Machine learning can be defined as the process from which an algorithm learns from data in order to be able to extract information that is useful for some business or research interest.
Even though all data science projects are different, a certain number of common steps can still be identified:
- Problem definition
- Data collection and cleaning
- Feature engineering
- Model building and evaluation
- Deployment
Even if these steps follow a logical order, the process is never linear and consists of back and forth operations between these different steps. It can be useful to go back to the problem definition after the data collection phase, for example, as well as returning to the feature engineering and model evaluation phases as many times as required to reach the desired outcomes. The following diagram illustrates this idea of moving back and forth between the different steps of a project:
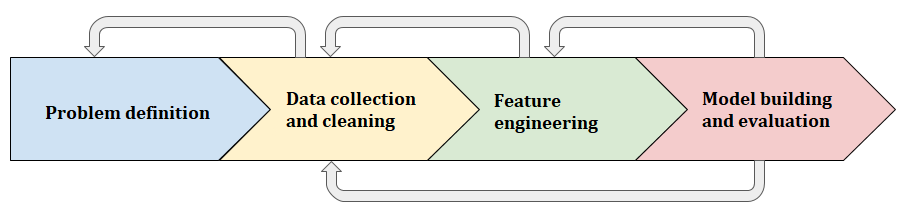
This project structure also applies when analyzing graph data, which...