In order to build a successful statistical or machine learning model, we need to follow a simple (but hard!) rule: make it as simple as possible (so it generalizes the phenomenon being modeled well) but not too simple (so it loses its main ability to predict). A visual example of how this manifests is as follows (from http://bit.ly/2GpRybB):
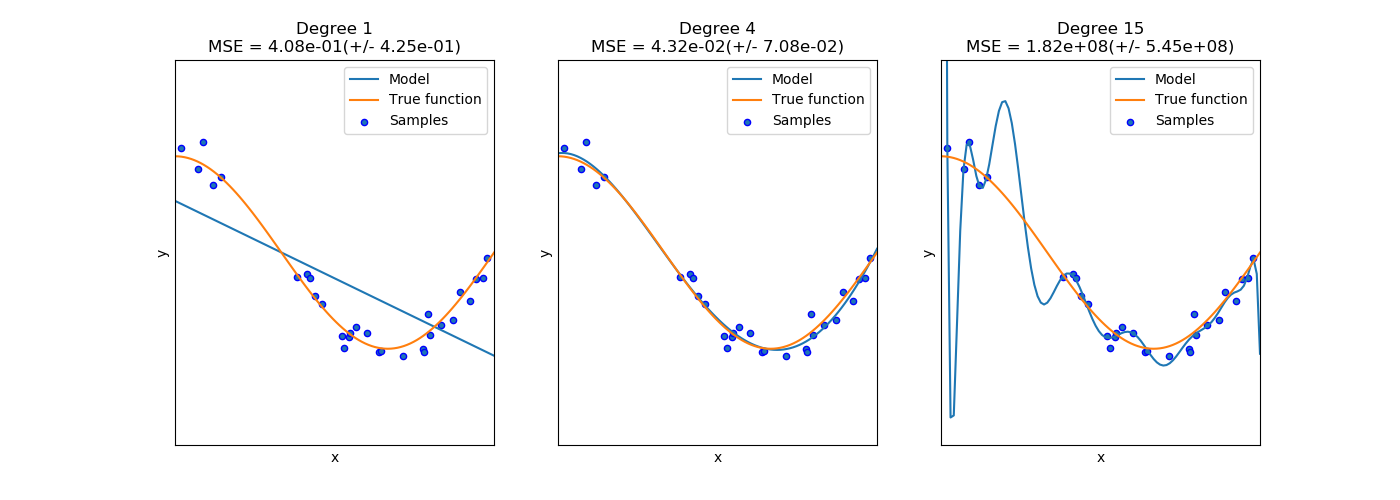
The middle chart shows a good fit: the model line follows the true function well. The model line on the left chart oversimplifies the phenomenon and has literally no predictive power (apart from a handful of points)—a perfect example of underfitting. The model line on the right follows the training data almost perfectly but if new data was presented, it would most likely misrepresent it—a concept known as overfitting, that is, it does not generalize well. As you can see from these three charts, the complexity...