Serverless architectures generally are leveraged as real-time systems as they work as a function as service which is triggered by a set of available triggers. However, this is a very common misconception, as serverless systems can be leveraged equally well both as real-time and batch architectures. Knowing how to leverage the concept of serverless systems as batch architectures will open up many engineering possibilities, as all engineering teams don't necessarily need or have real-time systems to operate.
Serverless systems can be batched by leveraging the following:
- The cron facility in triggers
- The concept of queues
Firstly, let's understand the concept of the cron facility in triggers. Serverless systems on the cloud have the ability to set up monitoring, which enables the trigger to get triggered every few minutes or hours and can be set as a normal cron job. This helps in leveraging the concept of serverless as a regular cron batch job. In the AWS environment, Lambda can be triggered as a cron via AWS CloudWatch, by setting the frequency of the cron by manually entering the time interval as the input and also by entering the interval in the cron format:
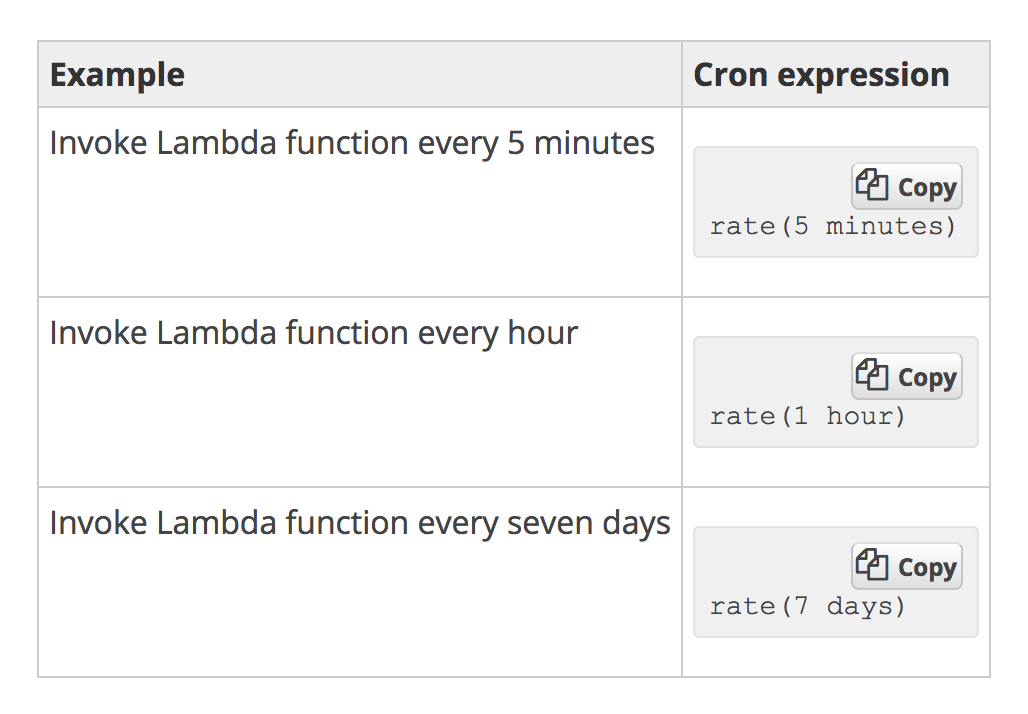
One can also leverage the concept of queues when building serverless batch architectures. Let's understand this by setting an example data pipeline. Let's say the system which we intend to build does the following tasks:
- A user or a service sends some data into a database or a much simpler data store, such as AWS's S3.
- Once there are more than 100 files in my data store, we'll want to do some task. Let's say, doing some analytics on them, for example, such as counting the pages.
This can be achieved via queues, and this is one of the simpler serverless systems we can consider as an example. So, this can be achieved as follows:
- The user or the service uploads or sends the data to the data store which we have selected.
- A queue is configured for the purpose of this task.
- An event can be configured to S3 buckets or data stores so that as soon as data enters into the store, a message is sent to the queue which we have configured earlier.
- Monitoring systems can be set to monitor the queue for the number of messages in it. It is advisable to use the monitoring system of the cloud provider you are using so that the system stays completely serverless.
- Alarms can be set to the monitoring systems, configuring a threshold for these alarms. For example, the alarm needs to be triggered whenever the number of messages in our queue reaches or exceeds 100.
- This alarm can act as a trigger to the Lambda function which does the analytics by first receiving messages from the queue and then querying the data store using the filename received from the message.
- Once the analytics are completed on the files, the processed files can be pushed to another data store for storage.
- After the entire task is completed, the container or the server where the Lambda function has run will be terminated, thus making this pipeline completely serverless.