Very few algorithms produce optimized models at the first attempt. This is because the algorithms might need some parameter tuning from the data scientist to improve their accuracy or performance. For example, the learning rate for deep neural networks that we mentioned in Chapter 7, Implementing Deep Learning Algorithms, needs to be manually tuned. A low learning rate may lead the algorithm to take longer (and hence be more expensive if we're running it on the cloud), whereas a high learning rate might miss the optimal set of weights. Likewise, a tree with more levels may take more time to train, but could create a model with better predictive capabilities (although it could also cause the tree to overfit). These parameters that direct the learning of the algorithms are called hyperparameters, and contrary to the model parameters (for...





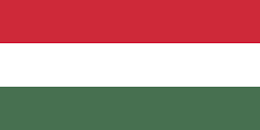

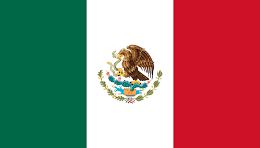


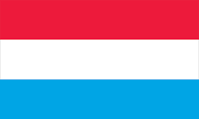



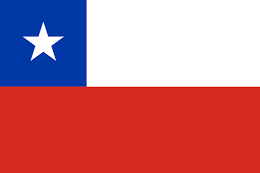



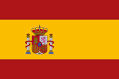


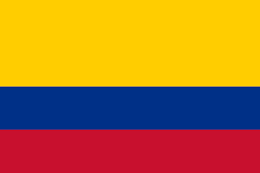
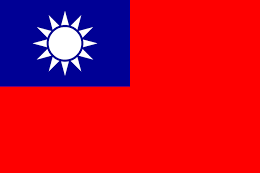


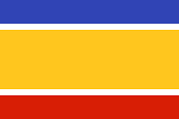
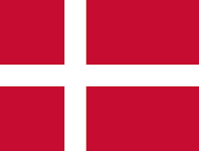
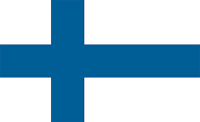



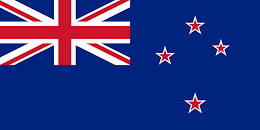


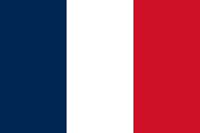



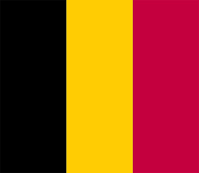

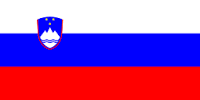




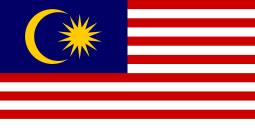


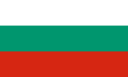



