In this chapter, the goal was to discuss how important the element of time is in the field of machine learning and analytics, to identify the common traps when analyzing the time series, and to demonstrate the techniques and methods to work around these traps. We explored both the univariate and bivariate time series analysis for global temperature anomalies and human carbon dioxide emissions. Additionally, we looked at Granger causality to determine whether we can say, statistically speaking, that atmospheric CO2 levels cause surface temperature anomalies. We discovered that the p-values are higher than 0.05 but less than 0.10 for Granger causality from CO2 to temperature. It does show that Granger causality is an effective tool in investigating causality in machine learning problems. In the next chapter, we'll shift gears and take a look at how to apply learning...




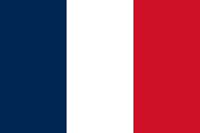


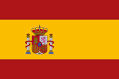



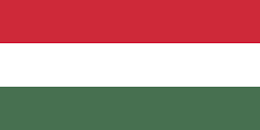

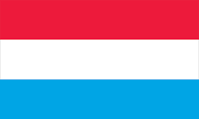





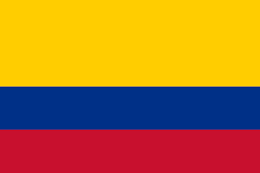
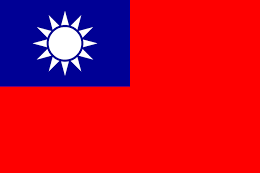
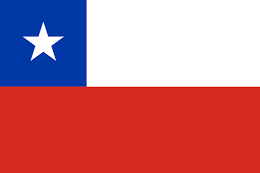



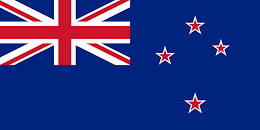
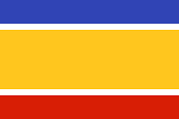
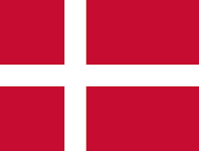
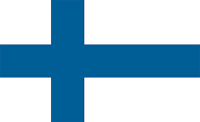







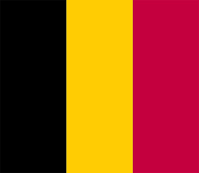

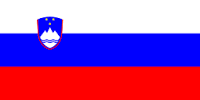





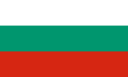


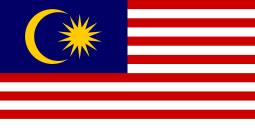



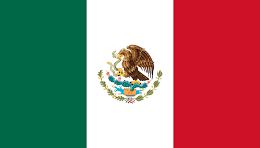
