Auto-encoders are a form of dimensionality reduction technique. When they are used in this manner, they mathematically and conceptually have similarities to other dimensionality reduction techniques such as PCA. Auto-encoders consist of two parts: an encoder which creates a representation of the data, and a decoder which tries to reproduce or predict the inputs. Thus, the hidden layers and neurons are not maps between an input and some other outcome, but are self (auto)-encoding. Given sufficient complexity, auto-encoders can simply learn the identity function, and the hidden neurons will exactly mirror the raw data, resulting in no meaningful benefit. Similarly, in PCA, using all the principal components also provides no benefit. Therefore, the best auto-encoder is not necessarily the most accurate one, but one that reveals some meaningful structure...




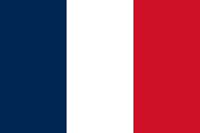


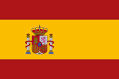



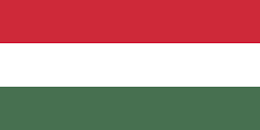

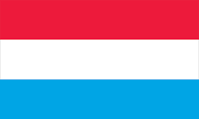





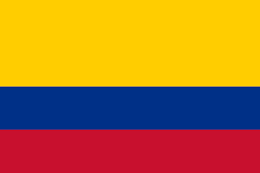
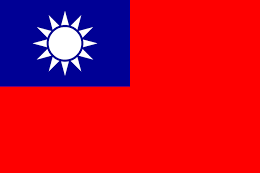
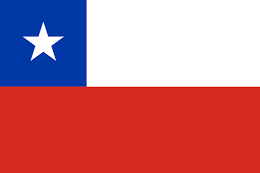



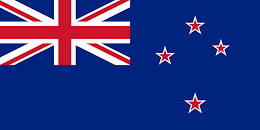
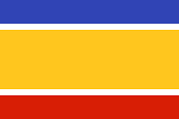
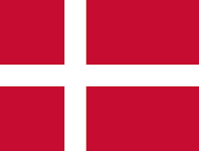
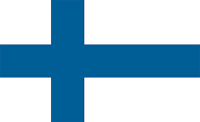







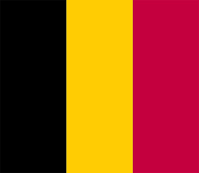

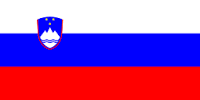





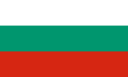


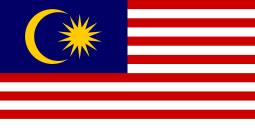



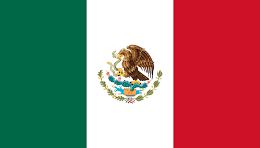
