In this chapter, we explored different forms of model-based architectures for one-shot learning. The most common thing we observed is the use of external memory, and how this can be helpful in learning representations at different stages of a neural network. NMT methods perform well on one-shot learning tasks, but they still have limited capacity because of hand-engineered memory-addressing functions, as they have to be differentiable. It might be interesting to explore more complex functions to address the memory. In meta networks, we saw how one new network was defined to enable fast learning of the original network, and how storing information about representations at the meta-learner level was useful to fine-tune parameters at the base level. Although model-based architectures are a good method of implementing one-shot learning, they come with the prerequisite of external...




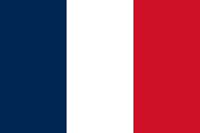


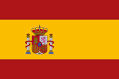




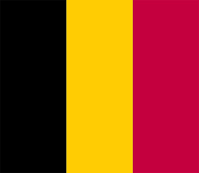
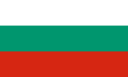
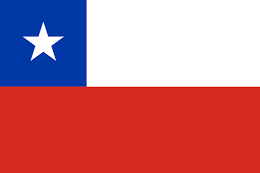
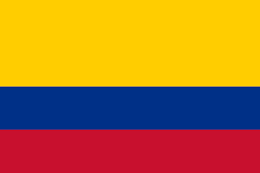
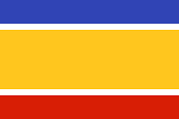

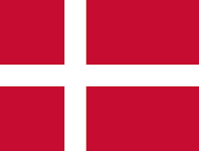



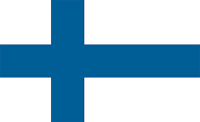

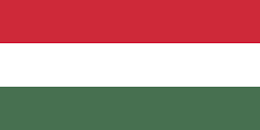






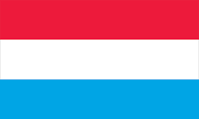
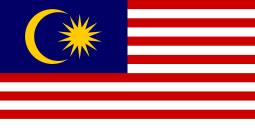

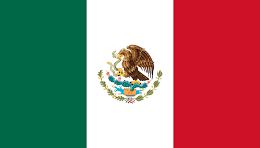

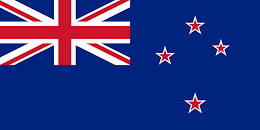







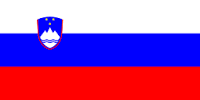




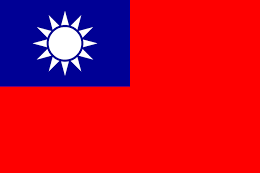


