In real-world scenarios, the performance of deployed machine learning models degrades over time. To explain this in the case of fraud detection, the models may not capture evolving fraudulent behaviors. Because fraudsters adapt their methods and processes over time to game systems, it is important to retrain fraud detection engines on the latest and greatest data (reflecting anomalous behavior) available. Take a look at the following diagram:
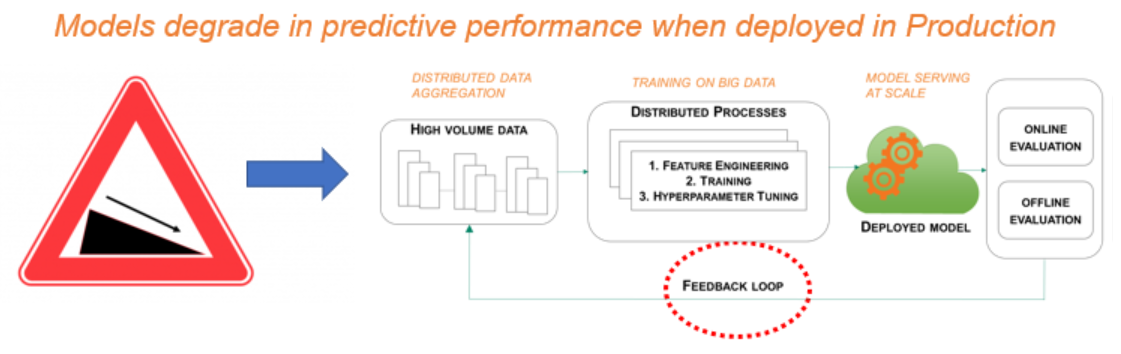
The preceding diagram shows how models degrade in terms of predictive performance when they are deployed in production. As another example, in the case of recommender systems, customer preferences keep changing based on a number of contextual and environmental factors. Therefore, it becomes important for personalization engines to capture such preferences and present the most relevant suggestions...