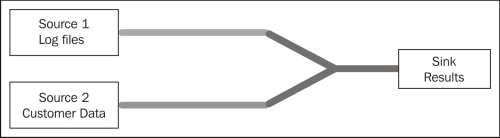
In the preceding image, three pipes are connected to a pipeline, and two input sources and one output sink complete the flow. A complete pipeline is called a flow, and multiple flows bind together to form a cascade. In the following diagram, three flows form a cascade:
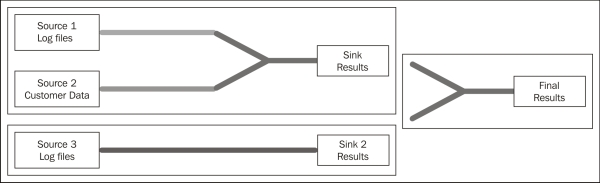
The Cascading framework translates the pipes, flows, and cascades into sets of map and reduce phases. The flow and cascade planner ensure that no flow or cascade is executed until all its dependencies are satisfied.
The preceding abstraction makes it easy to use a whiteboard to design and discuss data processing logic. We can now work on a productive higher level abstraction and build complex applications for ad targeting, logfile analysis, bioinformatics, machine learning, predictive analytics, web content mining, and for extract, transform and load (ETL) jobs.
By abstracting from the complexity of key-value pairs and map and reduce phases of MapReduce, Cascading provides an API that so many other technologies are built on.
What happens inside a pipe
Inside a pipe, data flows in small containers called tuples. A tuple is like a fixed size ordered list of elements and is a base element in Cascading. Unlike an array or list, a tuple can hold objects with different types.
Tuples stream within pipes. Each specific stream is associated with a schema. The schema evolves over time, as at one point in a pipe, a tuple of size one can receive an operation and transform into a tuple of size three.
To illustrate this concept, we will use a JSON transformation job. Each line is originally stored in tuples of size one with a schema: 'jsonLine
. An operation transforms these tuples into new tuples of size three: 'time
, 'user
, and 'action
. Finally, we extract the epoch, and then the pipe contains tuples of size four: 'epoch
, 'time
, 'user
, and 'action
.
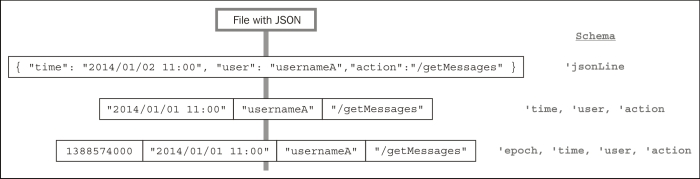
Pipe assemblies
Transformation of tuple streams occurs by applying one of the five types of operations, also called pipe assemblies:
- Each: To apply a function or a filter to each tuple
- GroupBy: To create a group of tuples by defining which element to use and to merge pipes that contain tuples with similar schemas
- Every: To perform aggregations (count, sum) and buffer operations to every group of tuples
- CoGroup: To apply SQL type joins, for example, Inner, Outer, Left, or Right joins
- SubAssembly: To chain multiple pipe assemblies into a pipe
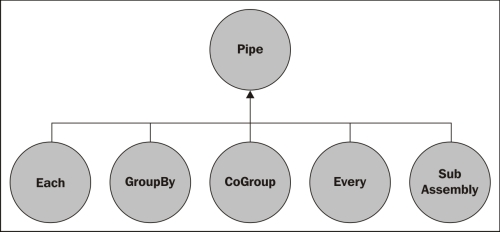
To implement the pipe for the logfile example with the INFO, WARNING, and ERROR levels, three assemblies are required: The Each assembly generates a tuple with two elements (level/message), the GroupBy assembly is used in the level, and then the Every assembly is applied to perform the count aggregation.
We also need a source tap to read from a file and a sink tap to store the results in another file. Implementing this in Cascading requires 20 lines of code; in Scala/Scalding, the boilerplate is reduced to just the following:
TextLine(inputFile) .mapTo('line->'level,'message) { line:String => tokenize(line) } .groupBy('level) { _.size } .write(Tsv(outputFile))
Cascading is the framework that provides the notions and abstractions of tuple streams and pipe assemblies. Scalding is a domain-specific language (DSL) that specializes in the particular domain of pipeline execution and further minimizes the amount of code that needs to be typed.
Cascading extensions
Cascading offers multiple extensions that can be used as taps to either read from or write data to, such as SQL, NoSQL, and several other distributed technologies that fit nicely with the MapReduce paradigm.
A data processing application, for example, can use taps to collect data from a SQL database and some more from the Hadoop file system. Then, process the data, use a NoSQL database, and complete a machine learning stage. Finally, it can store some resulting data into another SQL database and update a mem-cache application.
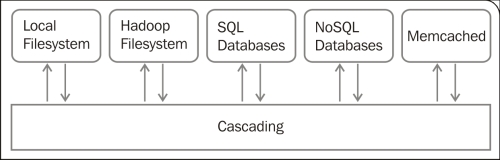