Data-centric versus model-centric ML
So far, we have established that data centricity is about systematically engineering the data used to build ML models. The conventional and more prevalent model-centric approach to ML suggests that optimizing the model itself is the key to better performance.
As illustrated in Figure 1.3, the central objective of a model-centric approach is improving the code underlying the model. Under a data-centric approach, the goal is to find a much larger upside in improved data quality:
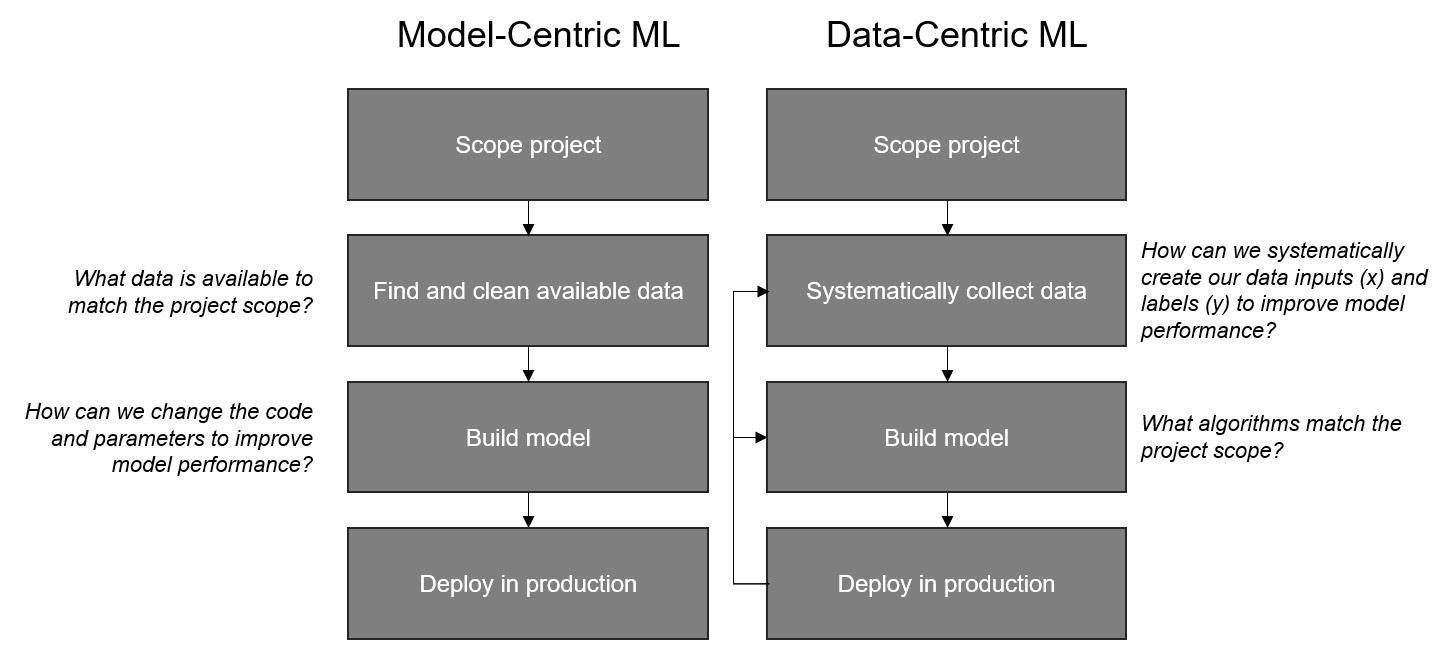
Figure 1.3 – Building ML solutions via model-centric and data-centric workflows
ML model development has traditionally focused on improving model performance mainly by optimizing the code. Under a data-centric approach, the focus shifts to achieving even larger performance enhancements, mainly by iteratively improving data quality. It is important to note that the data-centric approach sits on top of the principles and techniques that...