More complex time-series objects
The main limitation of the
ts
time-series R object class (besides the aforementioned x axis issue) is that it cannot deal with irregular time-series. To overcome this problem, we have several alternatives in R.
The zoo
package and its reverse dependent
xts
packages are ts
-compatible classes with tons of extremely useful methods. For a quick example, let's build a zoo
object from our data, and see how it's represented by the default plot:
> library(zoo) > zd <- zoo(daily[, -1, with = FALSE], daily[[1]]) > plot(zd)
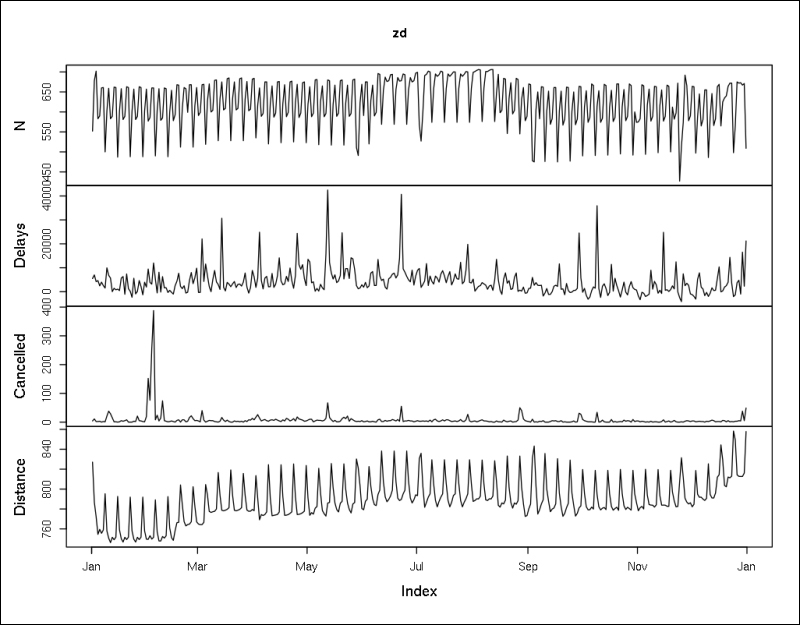
As we have defined the date
column to act as the timestamp of the observations, it's not shown here. The x axis has a nice human-friendly date annotation, which is really pleasant after having checked a bunch of integer-annotated plots in the previous pages.
Of course, zoo
supports most of the ts
methods, such as diff
, lag
or cumulative sums; these can be very useful for visualizing data velocity:
> plot(cumsum(zd))
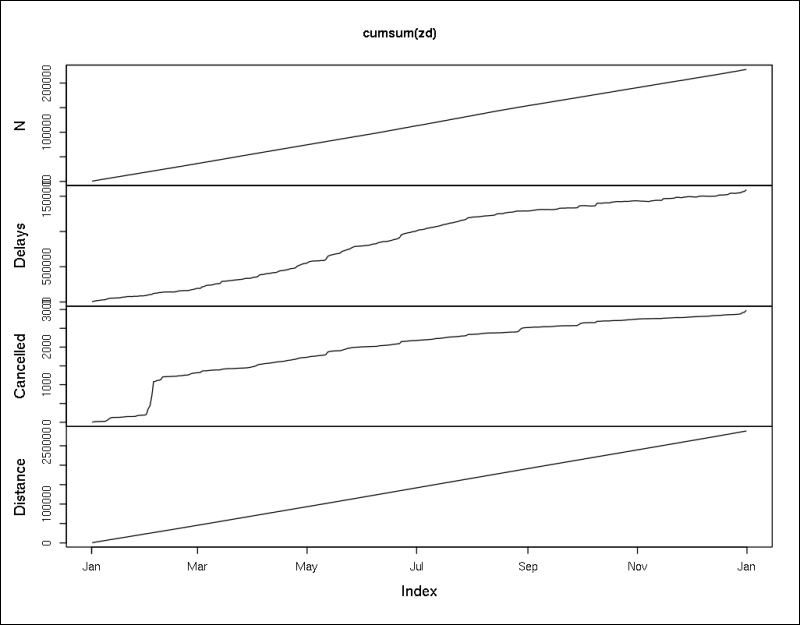
Here...