Leveraging the wisdom of the crowds with stacked ensembles
Stacking (stacked generalization) refers to a technique of creating ensembles of potentially heterogeneous machine learning models. The architecture of a stacking ensemble comprises at least two base models (known as level 0 models) and a meta-model (the level 1 model) that combines the predictions of the base models. The following figure illustrates an example with two base models.
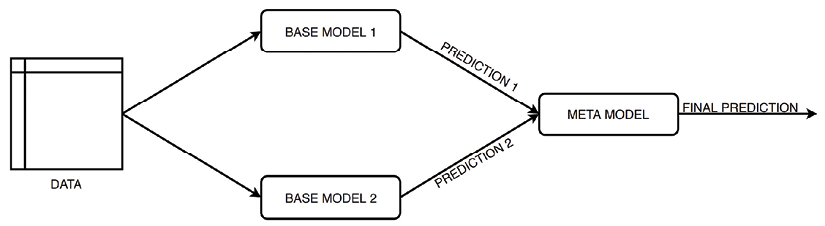
Figure 14.15: High-level schema of a stacking ensemble with two base learners
The goal of stacking is to combine the capabilities of a range of well-performing models and obtain predictions that result in a potentially better performance than any single model in the ensemble. That is possible as the stacked ensemble tries to leverage the different strengths of the base models. Because of that, the base models should often be complex and diverse. For example, we could use linear models, decision trees, various kinds of ensembles, k...