Tuning an algorithm is simply a process that one goes through in order to enable the algorithm to perform optimally in terms of runtime and memory usage. In Bayesian statistics, a hyperparameter is a parameter of a prior distribution. In terms of ML, the term hyperparameter refers to those parameters that cannot be directly learned from the regular training process.
Hyperparameters are usually fixed before the actual training process begins. This is done by setting different values for those hyperparameters, training different models, and deciding which ones work best by testing them. Here are some typical examples of such parameters:
- Number of leaves, bins, or depth of a tree
- Number of iterations
- Number of latent factors in a matrix factorization
- Learning rate
- Number of hidden layers in a deep neural network
- The number of clusters in k-means clustering and so on
In short, hyperparameter tuning is a technique for choosing the right combination of hyperparameters based on the performance of presented data. It is one of the fundamental requirements for obtaining meaningful and accurate results from ML algorithms in practice. The following figure shows the model tuning process, things to consider, and workflow:
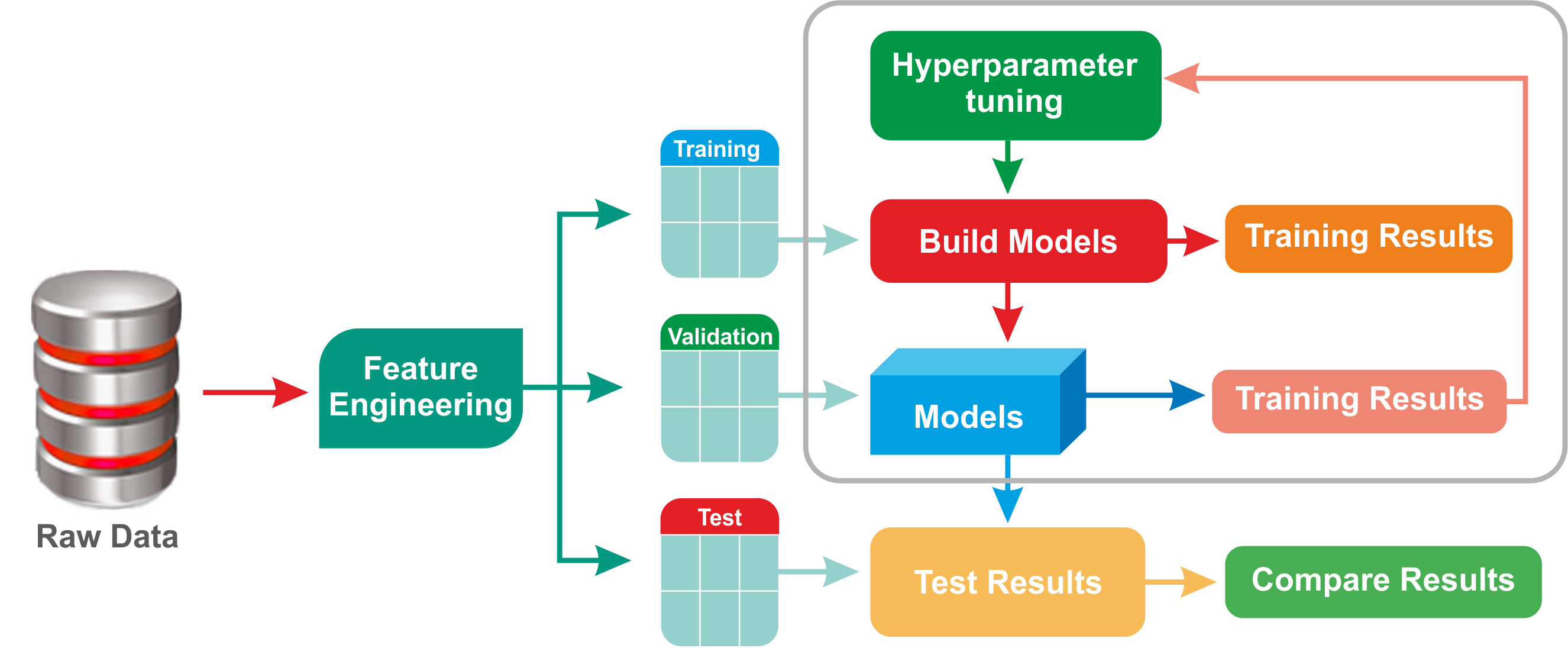
Cross-validation (also known as rotation estimation) is a model validation technique for assessing the quality of the statistical analysis and results. The target is to make the model generalized toward an independent test set. It will help if you want to estimate how a predictive model will perform accurately in practice when you deploy it as an ML application. During the cross-validation process, a model is usually trained with a dataset of a known type.
Conversely, it is tested using a dataset of an unknown type. In this regard, cross-validation helps to describe a dataset to test the model in the training phase using the validation set. There are two types of cross-validation that can be typed as follows:
- Exhaustive cross-validation: This includes leave-p-out cross-validation and leave-one-out cross-validation
- Non-exhaustive cross-validation: This includes K-fold cross-validation and repeated random subsampling cross-validation
In most cases, the researcher/data scientist/data engineer uses 10-fold cross-validation instead of testing on a validation set (see more in Figure 6, 10-fold cross-validation technique). This is the most widely used cross-validation technique across all use cases and problem types, as explained by the following figure.
Basically, using this technique, your complete training data is split into a number of folds. This parameter can be specified. Then the whole pipeline is run once for every fold and one ML model is trained for each fold. Finally, the different ML models obtained are joined by a voting scheme for classifiers or by averaging for regression:
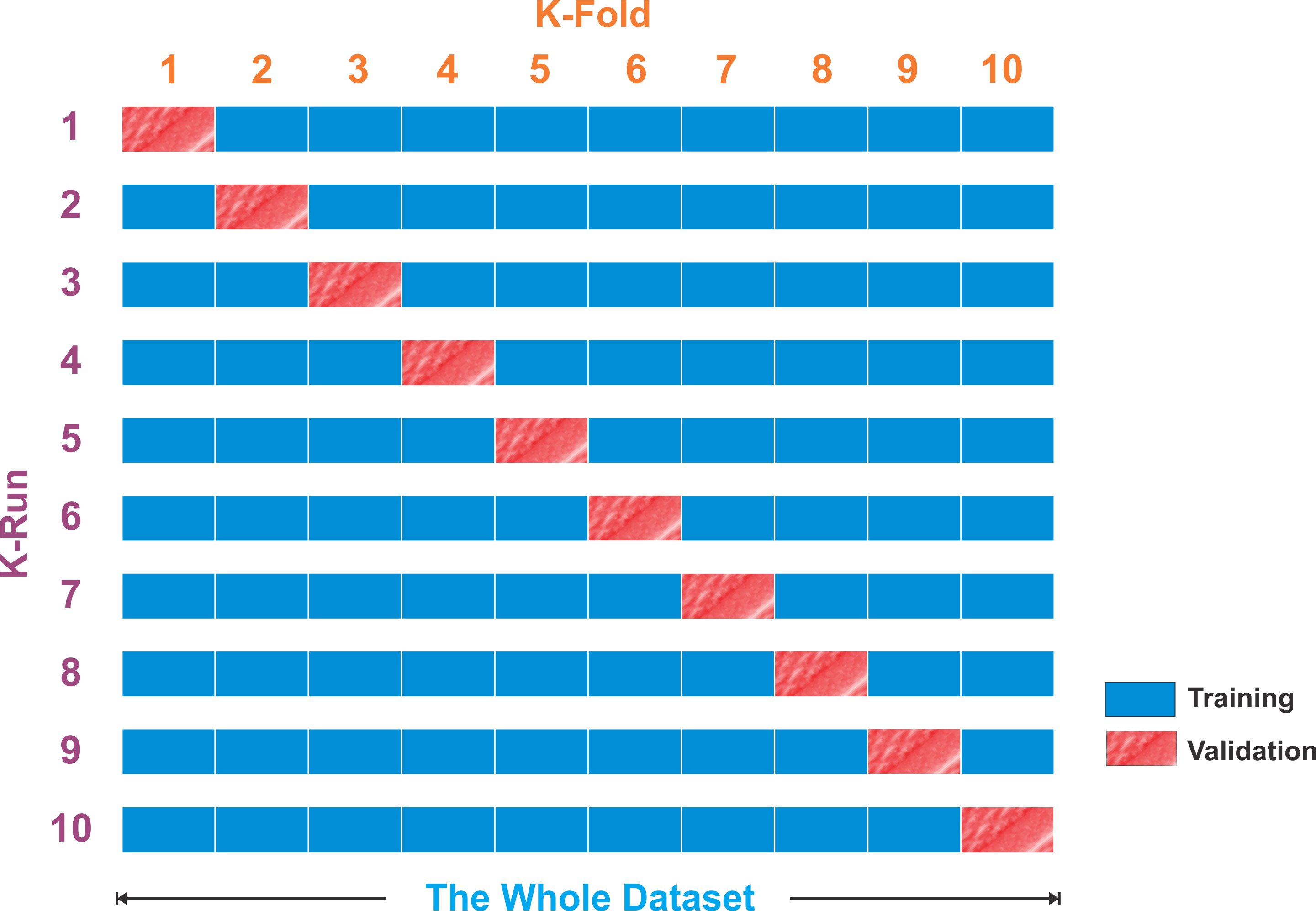
Moreover, to reduce the variability, multiple iterations of cross-validation are performed using different partitions; finally, the validation results are averaged over the rounds.