



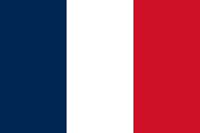


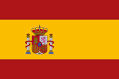



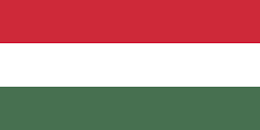

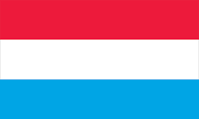





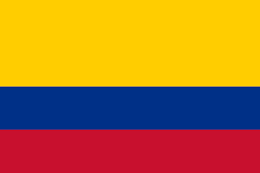
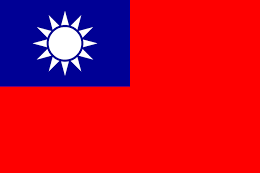
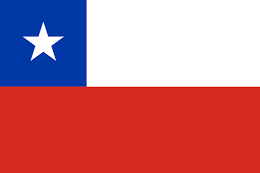



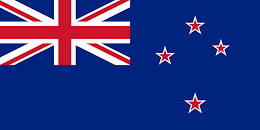
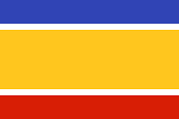
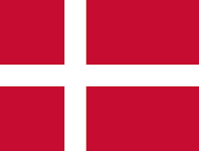
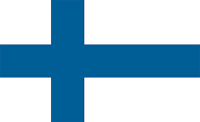







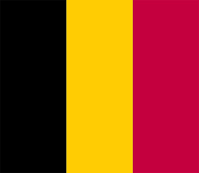

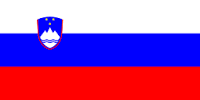





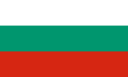


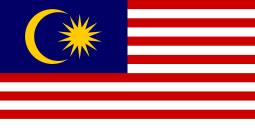



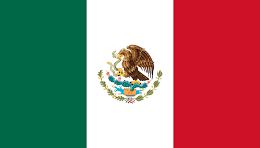

The world of data science is now starting to change quickly. This was arguably the year when discussions around AI and automation started to escalate, taking on more and more importance in the public sphere. But as interesting as all that is, there are nevertheless real people - like you - actually working with data not to rig elections or steal someone’s jobs but simply to make things better.
Arguably, data science and analysis has never been under the spotlight to the extent it is today. Whereas a decade ago there was a whole lotta hope stored in the big data revolution, today there’s anxiety that we’re not doing enough with data, that we don’t have the right data. That makes it a challenging but important time to be working in the world of data.
With that in mind, here are our 5 things that will matter in data science in 2018…
Find out what 5 data science tools we think will matter most in 2018 here.
This is huge, but it can’t be ignored. At its heart, this is important because it highlights that there’s human agency at the heart of modern data science, that algorithms are things created and designed by the engineers behind them. But even more important than that, these ethical considerations will be important in 2018 because it will end up defining everyone’s relationship to data for decades to come. And yes, although legislative bodies may play a part in that, it’s also up to people actually working with data to contribute to that discussion about what data does, who uses it and why. That might sound like a lot of responsibility, but it makes things pretty exciting, no?
This has long been a challenge for just about every business and, indeed, anyone who works in data - architect, analyst or scientist. But as the data hype curve flattens out with more organizations taking advantage of the opportunities it offers, with budgets getting tighter and expectations higher than they have ever been, ensuring that data programs are delivering real value will be crucial in 2018. That means there will be more pressure on data pros to deliver; sharpening your commercial instincts will be essential, and could be your route to the next step in your career.
If budgets are getting tighter and management expectations are higher than ever, the emergence of automated machine learning will be a godsend for 2018. Automated machine learning isn’t a threat to anyone’s job - it’s simply a way of making the steps of algorithm selection and optimization much faster. If you’ve ever lamented the time you’ve spent tweaking an algorithm only for it not to work as you wanted it to, only to move to a further iteration to find a similar problem, automated machine learning will automate away all those iterations. What this means is that you’ll be able to spend more time on value-adding activities that will never be automated away. And in turn this will make you a more valuable data scientist.
Cloud has been a big trend for some years now. But as a word on it’s own it’s always felt a bit abstract and amorphous. However, it’s once you start to see how it can be put into practice that you begin to see how potentially transformative it might be. In the case of machine learning, cloud becomes a vital solution in the battle for resources - it makes machine learning at scale more accessible to more people. The key tool here is Google’s cloud machine learning engine - it’s been built to make building machine learning models as straightforward as possible. When you look at this alongside automated machine learning, it’s possible to suggest that the data science skill set might change somewhat throughout 2018…
2018 is the year when all employees will need to be empowered by data. The idea that a specific team handles everything relating to data will end; using data will be crucial to a range of different stakeholders. This doesn’t mean the end of the data scientist - as said earlier, no one is going to be losing their jobs. But it does mean that self-service BI tools are going to take on greater importance than ever before in 2018. That means data scientists may have to start thinking more like data architects (especially if there’s no data architect in their organization), and taking into consideration how they make their work accessible and meaningful for stakeholders all around their organization.