AdaBoost classifier
Boosting is another state-of-the art model that is being used by many data scientists to win so many competitions. In this section, we will be covering the AdaBoost algorithm, followed by gradient boost and extreme gradient boost (XGBoost). Boosting is a general approach that can be applied to many statistical models. However, in this book, we will be discussing the application of boosting in the context of decision trees. In bagging, we have taken multiple samples from the training data and then combined the results of individual trees to create a single predictive model; this method runs in parallel, as each bootstrap sample does not depend on others. Boosting works in a sequential manner and does not involve bootstrap sampling; instead, each tree is fitted on a modified version of an original dataset and finally added up to create a strong classifier:
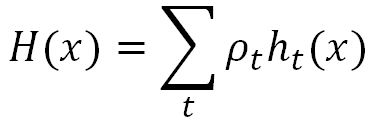
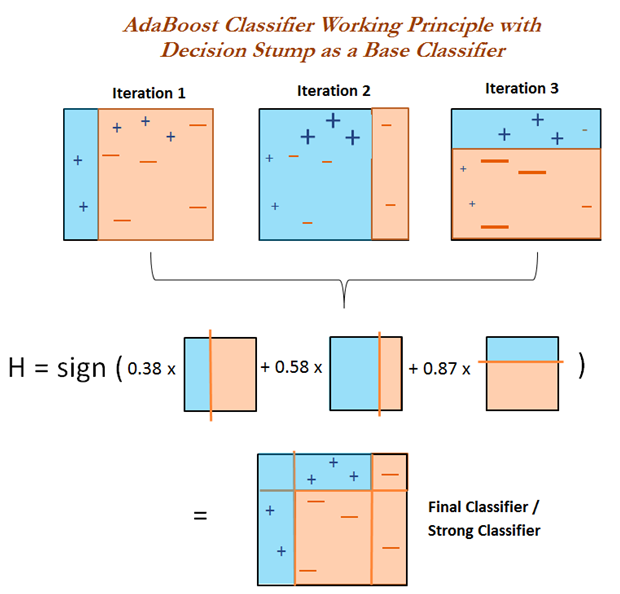
The preceding figure is the sample methodology on how AdaBoost works. We will cover step-by-step procedures in detail...