Data preparation
By examining Figure 12.7, you can see that many of the columns in our dataset are of the data type FLOAT
. While FLOAT
is a legacy SQL data type, the GoogleSQL modern datatype is FLOAT64
. FLOAT64
provides higher precision than FLOAT
as FLOAT64
uses 64 bits to represent floating-point numbers, while FLOAT
uses 32 bits.
For this hands-on example, we will leave most of the FLOAT
and other data types and only modify the TIME
column. We will be able to gain the insights we need from our dataset by leaving most of the data types as they are.
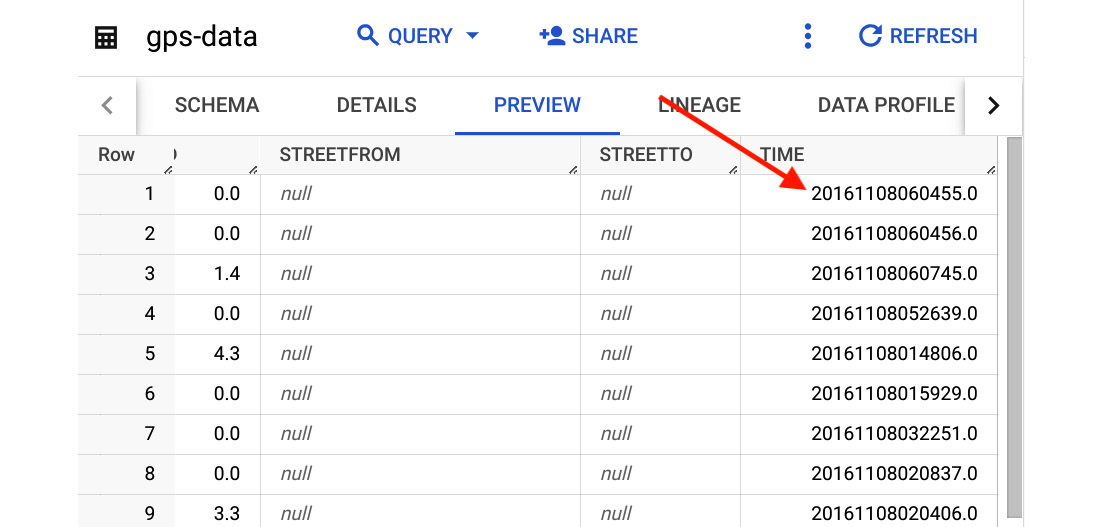
Figure 12.8 – Previewing our GPS data set to examine the TIME column
Note in Figure 12.7 that the TIME column is FLOAT
, has a decimal point, and is not very readable. Upon loading, the TIME column is formatted YYYYMMDDHHMMSS (14 digits). To prepare our dataset, we will convert this column into YYYY-MM-DD format (8 digits).
To convert the TIME column, we will use BigQuery Data Definition Language...