Traditional software development challenges
In the previous section, we saw the evolution in software development from the traditional waterfall model to Agile and DevOps practices. However, despite the success of these modern methods, we can't use the same methods for machine learning (ML) applications.
To see why, we have to look at what ML actually is; it's not just code, like in traditional software development, but code plus data. The data is fundamental to the ML model, and the code enables us to fit the data so we can derive insights from it:
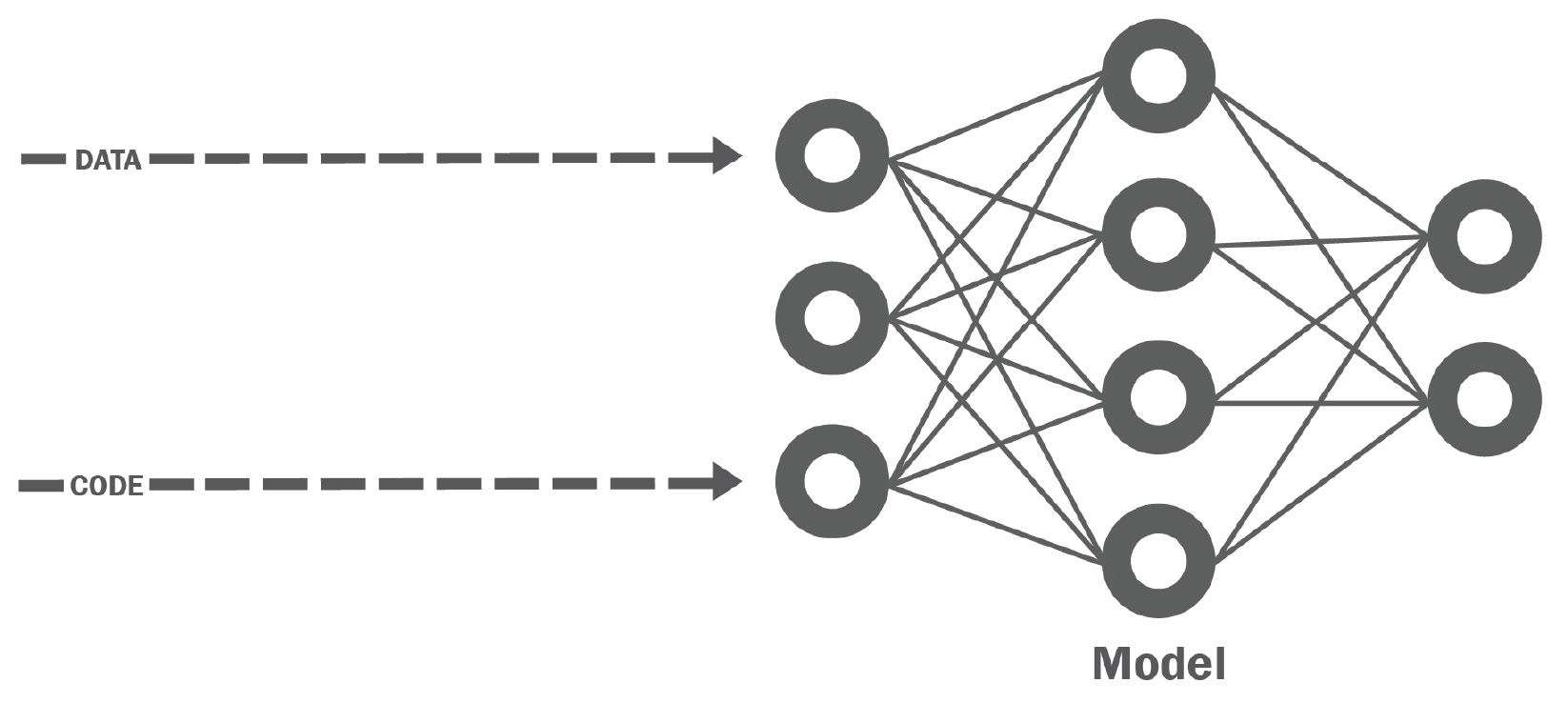
Figure 1.5 – ML = data + code
On account of this relationship between code and data, care must be taken to bridge the two together in development so they evolve in a controlled way, toward the common goal of a robust and scalable ML system; data for training, testing, and inference will change over time, across different sources, and needs to be met with changing code. Without a systematic MLOps approach, there can be divergence in how code and data evolve that causes problems in production, gets in the way of smooth deployment, and leads to results that are hard to trace or reproduce:
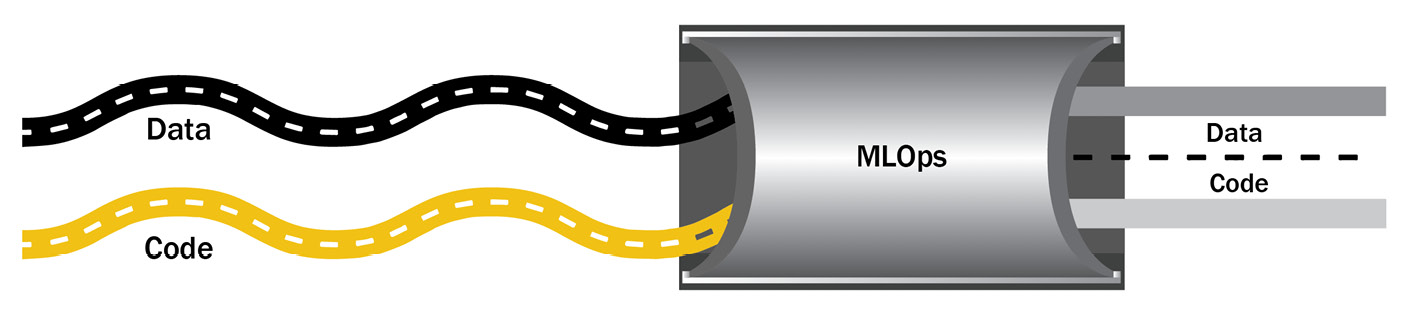
Figure 1.6 – MLOps – data and code progressing together
MLOps streamlines the development, deployment, and monitoring pipeline for ML applications, unifying the contributions from the different teams involved and ensuring that all steps in the process are recorded and repeatable. In the next sections, we will learn how MLOps enables and empowers data science and IT teams to collaborate to build and maintain robust and scalable ML systems.