A comparison with other IaC
In the preceding section, you got to know about Terraform, and similar to Terraform, there are many other IaC options that are more specific to individual cloud providers, such as ARM templates for the Azure cloud, CloudFormation for AWS, and Cloud Deployment Manager for GCP. Likewise, each provider has come up with their own IaC that is used for their infrastructure provisioning. Now, the challenge for an administrator or developer is to learn and remember a vast number of different template syntaxes. To overcome this challenge, Terraform came up with a solution involving common workflows and syntax that allows operators to operate complex infrastructure. Let's try to understand how Terraform is different in terms of being cross-platform, as well as its modularity, language of code, validation, readability, maintainability, workflow, error management, state management, and so on for major cloud providers including AWS, Azure, and GCP.
CloudFormation versus Terraform
In this section, we will see how Terraform differs from CloudFormation based on various parameters:
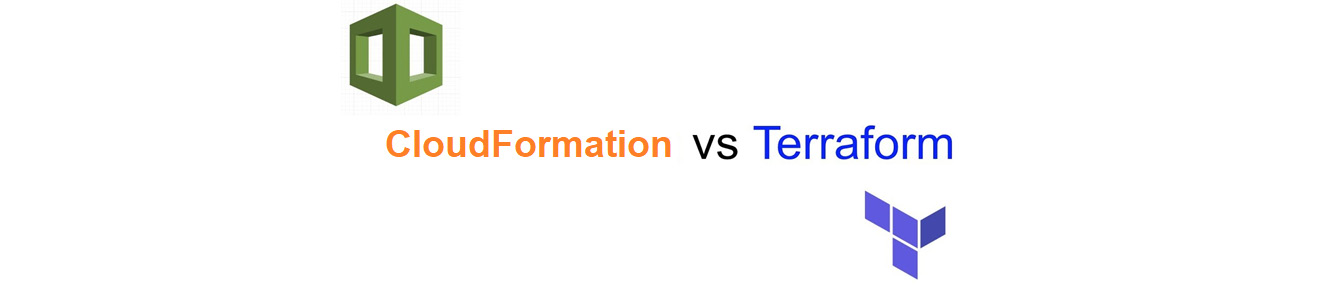
Figure 1.9 – CloudFormation versus Terraform
Let's get started.
Cross-platform
A major benefit of Terraform is that you can use it with a variety of cloud providers, including AWS, GCP, and Azure. CloudFormation templates are more centric to AWS. So here, Terraform would be more preferred than AWS CloudFormation.
Language
Terraform is written in HashiCorp Configuration Language (HCL). HCL's syntax is very powerful and allows you to reference variables, attributes, and so on, whereas CloudFormation uses either JSON or YAML, which are bare notation languages and are not as powerful as HCL. With CloudFormation, you can also reference parameters, such as other stacks, but overall, we think HCL makes Terraform more productive.
On the other hand, CloudFormation has good support for various conditional functionalities. Terraform has the count
, for_each
, and for
loops, which make certain things easy (such as creating N identical resources) and certain things a bit harder (such as the if-else
type conditional structure; for example, count = var.create_eip == true ? 1: 0
). CloudFormation has also a wait condition and creation policy, which can be important in certain deployment situations where you may have to wait before a certain condition is satisfied.
In terms of language, Terraform is simple and easy to draft, which would encourage developers and administrators to start exploring Terraform, but it practically depends upon the use case scenario, which might in some cases require you to use CloudFormation for AWS resource deployment and manageability.
Modularity
Modularity is generally defined as how much easier it is for you to implement your infrastructure code using Terraform or CloudFormation. Terraform's modules can be easily written and reused as infrastructure code in multiple projects by multiple teams. Hence, it is quite easy to modularize Terraform code. You have the option to modularize CloudFormation code using nested stacks. You cannot modularize your CloudFormation stack itself in the same way you can prepare stacks of stacks in Terraform. You can use cross-stack references in CloudFormation and in the same way, it can be referenced in Terraform using the terraform_remote_state
data source, which is used for deriving root module output from the other Terraform configuration.
Comparing both AWS CloudFormation and Terraform in terms of modularity, we can see that Terraform seems to be more friendly and easy to use.
Validation
Both tools allow you to validate infrastructure, but there is a difference. Using CloudFormation, you can validate AWS CloudFormation stacks, which means it will check only the syntax of your stack. If you want to update some resources, you can use CloudFormation change sets, which let you review the changes, meaning whether that specific resource defined in AWS CloudFormation will get replaced or updated, before you actually execute AWS CloudFormation stacks.
In the Terraform workflow, there is a plan
phase that provides complete information about what resources are getting modified, deleted, or created before you deploy the infrastructure code.
In AWS CloudFormation, you have CloudFormation Designer, which can help you to know all the resources you have defined in AWS CloudFormation. This will provide you with insight before you actually go ahead and perform the deployment.
In terms of validation, both Terraform and AWS CloudFormation have certain options to perform it. The only major difference that can be noticed is that in AWS CloudFormation, you would be required to use multiple AWS services whereas in Terraform, we are easily able to validate with just its workflow.
Readability
There is no doubt that when you write a Terraform configuration file you will find it easier compared to AWS CloudFormation. Terraform configuration files are written in HCL, meaning they are more presentable when you understand the syntax. When you think about CloudFormation, which is generally written in JSON or YAML, it is quite complex in terms of its readability, especially JSON; YAML is somewhat fine to read and understand but the problem with YAML is regarding its whitespace and linting. In Terraform also, there is the linting concern but Terraform has a smart way of performing linting by just running terraform fmt -recursive
, which will perform linting to all the Terraform configuration files present in the directory, whereas in YAML, you have to perform something manually or use some YAML validator tool that can help you with the validations.
So, in a nutshell, Terraform has more preferred than to AWS CloudFormation.
License and support
AWS CloudFormation is a managed service from AWS offered for free whereas Terraform is an open source infrastructure automation tool provided by HashiCorp. There is the Terraform Cloud Enterprise product from HashiCorp that is a licensed version and Enterprise customers need to purchase it from HashiCorp.
In terms of support plans, AWS has their own support plan that can be taken from AWS, in the same way that Terraform has their support option that can used by customers.
AWS console support
As AWS CloudFormation is a native AWS IaC tool, it naturally provides excellent support in the AWS console. In the AWS console, you can monitor the progress of deployments, see all deployment events, check various errors, integrate CloudFormation with CloudWatch, and so on. In the Terraform CLI, we don't have any console option, but in the Terraform Cloud Enterprise version, there is a console where you can see all the progress of the resource deployments.
Workflow
You must be thinking that AWS CloudFormation will be more mature from an operational perspective, and this is true to a certain extent, but here you need to understand the pain area. Let's take an example where you have a complex infrastructure of around 50 resources and you are creating it using AWS CloudFormation. After running for around 20-30 minutes, your AWS CloudFormation stack receives an error in one of the resources and because of that, the stack fails. In that case, it may roll back and destroy whatever it had created in AWS or sometimes, it may be able to deploy some of the resources even though it failed provided you have disabled rollback on failure in AWS CloudFormation. For further reading on the rollback on failure option in AWS CloudFormation, you can refer to https://aws.amazon.com/premiumsupport/knowledge-center/cloudformation-prevent-rollback-failure/. But to be very honest, if it did roll back and destroyed what it had created, then as an operator, you won't be looking for something like this because this adds more time and effort. Now, when you provision your AWS infrastructure using the Terraform workflow, this will provision all the required infrastructure in AWS. Even though, let's suppose, the Terraform workflow failed, then it will maintain the existing resources that had already been created prior to the failure. You can re-run the Terraform configuration, and this will simply provision the remaining resources rather than creating all the resources from scratch, which will save a significant amount of time and effort from the point of view of reworking.
So, based on the preceding paragraph, it appears that both AWS CloudFormation and Terraform workflows can be used. It's totally depends upon the consumer and which one they prefer.
Error message understandability
Errors thrown by Terraform can be tough for a novice to understand because sometimes they provide a strange error that is generated from the respective provider's APIs. In order to have an understanding of all different sorts of errors, you need to have an understanding of the arguments that are supported by the providers in Terraform.
So, comparing the understandability of error messages between Terraform and AWS CloudFormation, on occasion you may find that AWS CloudFormation is straightforward as you will have experience of working with the latter, and then, with your knowledge of Terraform, you will easily be able to figure out most of the errors.
Infrastructure state management
Both AWS CloudFormation and Terraform maintain the state of the infrastructure in their own way. In Terraform, state files get stored in the local disk or you can provide a remote backend location such as AWS S3 where you wish to store your Terraform state file. This state file would be written in JSON and would hold the information of the infrastructure that is defined in the Terraform configuration file. So, it is quite easy for Terraform to validate and check any sort of configuration drift.
In contrast, AWS CloudFormation is native to AWS, so we don't need to worry about how AWS CloudFormation would maintain its state. AWS CloudFormation used to get a signal from the resources that got provisioned or managed through AWS CloudFormation. If there is any configuration drift, then AWS CloudFormation may not be able to detect it.
Azure ARM templates versus Terraform
In this section, we will see how Terraform differs from ARM based on various parameters:
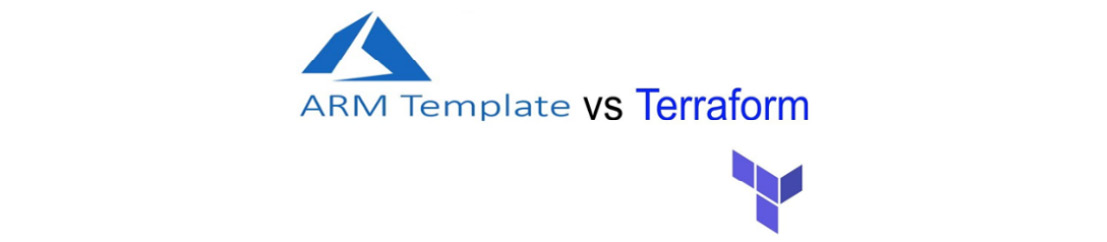
Figure 1.10 – ARM templates versus Terraform
Let's get started.
Cross-platform
The major benefit of Terraform is that you can use it with many cloud providers, including AWS, GCP, and Azure. The ARM template is more central to Azure whereas for Terraform, you just need to learn the HCL language syntax and Terraform workflow and start using any cloud provider, such as GCP, Azure, or AWS. So from this, we can understand that Terraform is cloud-agnostic and it would be easy for the user to implement infrastructure on any of these platforms using Terraform.
Language
Terraform is written in HCL. HCL's syntax is very powerful and allows you to reference variables, attributes, and so on, whereas ARM templates use JSON and is a little bit complex but very powerful, because you can use conditional statements, nested templates, and so on.
Keeping all of this in mind, here too, we would encourage end users and developers to use Terraform.
Modularity
Modularity generally means how much easier for you it is to implement your infrastructure code using Terraform or ARM templates. Terraform's modules can be easily written and reused as infrastructure code in multiple projects by multiple teams. Hence, it is quite easy to modularize the Terraform code.
You have the option to modularize ARM templates using a nested ARM template, although you also have the option of forming a nested template, the first one in line in your main template, and the second one using separate JSON files called from your main template.
Here, comparing both ARM templates and Terraform, Terraform scores more points than ARM templates, although in this case, ARM templates are still a viable option for end users if they are planning to create resources in Azure.
Validation
Both tools allow you to have validation of infrastructure, but there is a difference. Using the Azure CLI or PowerShell, you can validate a template by running az group deployment validate
, which means it will only check the syntax of your template. To validate what all the resources are that get provisioned, you have to deploy the complete ARM template.
Terraform provides a validation with the plan
phase that checks the current deployment in the Terraform state file, refreshes the status of the actual cloud configuration, and then calculates the delta between the current configuration and the target configuration.
In terms of validation, if you were to compare both, then here too, Terraform wins out over ARM templates.
Readability
There is no doubt that when you are intending to write a Terraform configuration file, you will find it more readable compared to ARM templates. Terraform configuration files written in HCL are more presentable when you understand their syntax, and the best part is that if you have learned the syntax, you would be able to write and use it anywhere in any cloud provider, including Azure, GCP, and AWS. When you consider ARM templates, which are generally written in JSON, they are quite complex in terms of their readability.
License and support
ARM templates use an Azure-native IaC approach and are available for free, whereas Terraform is an open source tool available from HashiCorp. There is the Terraform Cloud Enterprise version of Terraform that enterprise customers can purchase. Regarding support, both Azure and Terraform have their own support plans that can be utilized by the customer.
Azure portal support
As an ARM template is a native Azure IaC tool, it naturally provides excellent support in the Azure portal. You can monitor deployment progress in the Azure portal.
There is no Azure portal support for Terraform but if a customer is willing to use the Terraform Cloud Enterprise product, then they can utilize a portal that shows enough information about the infrastructure that they are planning to manage through Terraform.
Workflow
In terms of workflow, both ARM templates and Terraform have very good features. If the deployment of resources is interrupted halfway through, both Terraform and ARM templates have the capability to deploy the remaining resources during the second run, ignoring existing resources.
There is one minor difference, however. ARM template deployment requires an Azure resource group in place before you run the ARM template code, whereas Terraform configuration code is able to create an Azure resource group during the runtime itself, just as you would be required to define a resource group code block in the configuration file. Hence, it seems that both ARM templates and Terraform are quite satisfactory in terms of workflow.
Error message understandability
Terraform code can generate some weird errors. There is no doubt that in the backend, it would be using ARM provider APIs, which would show those errors, and it might be difficult for those end users who are new to Terraform to understand them, whereas in ARM templates, errors can be easily identified and fixed as end users may have experience of working with ARM templates.
So, in terms of comparing error message understandability between Terraform and ARM templates, this process may be easier in ARM templates.
Infrastructure state management
Both ARM templates and Terraform provide good state management. Terraform provides state file management using the backend state mechanism. In the state file, Terraform maps the resource that's been defined in the configuration file with what has actually been deployed so that it can easily trace any configuration drift. ARM is able to roll back to a previous successful deployment. You may have read that ARM is stateless as it does not store any state files.
Google Cloud Deployment Manager versus Terraform
In this section, we will see how Terraform differs from Google Cloud Deployment Manager based on various parameters:
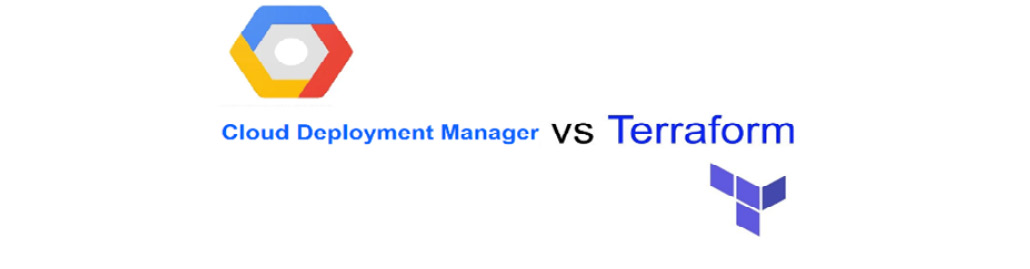
Figure 1.11 – Cloud Deployment Manager versus Terraform
Let's get started.
Cross-platform
As you have already seen a comparison of AWS and Azure, you should now have an understanding of Terraform, which can be used on any platform, including GCP too. You just need to know how you can write Terraform configuration files using HCL and their workflow.
Cloud Deployment Manager is an infrastructure deployment service that automates the creation and management of Google Cloud resources. So here, Terraform wins out over Cloud Deployment Manager.
Language
Terraform is written in HCL. HCL's syntax is very easy and powerful and it allows you to reference variables, attributes, and so on, whereas Cloud Deployment Manager uses YAML to create a configuration file and you can define multiple templates in the configuration file, which is written using Jinja or Python.
Keeping all of this in mind, here too we would encourage end users or developers to use Terraform because in order to use cloud deployment, you would be required to have command of Python or Jinja and YAML.
Modularity
Modularity means how easily you can define configuration files and reuse them for infrastructure provisioning and management using Terraform or Cloud Deployment Manager. Terraform's modules can be written and published easily, and these modules can easily be consumed by other product teams in their projects. So, modularizing Terraform code is quite straightforward.
You have the option to modularize Cloud Deployment Manager using a nested template inside a configuration file. These templates can be written in either Python or Jinja. A single configuration can import both Jinja and Python templates. These template files should be present locally or placed on a third-party URL so that Cloud Deployment Manager can access them.
Here, comparing both Cloud Deployment Manager and Terraform, Terraform scores more points than Google Cloud Deployment Manager, although Google Cloud Deployment Manager remains a viable option for end users here, too, if they are planning to create resources in Google.
Validation
Both tools allow you to validate infrastructure, but there is a difference. To figure out any syntax errors in the configuration file, you would be required to run it from Google Cloud Deployment Manager. This will throw any possible errors, as well as validating what resources get provisioned. In this case, you have to deploy a complete Google Cloud Deployment Manager configuration file in Google Cloud.
Terraform provides a validation with the plan phase that tells you what resources it is going to create, destroy, or update. It is used for comparison purposes with the existing Terraform state file and accordingly provides detailed insights to the administrator as to when they will run terraform plan
before being deployed to Google Cloud.
In terms of validation, if you were to compare both, then here, too, Terraform wins out over Google Cloud Deployment Manager.
Readability
If you are intending to write a Terraform configuration file, you will find it more readable compared to a Google Cloud Deployment Manager configuration file. Terraform configuration files written in HCL are more presentable, and the best part is that if you have learned Terraform syntaxes, you would be able to write and use them anywhere in any cloud, including Google, GCP, and AWS, or even on-premises.
When you think about Cloud Deployment Manager, which is generally written in YAML, it is also easy to understand, but when you need to introduce a template in the configuration file, which is written in Jinja or Python, then it becomes complex to read and understand.
Therefore, I am definitely of the opinion that Terraform is easier to use compared with Cloud Deployment Manager.
Maintainability
At a certain level, I think Terraform is more maintainable because of its superior modularity and how you can create different resources in Google Cloud and store its state file in Google Cloud Storage.
A Cloud Deployment Manager configuration file that is written in YAML and whose template is written in Python or Jinja is not that easy to read, which means the consumer needs to learn multiple coding languages. The nested template appears very complex and you need to store the template file in localhost or a third-party URL. Defining its template code locally in the configuration file and consuming it in the configuration file is not possible.
Cloud Deployment Manager doesn't have any kind of state file whereas Terraform has a state file that is stored in the remote backend.
The Terraform code configuration can be stored in any version control tool, such as Git, Azure Repos, or Bitbucket, if we are planning to use CI/CD DevOps pipelines.
Maintaining both Terraform code and Google Cloud Deployment Manager templates would be a little bit complex because both need to be stored either locally or in the source control tools.
Google console support
As Google Cloud Deployment Manager is a native Google IaC tool used for the provisioning and maintenance of infrastructure, it provides excellent support in the Google Cloud console. You can see the progress in terms of deployment in the Google Cloud console.
There is no Google Cloud console support for Terraform, though there is a separate portal if you are using the Terraform Cloud Enterprise version.
Workflow
In terms of workflow, both Cloud Deployment Manager and Terraform have very good features. If the deployment of resources is interrupted halfway through, both Terraform and Cloud Deployment Manager have the capability to deploy the remaining resources during the second run, ignoring existing resources.
There is one minor difference, however. Cloud Deployment Manager deployment requires a project in place before you run the configuration file using Cloud Deployment Manager, whereas Terraform configuration code is able to create a Google project during the runtime itself, just as you would be required to define a Google project resource block code in the configuration file. Therefore, I feel both of them are quite satisfactory when it comes to the workflow.
Error message understandability
In the previous AWS and Azure comparisons, we mentioned that errors used to emanate from the respective providers' APIs, and learning how to handle those errors comes with experience. If you were already equipped with that knowledge, then you would easily be able to rectify those issues. Again, compared to Google Cloud Deployment Manager, Terraform code exhibits a number of difficult errors that would be difficult for you to understand if you were new to Terraform. It doesn't mean that you can easily understand Google Cloud Deployment Manager errors simply because they involve multiple coding languages, such as YAML, JSON, or Jinja.
So, in terms of comparing the understandability of error messages between Terraform and Google Cloud Deployment Manager, if you had experience with Google Cloud, then it would be instinctive for you and this will help you to understand the errors easily. Regarding handling errors with Terraform, it may take some time for you to figure it out as Google Cloud resource APIs release errors specific to that.
Infrastructure state management
Both Cloud Deployment Manager and Terraform provide good state management. Terraform provides state file management using a backend state mechanism and state files can be stored in Google Cloud Storage. Cloud Deployment Manager is capable of rolling back to a previous successful deployment, since deployment used to happen incrementally by default, and it is quite easy to implement any changes you wish to make in terms of Google resources using Cloud Deployment Manager.
We covered how Terraform differs from other IaC options, mainly in terms of being cross-platform, as well as its modularity, readability, infrastructure state management, language, maintainability, workflow, cloud console support, and so on, and how it can be used by major cloud providers such as AWS, Azure, and GCP. All these comparisons will help you to understand how Terraform is a more powerful IaC and how you can effectively choose Terraform IaC for your infrastructure provisioning rather than selecting any specific IaC. Moving on, let's try to gain an understanding of Terraform architecture.